Open Access
ARTICLE
Fine-Tuned Extra Tree Classifier for Thermal Comfort Sensation Prediction
1 Department of Computer Engineering and Networks, College of Computer and Information Sciences, Jouf University, Sakaka, 72388, Saudi Arabia
2 School of Telecommunication Engineering, Suranaree University of Technology, Nakhon Ratchasima, 30000, Thailand
3 Department of Management Information Systems, Prince Sattam Bin Abdulaziz University, Al Kharaj, Riyadh, Saudi Arabia
4 Information Systems Department, Faculty of Management, Comenius University in Bratislava, Odbojárov, Bratislava, 440, Slovakia
5 Faculty of Computers & Information Technology, Computer Science Department, University of Tabuk, Tabuk, 71491, Saudi Arabia
6 Department of Computer Science and Software Engineering, International Islamic University, Islamabad, 44000, Pakistan
7 Department of Electrical Engineering, College of Engineering, Jouf University, Sakaka, 72388, Saudi Arabia
* Corresponding Authors: Chitapong Wechtaisong. Email: ; Natalia Kryvinska. Email:
Computer Systems Science and Engineering 2024, 48(1), 199-216. https://doi.org/10.32604/csse.2023.039546
Received 04 February 2023; Accepted 23 May 2023; Issue published 26 January 2024
A correction of this article was approved in:
Correction: Fine-Tuned Extra Tree Classifier for Thermal Comfort Sensation Prediction
Read correction
Abstract
Thermal comfort is an essential component of smart cities that helps to upgrade, analyze, and realize intelligent buildings. It strongly affects human psychological and physiological levels. Residents of buildings suffer stress because of poor thermal comfort. Buildings frequently use Heating, Ventilation, and Air Conditioning (HVAC) systems for temperature control. Better thermal states directly impact people’s productivity and health. This study revealed a human thermal comfort model that makes better predictions of thermal sensation by identifying essential features and employing a tuned Extra Tree classifier, MultiLayer Perceptron (MLP) and Naive Bayes (NB) models. The study employs the ASHRAE RP-884 standard dataset for experimentation and analysis, which is available to the public. Exploratory Data Analysis (EDA) is performed to examine the outliers and anomalies in the dataset. The Synthetic Minority Over-Sampling Technique (SMOTE) enhances the minority class’s interpretation. The proposed Extra Tree classifier outperforms by achieving an accuracy of 94%. The experiment shows that the suggested model is superior to other established methods and state-of-the-art.Keywords
Cite This Article
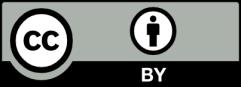
This work is licensed under a Creative Commons Attribution 4.0 International License , which permits unrestricted use, distribution, and reproduction in any medium, provided the original work is properly cited.