Open Access
ARTICLE
A Multivariate Relevance Frequency Analysis Based Feature Selection for Classification of Short Text Data
Department of Computing, Coimbatore Institute of Technology, Coimbatore, 641014, India
* Corresponding Author: Saravanan Arumugam. Email:
Computer Systems Science and Engineering 2024, 48(4), 989-1008. https://doi.org/10.32604/csse.2024.051770
Received 14 March 2024; Accepted 07 May 2024; Issue published 17 July 2024
Abstract
Text mining presents unique challenges in extracting meaningful information from the vast volumes of digital documents. Traditional filter feature selection methods often fall short in handling the complexities of short text data. To address this issue, this paper presents a novel approach to feature selection in text classification, aiming to overcome challenges posed by high dimensionality and reduced accuracy in the face of increasing digital document volumes. Unlike traditional filter feature selection techniques, the proposed method, Multivariate Relevance Frequency Analysis, offers a tailored solution for diverse text data types. By integrating positive, negative, and dependency relevance computations, the proposed approach effectively prunes features, enhancing classification performance. Extensive experimental analysis has been performed for the proposed model and compared with several standard existing feature selection models on five datasets involving short and long texts using four standard classifiers. The results indicate that the proposed model has the highest macro-F1 score of 94% for the SMS dataset, 78.1% for the SLS dataset, 89.4% for the AYSC dataset, 71.32% for the Reuters dataset, and 98.63% for the 20Newsgroup dataset. The statistical analysis also indicates that the proposed model provides better performance with both short texts such as messages and reviews as well as long texts containing documents, with superior performance for short-text data. The comparative analysis shows that the proposed model offers better performance than many other standard filtration models.Keywords
Cite This Article
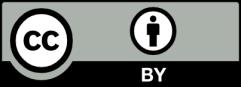
This work is licensed under a Creative Commons Attribution 4.0 International License , which permits unrestricted use, distribution, and reproduction in any medium, provided the original work is properly cited.