Open Access
ARTICLE
Multimodal Deep Neural Networks for Digitized Document Classification
1 Department of Computer Engineering, Khoja Akhmet Yassawi International Kazakh-Turkish University, Turkistan, Kazakhstan
2 Department of Computer and Software Engineering, L.N. Gumilyov Eurasian National University, Astana, Kazakhstan
3 Department of Information and Computing Systems, Non-Profit Joint Stock Company Abylkas Saginov Karaganda Technical University, Karaganda, Kazakhstan
4 Department of Information Systems, Al-Farabi Kazakh National University, Almaty, Kazakhstan
* Corresponding Author: Aigerim Baimakhanova. Email:
Computer Systems Science and Engineering 2024, 48(3), 793-811. https://doi.org/10.32604/csse.2024.043273
Received 27 June 2023; Accepted 14 November 2023; Issue published 20 May 2024
Abstract
As digital technologies have advanced more rapidly, the number of paper documents recently converted into a digital format has exponentially increased. To respond to the urgent need to categorize the growing number of digitized documents, the classification of digitized documents in real time has been identified as the primary goal of our study. A paper classification is the first stage in automating document control and efficient knowledge discovery with no or little human involvement. Artificial intelligence methods such as Deep Learning are now combined with segmentation to study and interpret those traits, which were not conceivable ten years ago. Deep learning aids in comprehending input patterns so that object classes may be predicted. The segmentation process divides the input image into separate segments for a more thorough image study. This study proposes a deep learning-enabled framework for automated document classification, which can be implemented in higher education. To further this goal, a dataset was developed that includes seven categories: Diplomas, Personal documents, Journal of Accounting of higher education diplomas, Service letters, Orders, Production orders, and Student orders. Subsequently, a deep learning model based on Conv2D layers is proposed for the document classification process. In the final part of this research, the proposed model is evaluated and compared with other machine-learning techniques. The results demonstrate that the proposed deep learning model shows high results in document categorization overtaking the other machine learning models by reaching 94.84%, 94.79%, 94.62%, 94.43%, 94.07% in accuracy, precision, recall, F-score, and AUC-ROC, respectively. The achieved results prove that the proposed deep model is acceptable to use in practice as an assistant to an office worker.Keywords
Cite This Article
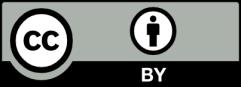