Open Access
ARTICLE
Enhanced 3D Point Cloud Reconstruction for Light Field Microscopy Using U-Net-Based Convolutional Neural Networks
1 Department of Information and Communication Engineering, Chungbuk National University, Cheongju-si, Chungcheongbuk-do, 28644, Korea
2 Department of Electronic Engineering, University of Suwon, Hwaseong-si, Gyeonggi-do, 18323, Korea
* Corresponding Author: Nam Kim. Email:
(This article belongs to the Special Issue: Advanced Machine Learning and Artificial Intelligence in Engineering Applications)
Computer Systems Science and Engineering 2023, 47(3), 2921-2937. https://doi.org/10.32604/csse.2023.040205
Received 08 March 2023; Accepted 17 May 2023; Issue published 09 November 2023
Abstract
This article describes a novel approach for enhancing the three-dimensional (3D) point cloud reconstruction for light field microscopy (LFM) using U-net architecture-based fully convolutional neural network (CNN). Since the directional view of the LFM is limited, noise and artifacts make it difficult to reconstruct the exact shape of 3D point clouds. The existing methods suffer from these problems due to the self-occlusion of the model. This manuscript proposes a deep fusion learning (DL) method that combines a 3D CNN with a U-Net-based model as a feature extractor. The sub-aperture images obtained from the light field microscopy are aligned to form a light field data cube for preprocessing. A multi-stream 3D CNNs and U-net architecture are applied to obtain the depth feature from the directional sub-aperture LF data cube. For the enhancement of the depth map, dual iteration-based weighted median filtering (WMF) is used to reduce surface noise and enhance the accuracy of the reconstruction. Generating a 3D point cloud involves combining two key elements: the enhanced depth map and the central view of the light field image. The proposed method is validated using synthesized Heidelberg Collaboratory for Image Processing (HCI) and real-world LFM datasets. The results are compared with different state-of-the-art methods. The structural similarity index (SSIM) gain for boxes, cotton, pillow, and pens are 0.9760, 0.9806, 0.9940, and 0.9907, respectively. Moreover, the discrete entropy (DE) value for LFM depth maps exhibited better performance than other existing methods.Keywords
Cite This Article
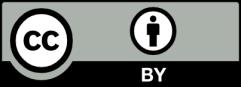
This work is licensed under a Creative Commons Attribution 4.0 International License , which permits unrestricted use, distribution, and reproduction in any medium, provided the original work is properly cited.