Open Access
ARTICLE
Reducing the Encrypted Data Size: Healthcare with IoT-Cloud Computing Applications
1 LIAP Laboratory, University of El Oued, P.O. Box 789, El Oued, 39000, Algeria
2 Computer Science Department, King Fahd University of Petroleum and Minerals, Dhahran, 31261, Kingdom of Saudi Arabia
3 National Higher School of Mathematics, Scientific and Technology Hub of Sidi Abdellah, P.O. Box 75, Algiers, 16093, Algeria
4 Department of Electrical and Computer Engineering, Hellenic Mediterranean University, Heraklion, 71004, Greece
* Corresponding Author: Abdelkader Laouid. Email:
(This article belongs to the Special Issue: Explainable AI and Cybersecurity Techniques for IoT-Based Medical and Healthcare Applications)
Computer Systems Science and Engineering 2024, 48(4), 1055-1072. https://doi.org/10.32604/csse.2024.048738
Received 17 December 2023; Accepted 29 March 2024; Issue published 17 July 2024
Abstract
Internet cloud services come at a price, especially when they provide top-tier security measures. The cost incurred by cloud utilization is directly proportional to the storage requirements. Companies are always looking to increase profits and reduce costs while preserving the security of their data by encrypting them. One of the offered solutions is to find an efficient encryption method that can store data in a much smaller space than traditional encryption techniques. This article introduces a novel encryption approach centered on consolidating information into a single ciphertext by implementing Multi-Key Embedded Encryption (MKEE). The effectiveness of MKEE scales in tandem with the volume of information encapsulated within the ciphertext. MKEE substantially reduced the size of the ciphertext, achieving an 88% decrease when incorporating ten plaintext values. To further reduce the size of the ciphertext in our proposal, a Modular Multiplicative Inverse method (MMI) is introduced. MMI experiments were conducted, demonstrating that we achieved a commendable 50% reduction in the ciphertext size. To validate the practicality of the proposed method, a case study was conducted on a diabetes dataset. By integrating MKEE and MMI, this study showed a data storage reduction of 94%.Keywords
Cite This Article
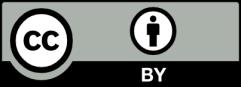
This work is licensed under a Creative Commons Attribution 4.0 International License , which permits unrestricted use, distribution, and reproduction in any medium, provided the original work is properly cited.