Open Access
ARTICLE
Efficient DP-FL: Efficient Differential Privacy Federated Learning Based on Early Stopping Mechanism
1 College of Automation and Information Engineering, Sichuan University of Science and Engineering, Yibin, 644000, China
2 Sanjiang Research Institute of Artificial Intelligence and Robotics, Yibin University, Yibin, 644000, China
3 Science and Technology on Communication Security Laboratory, China Electronics Technology Corporation 30th Research Institute, Chengdu, 610041, China
* Corresponding Author: Lecai Cai. Email:
(This article belongs to the Special Issue: Security and Privacy Challenges in Smart City)
Computer Systems Science and Engineering 2024, 48(1), 247-265. https://doi.org/10.32604/csse.2023.040194
Received 08 March 2023; Accepted 27 April 2023; Issue published 26 January 2024
Abstract
Federated learning is a distributed machine learning framework that solves data security and data island problems faced by artificial intelligence. However, federated learning frameworks are not always secure, and attackers can attack customer privacy information by analyzing parameters in the training process of federated learning models. To solve the problems of data security and availability during federated learning training, this paper proposes an Efficient Differential Privacy Federated Learning Algorithm based on early stopping mechanism (Efficient DP-FL). This method inherits the advantages of differential privacy and federated learning and improves the performance of model training while protecting the parameter information uploaded by the client during the training process. Specifically, in the federated learning framework, this article uses an adaptive DP-FL method for gradient descent training, which makes the model converge faster than traditional stochastic gradient descent. In addition, due to model convergence, noise should be reduced accordingly. This paper introduces an early stopping mechanism to improve data availability. This paper demonstrates the performance improvement of the Efficient DP-FL algorithm through simulation experiments on real MNIST and Fashion-MNIST datasets. Experimental show that the efficient DP-FL algorithm is significantly superior to other algorithms.Keywords
Cite This Article
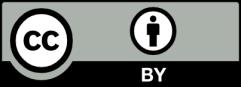
This work is licensed under a Creative Commons Attribution 4.0 International License , which permits unrestricted use, distribution, and reproduction in any medium, provided the original work is properly cited.