Open Access
ARTICLE
MG-YOLOv5s: A Faster and Stronger Helmet Detection Algorithm
College of Computer Science, Hubei University of Technology, Wuhan, 430000, China
* Corresponding Author: Zhiwei Ye. Email:
Computer Systems Science and Engineering 2024, 48(4), 1009-1029. https://doi.org/10.32604/csse.2023.040475
Received 20 March 2023; Accepted 23 May 2023; Issue published 17 July 2024
Abstract
Nowadays, construction site safety accidents are frequent, and wearing safety helmets is essential to prevent head injuries caused by object collisions and falls. However, existing helmet detection algorithms have several drawbacks, including a complex structure with many parameters, high calculation volume, and poor detection of small helmets, making deployment on embedded or mobile devices difficult. To address these challenges, this paper proposes a YOLOv5-based multi-head detection safety helmet detection algorithm that is faster and more robust for detecting helmets on construction sites. By replacing the traditional DarkNet backbone network of YOLOv5s with a new backbone network composed of RepStemBlock and MG, introducing the C3STR multi-head self-attention mechanism and adding a small detection head P6 to replace the original feature pyramid network, the improved model reduces parameters by 44.1%, responds faster to inference, significantly outperforms the original model in accuracy and mAP (2.3% and 2.7% improvement). Two models are presented in this paper: a large model (YOLOv5s-hat) for optimal performance, mAP@0.5 is 0.957, and a small model (MG-YOLOv5s) for real-time detection on embedded or mobile devices. Compared to large models the lightweight model has 44% fewer parameters, mAP@0.5 of 0.954 and an Fps of 67. Overall, the proposed algorithm shows promise for improving helmet detection on construction sites, with the potential for deployment on a range of devices.Keywords
Cite This Article
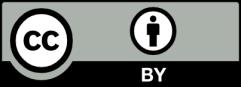
This work is licensed under a Creative Commons Attribution 4.0 International License , which permits unrestricted use, distribution, and reproduction in any medium, provided the original work is properly cited.