Open Access
ARTICLE
SwinVid: Enhancing Video Object Detection Using Swin Transformer
1 Computer Science Department, Faculty of Artificial Intelligence and Informatics, Benha, Egypt
2 Mathematics Department, Faculty of Sciences, Al-Azhar University, Cairo, Egypt
3 Department of Computer Science, College of Science and Arts in Qurayyat, Jouf University, Sakaka, Saudi Arabia
* Corresponding Author: Abdelrahman Maharek. Email:
(This article belongs to the Special Issue: Explainable AI and Cybersecurity Techniques for IoT-Based Medical and Healthcare Applications)
Computer Systems Science and Engineering 2024, 48(2), 305-320. https://doi.org/10.32604/csse.2024.039436
Received 30 January 2023; Accepted 11 May 2023; Issue published 19 March 2024
Abstract
What causes object detection in video to be less accurate than it is in still images? Because some video frames have degraded in appearance from fast movement, out-of-focus camera shots, and changes in posture. These reasons have made video object detection (VID) a growing area of research in recent years. Video object detection can be used for various healthcare applications, such as detecting and tracking tumors in medical imaging, monitoring the movement of patients in hospitals and long-term care facilities, and analyzing videos of surgeries to improve technique and training. Additionally, it can be used in telemedicine to help diagnose and monitor patients remotely. Existing VID techniques are based on recurrent neural networks or optical flow for feature aggregation to produce reliable features which can be used for detection. Some of those methods aggregate features on the full-sequence level or from nearby frames. To create feature maps, existing VID techniques frequently use Convolutional Neural Networks (CNNs) as the backbone network. On the other hand, Vision Transformers have outperformed CNNs in various vision tasks, including object detection in still images and image classification. We propose in this research to use Swin-Transformer, a state-of-the-art Vision Transformer, as an alternative to CNN-based backbone networks for object detection in videos. The proposed architecture enhances the accuracy of existing VID methods. The ImageNet VID and EPIC KITCHENS datasets are used to evaluate the suggested methodology. We have demonstrated that our proposed method is efficient by achieving 84.3% mean average precision (mAP) on ImageNet VID using less memory in comparison to other leading VID techniques. The source code is available on the website .Keywords
Cite This Article
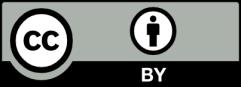
This work is licensed under a Creative Commons Attribution 4.0 International License , which permits unrestricted use, distribution, and reproduction in any medium, provided the original work is properly cited.