Open Access
ARTICLE
A Stacking Machine Learning Model for Student Performance Prediction Based on Class Activities in E-Learning
Department of Computer Engineering, University of Science and Culture, Tehran, 1461968151, Iran
* Corresponding Author: Mohammad Javad Shayegan. Email:
Computer Systems Science and Engineering 2024, 48(5), 1251-1272. https://doi.org/10.32604/csse.2024.052587
Received 08 April 2024; Accepted 22 July 2024; Issue published 13 September 2024
Abstract
After the spread of COVID-19, e-learning systems have become crucial tools in educational systems worldwide, spanning all levels of education. This widespread use of e-learning platforms has resulted in the accumulation of vast amounts of valuable data, making it an attractive resource for predicting student performance. In this study, we aimed to predict student performance based on the analysis of data collected from the OULAD and Deeds datasets. The stacking method was employed for modeling in this research. The proposed model utilized weak learners, including nearest neighbor, decision tree, random forest, enhanced gradient, simple Bayes, and logistic regression algorithms. After a trial-and-error process, the logistic regression algorithm was selected as the final learner for the proposed model. The results of experiments with the above algorithms are reported separately for the pass and fail classes. The findings indicate that the accuracy of the proposed model on the OULAD dataset reached 98%. Overall, the proposed method improved accuracy by 4% on the OULAD dataset.Keywords
Cite This Article
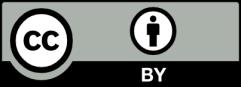
This work is licensed under a Creative Commons Attribution 4.0 International License , which permits unrestricted use, distribution, and reproduction in any medium, provided the original work is properly cited.