Open Access
ARTICLE
A New Malicious Code Classification Method for the Security of Financial Software
1 Postdoctoral Workstation, Bank of Suzhou, Suzhou, 215000, China
2 Postdoctoral Research Station, Nanjing University, Nanjing, 210093, China
3 Business School, Nanjing University, Nanjing, 210093, China
4 College of Air and Missile Defense, Air Force Engineering University, Xi’an, 710051, China
* Corresponding Author: Mingliang Zhang. Email:
(This article belongs to the Special Issue: Artificial Intelligence for Cyber Security)
Computer Systems Science and Engineering 2024, 48(3), 773-792. https://doi.org/10.32604/csse.2024.039849
Received 20 February 2023; Accepted 27 December 2023; Issue published 20 May 2024
Abstract
The field of finance heavily relies on cybersecurity to safeguard its systems and clients from harmful software. The identification of malevolent code within financial software is vital for protecting both the financial system and individual clients. Nevertheless, present detection models encounter limitations in their ability to identify malevolent code and its variations, all while encompassing a multitude of parameters. To overcome these obstacles, we introduce a lean model for classifying families of malevolent code, formulated on Ghost-DenseNet-SE. This model integrates the Ghost module, DenseNet, and the squeeze-and-excitation (SE) channel domain attention mechanism. It substitutes the standard convolutional layer in DenseNet with the Ghost module, thereby diminishing the model’s size and augmenting recognition speed. Additionally, the channel domain attention mechanism assigns distinctive weights to feature channels, facilitating the extraction of pivotal characteristics of malevolent code and bolstering detection precision. Experimental outcomes on the Malimg dataset indicate that the model attained an accuracy of 99.14% in discerning families of malevolent code, surpassing AlexNet (97.8%) and The visual geometry group network (VGGNet) (96.16%). The proposed model exhibits reduced parameters, leading to decreased model complexity alongside enhanced classification accuracy, rendering it a valuable asset for categorizing malevolent code.Keywords
Cite This Article
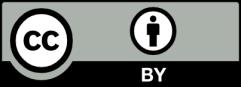
This work is licensed under a Creative Commons Attribution 4.0 International License , which permits unrestricted use, distribution, and reproduction in any medium, provided the original work is properly cited.