Open Access
ARTICLE
An Adaptive Edge Detection Algorithm for Weed Image Analysis
1 College of Computer and Information Sciences, Jouf University, Sakaka, Aljouf, 73211, Saudi Arabia
2 Department of Computer Science, Islamia College (Chartered University), Peshawar, 25000, Pakistan
* Corresponding Author: Yousef Alhwaiti. Email:
Computer Systems Science and Engineering 2023, 47(3), 3011-3031. https://doi.org/10.32604/csse.2023.042110
Received 18 May 2023; Accepted 04 July 2023; Issue published 09 November 2023
Abstract
Weeds are one of the utmost damaging agricultural annoyers that have a major influence on crops. Weeds have the responsibility to get higher production costs due to the waste of crops and also have a major influence on the worldwide agricultural economy. The significance of such concern got motivation in the research community to explore the usage of technology for the detection of weeds at early stages that support farmers in agricultural fields. Some weed methods have been proposed for these fields; however, these algorithms still have challenges as they were implemented against controlled environments. Therefore, in this paper, a weed image analysis approach has been proposed for the system of weed classification. In this system, for preprocessing, a Homomorphic filter is exploited to diminish the environmental factors. While, for feature extraction, an adaptive feature extraction method is proposed that exploited edge detection. The proposed technique estimates the directions of the edges while accounting for non-maximum suppression. This method has several benefits, including its ease of use and ability to extend to other types of features. Typically, low-level details in the form of features are extracted to identify weeds, and additional techniques for detecting cultured weeds are utilized if necessary. In the processing of weed images, certain edges may be verified as a footstep function, and our technique may outperform other operators such as gradient operators. The relevant details are extracted to generate a feature vector that is further given to a classifier for weed identification. Finally, the features have been used in logistic regression for weed classification. The model was assessed against logistic regression that accurately identified different kinds of weed images in naturalistic domains. The proposed approach attained weighted average recognition of 98.5% against the weed images dataset. Hence, it is assumed that the proposed approach might help in the weed classification system to accurately identify narrow and broad weeds taken captured in real environments.Keywords
Cite This Article
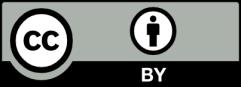
This work is licensed under a Creative Commons Attribution 4.0 International License , which permits unrestricted use, distribution, and reproduction in any medium, provided the original work is properly cited.