Open Access
ARTICLE
Emotion Detection Using ECG Signals and a Lightweight CNN Model
Department of Electronics and Telecommunication Engineering, Goa College of Engineering, Goa University, Ponda, 403401, India
* Corresponding Author: Amita U. Dessai. Email:
(This article belongs to the Special Issue: Deep Learning and Ontology in Smart Healthcare)
Computer Systems Science and Engineering 2024, 48(5), 1193-1211. https://doi.org/10.32604/csse.2024.052710
Received 12 April 2024; Accepted 15 July 2024; Issue published 13 September 2024
Abstract
Emotion recognition is a growing field that has numerous applications in smart healthcare systems and Human-Computer Interaction (HCI). However, physical methods of emotion recognition such as facial expressions, voice, and text data, do not always indicate true emotions, as users can falsify them. Among the physiological methods of emotion detection, Electrocardiogram (ECG) is a reliable and efficient way of detecting emotions. ECG-enabled smart bands have proven effective in collecting emotional data in uncontrolled environments. Researchers use deep machine learning techniques for emotion recognition using ECG signals, but there is a need to develop efficient models by tuning the hyperparameters. Furthermore, most researchers focus on detecting emotions in individual settings, but there is a need to extend this research to group settings as well since most of the emotions are experienced in groups. In this study, we have developed a novel lightweight one dimensional (1D) Convolutional Neural Network (CNN) model by reducing the number of convolution, max pooling, and classification layers. This optimization has led to more efficient emotion classification using ECG. We tested the proposed model’s performance using ECG data from the AMIGOS (A Dataset for Affect, Personality and Mood Research on Individuals and Groups) dataset for both individual and group settings. The results showed that the model achieved an accuracy of 82.21% and 85.62% for valence and arousal classification, respectively, in individual settings. In group settings, the accuracy was even higher, at 99.56% and 99.68% for valence and arousal classification, respectively. By reducing the number of layers, the lightweight CNN model can process data more quickly and with less complexity in the hardware, making it suitable for the implementation on the mobile phone devices to detect emotions with improved accuracy and speed.Keywords
Cite This Article
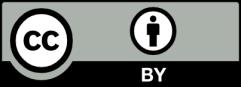
This work is licensed under a Creative Commons Attribution 4.0 International License , which permits unrestricted use, distribution, and reproduction in any medium, provided the original work is properly cited.