Open Access
ARTICLE
Prairie Araneida Optimization Based Fused CNN Model for Intrusion Detection
School of Computer Science, Dr. Vishwanath Karad MIT-WPU, Pune, 411038, Maharashtra, India
* Corresponding Author: Shubhalaxmi Joshi. Email:
(This article belongs to the Special Issue: Artificial Intelligence for Cyber Security)
Computer Systems Science and Engineering 2025, 49, 49-77. https://doi.org/10.32604/csse.2024.057702
Received 25 October 2024; Accepted 05 November 2024; Issue published 03 January 2025
Abstract
Intrusion detection (ID) is a cyber security practice that encompasses the process of monitoring network activities to identify unauthorized or malicious actions. This includes problems like the difficulties of existing intrusion detection models to identify emerging attacks, generating many false alarms, and their inability and difficulty to adapt themselves with time when it comes to threats, hence to overcome all those existing challenges in this research develop a Prairie Araneida optimization based fused Convolutional Neural Network model (PAO-CNN) for intrusion detection. The fused CNN (Convolutional Neural Netowrk) is a remarkable development since it combines statistical features that are extracted from the processed data and provide enhanced capabilities for the model to capture complicated patterns existing in intrusion datasets. The adoption of a fused architecture represents an integrated way towards intrusion detection where the model can significantly interpret various features to achieve higher accuracy. On top of this, the Prairie Araneida stage which is based on coyote behavior and social spider colonies respectively plays a role in enabling to handling of intricate optimization landscapes. The dual contribution of a fused CNN and novel optimization strategies strengthens the research’s goal to design an effective intrusion detection system that can evolve with new cyber threats. When the Training percentage (TP) is set to 90, the model’s performance can be assessed using metrics like Accuracy, Sensitivity, and Specificity. In this particular dataset, these metrics reach approximately 97.78%, 96.25%, and 96.15%, respectively, which are crucial values. Additionally, when using a k-fold value of 6, the model achieves metrics of 97.04% Accuracy, 97.37% Sensitivity, and 96.48% Specificity.Keywords
Cite This Article
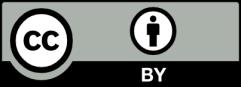
This work is licensed under a Creative Commons Attribution 4.0 International License , which permits unrestricted use, distribution, and reproduction in any medium, provided the original work is properly cited.