Open Access
ARTICLE
An Expert System to Detect Political Arabic Articles Orientation Using CatBoost Classifier Boosted by Multi-Level Features
1 Department of Information Technology, Institute of Graduate Studies and Research, Alexandria University, El Shatby, P.O. Box 832, Alexandria, 21526, Egypt
2 Department of Computer Science, College of Computer Science and Information Technology, University of Anbar, Baghdad, 55431, Iraq
* Corresponding Author: Saad M. Darwish. Email:
Computer Systems Science and Engineering 2024, 48(6), 1595-1624. https://doi.org/10.32604/csse.2024.054615
Received 03 June 2024; Accepted 08 August 2024; Issue published 22 November 2024
Abstract
The number of blogs and other forms of opinionated online content has increased dramatically in recent years. Many fields, including academia and national security, place an emphasis on automated political article orientation detection. Political articles (especially in the Arab world) are different from other articles due to their subjectivity, in which the author’s beliefs and political affiliation might have a significant influence on a political article. With categories representing the main political ideologies, this problem may be thought of as a subset of the text categorization (classification). In general, the performance of machine learning models for text classification is sensitive to hyperparameter settings. Furthermore, the feature vector used to represent a document must capture, to some extent, the complex semantics of natural language. To this end, this paper presents an intelligent system to detect political Arabic article orientation that adapts the categorical boosting (CatBoost) method combined with a multi-level feature concept. Extracting features at multiple levels can enhance the model’s ability to discriminate between different classes or patterns. Each level may capture different aspects of the input data, contributing to a more comprehensive representation. CatBoost, a robust and efficient gradient-boosting algorithm, is utilized to effectively learn and predict the complex relationships between these features and the political orientation labels associated with the articles. A dataset of political Arabic texts collected from diverse sources, including postings and articles, is used to assess the suggested technique. Conservative, reform, and revolutionary are the three subcategories of these opinions. The results of this study demonstrate that compared to other frequently used machine learning models for text classification, the CatBoost method using multi-level features performs better with an accuracy of 98.14%.Keywords
Cite This Article
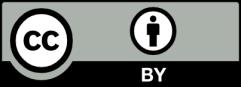
This work is licensed under a Creative Commons Attribution 4.0 International License , which permits unrestricted use, distribution, and reproduction in any medium, provided the original work is properly cited.