Open Access
ARTICLE
Modern Mobile Malware Detection Framework Using Machine Learning and Random Forest Algorithm
School of Computing Sciences, Princess Sumaya University for Technology, Amman, 11941, Jordan
* Corresponding Author: Mohammad Ababneh. Email:
Computer Systems Science and Engineering 2024, 48(5), 1171-1191. https://doi.org/10.32604/csse.2024.052875
Received 18 April 2024; Accepted 05 July 2024; Issue published 13 September 2024
Abstract
With the high level of proliferation of connected mobile devices, the risk of intrusion becomes higher. Artificial Intelligence (AI) and Machine Learning (ML) algorithms started to feature in protection software and showed effective results. These algorithms are nonetheless hindered by the lack of rich datasets and compounded by the appearance of new categories of malware such that the race between attackers’ malware, especially with the assistance of Artificial Intelligence tools and protection solutions makes these systems and frameworks lose effectiveness quickly. In this article, we present a framework for mobile malware detection based on a new dataset containing new categories of mobile malware. We focus on categories of malware that were not tested before by Machine Learning algorithms proven effective in malware detection. We carefully select an optimal number of features, do necessary preprocessing, and then apply Machine Learning algorithms to discover malicious code effectively. From our experiments, we have found that the Random Forest algorithm is the best-performing algorithm with such mobile malware with detection rates of around 99%. We compared our results from this work and found that they are aligned well with our previous work. We also compared our work with State-of-the-Art works of others and found that the results are very close and competitive.Keywords
Cite This Article
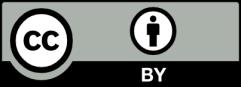
This work is licensed under a Creative Commons Attribution 4.0 International License , which permits unrestricted use, distribution, and reproduction in any medium, provided the original work is properly cited.