Open Access
ARTICLE
Security Monitoring and Management for the Network Services in the Orchestration of SDN-NFV Environment Using Machine Learning Techniques
1 Department of Computer Science, College of Computer and Information Sciences, Jouf University, Sakaka, Al Jouf, 72341, Saudi Arabia
2 Department of Computer Sciences, Kinnaird College for Women, Lahore, Punjab, 54700, Pakistan
3 Department of Information Sciences, Division of Sciences and Technology, University of Education, Lahore, 54770, Pakistan
4 Department of Computer Engineering and Networks, College of Computer and Information Sciences, Jouf University, Sakaka, 72341, Saudi Arabia
5 Computer Science, Florida Polytechnic University, Lakeland, Florida, 33805, USA
6 Delta3T, Lahore, Punjab, 54700, Pakistan
7 Department of Basic Sciences, Common First Year, Jouf University, Sakaka, 72341, Saudi Arabia
* Corresponding Author: Saad Awadh Alanazi. Email:
(This article belongs to the Special Issue: Advances in Mobile Internet Security)
Computer Systems Science and Engineering 2024, 48(2), 363-394. https://doi.org/10.32604/csse.2023.040721
Received 29 March 2023; Accepted 22 May 2023; Issue published 19 March 2024
Abstract
Software Defined Network (SDN) and Network Function Virtualization (NFV) technology promote several benefits to network operators, including reduced maintenance costs, increased network operational performance, simplified network lifecycle, and policies management. Network vulnerabilities try to modify services provided by Network Function Virtualization MANagement and Orchestration (NFV MANO), and malicious attacks in different scenarios disrupt the NFV Orchestrator (NFVO) and Virtualized Infrastructure Manager (VIM) lifecycle management related to network services or individual Virtualized Network Function (VNF). This paper proposes an anomaly detection mechanism that monitors threats in NFV MANO and manages promptly and adaptively to implement and handle security functions in order to enhance the quality of experience for end users. An anomaly detector investigates these identified risks and provides secure network services. It enables virtual network security functions and identifies anomalies in Kubernetes (a cloud-based platform). For training and testing purpose of the proposed approach, an intrusion-containing dataset is used that hold multiple malicious activities like a Smurf, Neptune, Teardrop, Pod, Land, IPsweep, etc., categorized as Probing (Prob), Denial of Service (DoS), User to Root (U2R), and Remote to User (R2L) attacks. An anomaly detector is anticipated with the capabilities of a Machine Learning (ML) technique, making use of supervised learning techniques like Logistic Regression (LR), Support Vector Machine (SVM), Random Forest (RF), Naïve Bayes (NB), and Extreme Gradient Boosting (XGBoost). The proposed framework has been evaluated by deploying the identified ML algorithm on a Jupyter notebook in Kubeflow to simulate Kubernetes for validation purposes. RF classifier has shown better outcomes (99.90% accuracy) than other classifiers in detecting anomalies/intrusions in the containerized environment.Keywords
Cite This Article
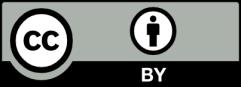
This work is licensed under a Creative Commons Attribution 4.0 International License , which permits unrestricted use, distribution, and reproduction in any medium, provided the original work is properly cited.