Open Access
ARTICLE
A Hybrid Machine Learning Framework for Security Intrusion Detection
Mathematics and Computer Science Department, Faculty of Science, Alexandria University, Alexandria, Egypt
* Corresponding Author: Fatimah Mudhhi Alanazi. Email:
Computer Systems Science and Engineering 2024, 48(3), 835-851. https://doi.org/10.32604/csse.2024.042401
Received 29 May 2023; Accepted 08 January 2024; Issue published 20 May 2024
Abstract
Proliferation of technology, coupled with networking growth, has catapulted cybersecurity to the forefront of modern security concerns. In this landscape, the precise detection of cyberattacks and anomalies within networks is crucial, necessitating the development of efficient intrusion detection systems (IDS). This article introduces a framework utilizing the fusion of fuzzy sets with support vector machines (SVM), named FSVM. The core strategy of FSVM lies in calculating the significance of network features to determine their relative importance. Features with minimal significance are prudently disregarded, a method akin to feature selection. This process not only curtails the computational burden of the classification algorithm but also ensures the preservation of high accuracy levels. To ascertain the efficacy of the FSVM model, we have employed a publicly available dataset from Kaggle, which encompasses two distinct decision labels. Our evaluation methodology involves a comprehensive comparison of the classification accuracy of the processed dataset against four contemporary models in the field. Key performance metrics scores are meticulously calculated for each model. The comparative analysis reveals that the FSVM model demonstrates a marked superiority over its counterparts, enhancing classification accuracy by a minimum of 3%. These findings underscore the FSVM model’s robustness and reliability, positioning it as a highly effective tool in the realm of cybersecurity.Keywords
Cite This Article
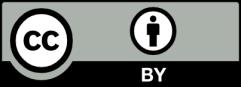
This work is licensed under a Creative Commons Attribution 4.0 International License , which permits unrestricted use, distribution, and reproduction in any medium, provided the original work is properly cited.