Open Access
ARTICLE
XGBoost Based Multiclass NLOS Channels Identification in UWB Indoor Positioning System
1 Faculty of Electrical Engineering, University of Technology Malaysia, Johor Bahru, 81310, Malaysia
2 Iraqi Ministry of Interior, Directorate of Training and Scientific Qualification, Baghdad, 10001, Iraq
3 Air Navigation Academy, General Company of Air Navigation Service, Baghdad, 10001, Iraq
* Corresponding Author: Ammar Fahem Majeed. Email:
Computer Systems Science and Engineering 2025, 49, 159-183. https://doi.org/10.32604/csse.2024.058741
Received 19 September 2024; Accepted 03 December 2024; Issue published 03 January 2025
Abstract
Accurate non-line of sight (NLOS) identification technique in ultra-wideband (UWB) location-based services is critical for applications like drone communication and autonomous navigation. However, current methods using binary classification (LOS/NLOS) oversimplify real-world complexities, with limited generalisation and adaptability to varying indoor environments, thereby reducing the accuracy of positioning. This study proposes an extreme gradient boosting (XGBoost) model to identify multi-class NLOS conditions. We optimise the model using grid search and genetic algorithms. Initially, the grid search approach is used to identify the most favourable values for integer hyperparameters. In order to achieve an optimised model configuration, the genetic algorithm is employed to fine-tune the floating-point hyperparameters. The model evaluations utilise a wide-ranging dataset of real-world measurements obtained with a Qorvo DW1000 UWB device, covering various indoor scenarios. Experimental results show that our proposed XGBoost achieved the highest overall accuracy of 99.47%, precision of 99%, recall of 99%, and an F-score of 99% on an open-source dataset. Additionally, based on a local dataset, the model achieved the highest performance, with an accuracy of 96%, precision of 96%, recall of 97%, and an F-score of 97%. In contrast to current machine learning methods in the literature, the suggestion model enhances classification accuracy and effectively addresses the NLOS/LOS identification as a multiclass propagation channel. This approach provides a robust solution with generalisation and adaptability across various dataset types and environments for more reliable and accurate indoor positioning technologies.Keywords
Cite This Article
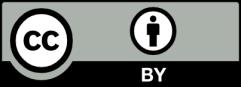
This work is licensed under a Creative Commons Attribution 4.0 International License , which permits unrestricted use, distribution, and reproduction in any medium, provided the original work is properly cited.