Open Access
ARTICLE
Analyzing COVID-19 Discourse on Twitter: Text Clustering and Classification Models for Public Health Surveillance
1 Department of Information Technology, Lampang Rajabhat University, Lampang, 52100, Thailand
2 Department of Computer Science, Lampang Rajabhat University, Lampang, 52100, Thailand
* Corresponding Author: Samai Srisuay. Email:
Computer Systems Science and Engineering 2024, 48(3), 665-689. https://doi.org/10.32604/csse.2024.045066
Received 16 August 2023; Accepted 26 January 2024; Issue published 20 May 2024
Abstract
Social media has revolutionized the dissemination of real-life information, serving as a robust platform for sharing life events. Twitter, characterized by its brevity and continuous flow of posts, has emerged as a crucial source for public health surveillance, offering valuable insights into public reactions during the COVID-19 pandemic. This study aims to leverage a range of machine learning techniques to extract pivotal themes and facilitate text classification on a dataset of COVID-19 outbreak-related tweets. Diverse topic modeling approaches have been employed to extract pertinent themes and subsequently form a dataset for training text classification models. An assessment of coherence metrics revealed that the Gibbs Sampling Dirichlet Mixture Model (GSDMM), which utilizes trigram and bag-of-words (BOW) feature extraction, outperformed Non-negative Matrix Factorization (NMF), Latent Dirichlet Allocation (LDA), and a hybrid strategy involving Bidirectional Encoder Representations from Transformers (BERT) combined with LDA and K-means to pinpoint significant themes within the dataset. Among the models assessed for text clustering, the utilization of LDA, either as a clustering model or for feature extraction combined with BERT for K-means, resulted in higher coherence scores, consistent with human ratings, signifying their efficacy. In particular, LDA, notably in conjunction with trigram representation and BOW, demonstrated superior performance. This underscores the suitability of LDA for conducting topic modeling, given its proficiency in capturing intricate textual relationships. In the context of text classification, models such as Linear Support Vector Classification (LSVC), Long Short-Term Memory (LSTM), Bidirectional Long Short-Term Memory (BiLSTM), Convolutional Neural Network with BiLSTM (CNN-BiLSTM), and BERT have shown outstanding performance, achieving accuracy and weighted F1-Score scores exceeding 80%. These results significantly surpassed other models, such as Multinomial Naive Bayes (MNB), Linear Support Vector Machine (LSVM), and Logistic Regression (LR), which achieved scores in the range of 60 to 70 percent.Keywords
Cite This Article
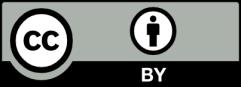