Open Access
ARTICLE
Liver Tumor Segmentation Based on Multi-Scale and Self-Attention Mechanism
School of Computer Science and Cyber Engineering, Guangzhou University, Guangzhou, 510006, China
* Corresponding Author: Manlin Luo. Email:
Computer Systems Science and Engineering 2023, 47(3), 2835-2850. https://doi.org/10.32604/csse.2023.039765
Received 15 February 2023; Accepted 17 April 2023; Issue published 09 November 2023
Abstract
Liver cancer has the second highest incidence rate among all types of malignant tumors, and currently, its diagnosis heavily depends on doctors’ manual labeling of CT scan images, a process that is time-consuming and susceptible to subjective errors. To address the aforementioned issues, we propose an automatic segmentation model for liver and tumors called Res2Swin Unet, which is based on the Unet architecture. The model combines Attention-Res2 and Swin Transformer modules for liver and tumor segmentation, respectively. Attention-Res2 merges multiple feature map parts with an Attention gate via skip connections, while Swin Transformer captures long-range dependencies and models the input globally. And the model uses deep supervision and a hybrid loss function for faster convergence. On the LiTS2017 dataset, it achieves better segmentation performance than other models, with an average Dice coefficient of 97.0% for liver segmentation and 81.2% for tumor segmentation.Keywords
Cite This Article
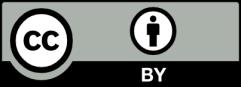
This work is licensed under a Creative Commons Attribution 4.0 International License , which permits unrestricted use, distribution, and reproduction in any medium, provided the original work is properly cited.