Open Access
ARTICLE
RLAT: Lightweight Transformer for High-Resolution Range Profile Sequence Recognition
College of Air and Missile Defense, Air Force Engineering University, Xi’an, 710051, China
* Corresponding Author: Xiaodan Wang. Email:
(This article belongs to the Special Issue: Pattern Recognition Based on Machine Learning)
Computer Systems Science and Engineering 2024, 48(1), 217-246. https://doi.org/10.32604/csse.2023.039846
Received 20 February 2023; Accepted 20 April 2023; Issue published 26 January 2024
Abstract
High-resolution range profile (HRRP) automatic recognition has been widely applied to military and civilian domains. Present HRRP recognition methods have difficulty extracting deep and global information about the HRRP sequence, which performs poorly in real scenes due to the ambient noise, variant targets, and limited data. Moreover, most existing methods improve the recognition performance by stacking a large number of modules, but ignore the lightweight of methods, resulting in over-parameterization and complex computational effort, which will be challenging to meet the deployment and application on edge devices. To tackle the above problems, this paper proposes an HRRP sequence recognition method based on a lightweight Transformer named RLAT, which consists of rotary position encoding, local-aggregated attention unit (LAU), and lightweight feedforward neural network (LW-FFN). Rotary position encoding is utilized to embed the relative position information for the HRRP sequence. Local aggregation attention unit can effectively aggregate and extract local features by local group linear transformation, and then the self-attention mechanism is adopted for perception and enhancement of global information. Thereby, the enhanced features are extracted by lightweight FFN. In addition, this paper adopts Label Smoothing regularization to add noise to the sample labels, which can improve the generalization performance of the method. Finally, the effectiveness of the proposed method in real scenes is verified based on the MSTAR dataset, a real-world dataset for radar target recognition. Experimental results show that the proposed method achieves superior recognition performance compared to other remarkable methods and achieves significant generalization performance and robustness under variant sample and limited sample conditions. RLAT achieved an accuracy of 99.86% on the MSTAR standard dataset and 99.73% on the MSTAR variant dataset. In particular, it achieves an accuracy of 95.83% with only 274 training samples. Furthermore, the proposed method is more lightweight, with 90.90% reduction in the number of parameters and 96.70% reduction in the computation compared to the Vanilla Transformer, which facilitates deployment in edge devices.Keywords
Cite This Article
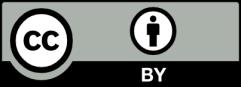
This work is licensed under a Creative Commons Attribution 4.0 International License , which permits unrestricted use, distribution, and reproduction in any medium, provided the original work is properly cited.