Open Access
ARTICLE
Classifying Network Flows through a Multi-Modal 1D CNN Approach Using Unified Traffic Representations
Department of Computer Science and Engineering, Siddaganga Institute of Technology, Tumkur, 572103, India
* Corresponding Author: Ravi Veerabhadrappa. Email:
Computer Systems Science and Engineering 2025, 49, 333-351. https://doi.org/10.32604/csse.2025.061285
Received 21 November 2024; Accepted 06 February 2025; Issue published 19 March 2025
Abstract
In recent years, the analysis of encrypted network traffic has gained momentum due to the widespread use of Transport Layer Security and Quick UDP Internet Connections protocols, which complicate and prolong the analysis process. Classification models face challenges in understanding and classifying unknown traffic because of issues related to interpret ability and the representation of traffic data. To tackle these complexities, multi-modal representation learning can be employed to extract meaningful features and represent them in a lower-dimensional latent space. Recently, auto-encoder-based multi-modal representation techniques have shown superior performance in representing network traffic. By combining the advantages of multi-modal representation with efficient classifiers, we can develop robust network traffic classifiers. In this paper, we propose a novel multi-modal encoder-decoder model to create unified representations of network traffic, paired with a robust 1D-CNN (one-dimensional convolution neural network) classifier for effective traffic classification. The proposed model utilizes the ISCX Virtual Private Network-non Virtual Private Network 2016 datasets to extract general multi-modal representations and to train both shallow and deep learning models, such as Random Forest and the 1D-CNN model, for traffic classification. We compare these learning approaches based on the multi-modal representations generated from the autoencoder and the early feature fusion technique. For the classification task, both the Random Forest and 1D-CNN models, when trained on multimodal representations, achieve over 90% accuracy on a highly imbalanced dataset.Keywords
Cite This Article
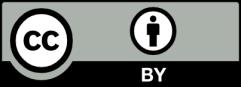
This work is licensed under a Creative Commons Attribution 4.0 International License , which permits unrestricted use, distribution, and reproduction in any medium, provided the original work is properly cited.