Open Access
ARTICLE
Machine Learning-Driven Classification for Enhanced Rule Proposal Framework
1 Department of Computer Science and Engineering, PSG Institute of Technology and Applied Research, Coimbatore, 641062, India
2 SAP Labs India Private Limited, Bengaluru, 560066, India
3 SAP (UK) Ltd., Middlesex, TW148HD, UK
* Corresponding Author: B. Gomathi. Email:
Computer Systems Science and Engineering 2024, 48(6), 1749-1765. https://doi.org/10.32604/csse.2024.056659
Received 27 July 2024; Accepted 18 October 2024; Issue published 22 November 2024
Abstract
In enterprise operations, maintaining manual rules for enterprise processes can be expensive, time-consuming, and dependent on specialized domain knowledge in that enterprise domain. Recently, rule-generation has been automated in enterprises, particularly through Machine Learning, to streamline routine tasks. Typically, these machine models are black boxes where the reasons for the decisions are not always transparent, and the end users need to verify the model proposals as a part of the user acceptance testing to trust it. In such scenarios, rules excel over Machine Learning models as the end-users can verify the rules and have more trust. In many scenarios, the truth label changes frequently thus, it becomes difficult for the Machine Learning model to learn till a considerable amount of data has been accumulated, but with rules, the truth can be adapted. This paper presents a novel framework for generating human-understandable rules using the Classification and Regression Tree (CART) decision tree method, which ensures both optimization and user trust in automated decision-making processes. The framework generates comprehensible rules in the form of if condition and then predicts class even in domains where noise is present. The proposed system transforms enterprise operations by automating the production of human-readable rules from structured data, resulting in increased efficiency and transparency. Removing the need for human rule construction saves time and money while guaranteeing that users can readily check and trust the automatic judgments of the system. The remarkable performance metrics of the framework, which achieve 99.85% accuracy and 96.30% precision, further support its efficiency in translating complex data into comprehensible rules, eventually empowering users and enhancing organizational decision-making processes.Keywords
Cite This Article
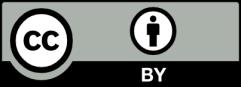
This work is licensed under a Creative Commons Attribution 4.0 International License , which permits unrestricted use, distribution, and reproduction in any medium, provided the original work is properly cited.