Open Access
ARTICLE
DeepSVDNet: A Deep Learning-Based Approach for Detecting and Classifying Vision-Threatening Diabetic Retinopathy in Retinal Fundus Images
1 College of Information Science and Technology, Hainan Normal University, Haikou, 571158, China
2 Department of Creative Technologies, Air University, Islamabad, Pakistan
3 School of Life Science and Technology, University of Electronic Science and Technology of China UESTC, Chengdu, China
4 School of Science and Technology, University of Management and Technology UMT, Lahore, Punjab, Pakistan
5 Faculty of Computer Science & Information Technology, Al Baha University, Al Baha, Saudi Arabia
6 School of Information Engineering, Qujing Normal University, Qujing, China
* Corresponding Author: Xiaowen Liu. Email:
(This article belongs to the Special Issue: Explainable AI and Cybersecurity Techniques for IoT-Based Medical and Healthcare Applications)
Computer Systems Science and Engineering 2024, 48(2), 511-528. https://doi.org/10.32604/csse.2023.039672
Received 10 February 2023; Accepted 16 June 2023; Issue published 19 March 2024
Abstract
Artificial Intelligence (AI) is being increasingly used for diagnosing Vision-Threatening Diabetic Retinopathy (VTDR), which is a leading cause of visual impairment and blindness worldwide. However, previous automated VTDR detection methods have mainly relied on manual feature extraction and classification, leading to errors. This paper proposes a novel VTDR detection and classification model that combines different models through majority voting. Our proposed methodology involves preprocessing, data augmentation, feature extraction, and classification stages. We use a hybrid convolutional neural network-singular value decomposition (CNN-SVD) model for feature extraction and selection and an improved SVM-RBF with a Decision Tree (DT) and K-Nearest Neighbor (KNN) for classification. We tested our model on the IDRiD dataset and achieved an accuracy of 98.06%, a sensitivity of 83.67%, and a specificity of 100% for DR detection and evaluation tests, respectively. Our proposed approach outperforms baseline techniques and provides a more robust and accurate method for VTDR detection.Keywords
Cite This Article
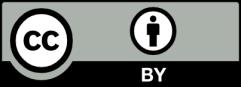