Open Access
ARTICLE
Performance of Deep Learning Techniques in Leaf Disease Detection
1 Centre of Real Time Computer Systems, Kaunas University of Technology, Kaunas, 51386, Lithuania
2 Department of Computer Science, Air University, Islamabad, 44000, Pakistan
3 Department of Computer Science, City University, Peshawar, 25000, Pakistan
* Corresponding Author: Robertas Damasevicius. Email:
Computer Systems Science and Engineering 2024, 48(5), 1349-1366. https://doi.org/10.32604/csse.2024.050359
Received 04 February 2024; Accepted 24 July 2024; Issue published 13 September 2024
Abstract
Plant diseases must be identified as soon as possible since they have an impact on the growth of the corresponding species. Consequently, the identification of leaf diseases is essential in this field of agriculture. Diseases brought on by bacteria, viruses, and fungi are a significant factor in reduced crop yields. Numerous machine learning models have been applied in the identification of plant diseases, however, with the recent developments in deep learning, this field of study seems to hold huge potential for improved accuracy. This study presents an effective method that uses image processing and deep learning approaches to distinguish between healthy and infected leaves. To effectively identify leaf diseases, we employed pre-trained models based on Convolutional Neural Networks (CNNs). There are four deep neural networks approaches used in this study: Convolutional Neural Network (CNN), Inception-V3, DenseNet-121, and VGG-16. Our focus was on optimizing the hyper-parameters of these deep learning models with prior training. For the evaluation of these deep neural networks, standard evaluation measures are used, such as F1-score, recall, precision, accuracy, and Area Under Curve (AUC). The overall outcomes show the better performance of Inception-V3 with an achieved accuracy of 95.5%, as well as the performance of DenseNet-121 with an accuracy of 94.4%. VGG-16 performed well as well, with an accuracy of 93.3%, and CNN achieved an accuracy of 91.9%.Keywords
Cite This Article
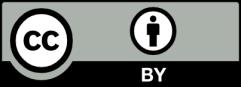
This work is licensed under a Creative Commons Attribution 4.0 International License , which permits unrestricted use, distribution, and reproduction in any medium, provided the original work is properly cited.