Open Access
ARTICLE
Point-Based Fusion for Multimodal 3D Detection in Autonomous Driving
School of Information and Control Engineering, China University of Mining and Technology, Xuzhou, 221116, China
* Corresponding Author: Bin Ye. Email:
(This article belongs to the Special Issue: Advanced Machine Learning and Artificial Intelligence in Engineering Applications)
Computer Systems Science and Engineering 2025, 49, 287-300. https://doi.org/10.32604/csse.2025.061655
Received 29 November 2024; Accepted 09 January 2025; Issue published 20 February 2025
Abstract
In the broader field of mechanical technology, and particularly in the context of self-driving vehicles, cameras and Light Detection and Ranging (LiDAR) sensors provide complementary modalities that hold significant potential for sensor fusion. However, directly merging multi-sensor data through point projection often results in information loss due to quantization, and managing the differing data formats from multiple sensors remains a persistent challenge. To address these issues, we propose a new fusion method that leverages continuous convolution, point-pooling, and a learned Multilayer Perceptron (MLP) to achieve superior detection performance. Our approach integrates the segmentation mask with raw LiDAR points rather than relying on projected points, effectively avoiding quantization loss. Additionally, when retrieving corresponding semantic information from images through point cloud projection, we employ linear interpolation and upsample the image feature maps to mitigate quantization loss. We employ nearest-neighbor search and continuous convolution to seamlessly fuse data from different formats. Moreover, we integrate pooling and aggregation operations, which serve as conceptual extensions of convolution, and are specifically designed to reconcile the inherent disparities among these data representations. Our detection network operates in two stages: in the first stage, preliminary proposals and segmentation features are generated; in the second stage, we refine the fusion results together with the segmentation mask to yield the final prediction. Notably, in our approach, the image network is used solely to provide semantic information, serving to enhance the point cloud features. Extensive experiments on the Karlsruhe Institute of Technology and Toyota Technological Institute (KITTI) dataset demonstrate the effectiveness of our approach, which achieves both high precision and robust performance in 3D object detection tasks.Keywords
Cite This Article
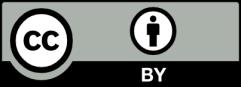
This work is licensed under a Creative Commons Attribution 4.0 International License , which permits unrestricted use, distribution, and reproduction in any medium, provided the original work is properly cited.