Open Access
ARTICLE
Cybersecurity Threats Detection Using Optimized Machine Learning Frameworks
1 Department of Information Systems, College of Computing and Information Technology, University of Bisha, P. O. Box 551, Bisha, 61922, Saudi Arabia
2 Department of Computer Science, College of Computing and Information Technology, University of Bisha, P. O. Box 551, Bisha, 61922, Saudi Arabia
3 Department of Computer Science, College of Science and Arts in Qurayyat, Jouf University, Sakaka, 75911, Saudi Arabia
4 Department of Information Systems, Faculty of Computer and Information, Assiut University, Assiut, 71515, Egypt
5 Department of Computer Science, Faculty of Computer and Information, Assiut University, Assiut, 71515, Egypt
* Corresponding Author: Nadir Omer. Email:
(This article belongs to the Special Issue: Explainable AI and Cybersecurity Techniques for IoT-Based Medical and Healthcare Applications)
Computer Systems Science and Engineering 2024, 48(1), 77-95. https://doi.org/10.32604/csse.2023.039265
Received 19 January 2023; Accepted 13 April 2023; Issue published 26 January 2024
Abstract
Today’s world depends on the Internet to meet all its daily needs. The usage of the Internet is growing rapidly. The world is using the Internet more frequently than ever. The hazards of harmful attacks have also increased due to the growing reliance on the Internet. Hazards to cyber security are actions taken by someone with malicious intent to steal data, destroy computer systems, or disrupt them. Due to rising cyber security concerns, cyber security has emerged as the key component in the fight against all online threats, forgeries, and assaults. A device capable of identifying network irregularities and cyber-attacks is intrusion detection. Several techniques have been created for Intrusion Detection Systems (IDS). There are elements in their effectiveness. Nevertheless, that provides room for more study. Finding an automatic method for detecting cyber-attacks is one of the biggest problems in cyber security. The recent trend is that the Machine Learning (ML) method has been demonstrated to be superior to conventional methods for IDS. Utilizing machine learning approaches, an effective intrusion prevention system will be designed. This research assessed different intrusion detection classification systems with particular applications. Before using ML classifiers for the classification process, the matrix factorization step of the Particle Swarm Optimization (PSO) technique was carried out. The categorization methods used in this study to classify network abnormalities were taken into consideration. Particle Swarm Optimization and Support Vector Machine classifiers (PSO + SVM) will be utilized in the proposed approach. The KDD-CUP 99 dataset will be used to confirm the results of the recognition algorithms. Due to the implementation, several performance metrics will be evaluated for various cyber-attack types, including specificity, recall, F1-score, accuracy, precision, and reliability.Keywords
Cite This Article
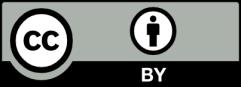