Open Access
ARTICLE
Deep Learning-Based Stacked Auto-Encoder with Dynamic Differential Annealed Optimization for Skin Lesion Diagnosis
Department of Medical Equipment Technology, College of Applied Medical Sciences, Majmaah University, Al Majmaah, 11952, Saudi Arabia
* Corresponding Author: Ahmad Alassaf. Email:
Computer Systems Science and Engineering 2023, 47(3), 2773-2789. https://doi.org/10.32604/csse.2023.035899
Received 08 September 2022; Accepted 13 January 2023; Issue published 09 November 2023
Abstract
Intelligent diagnosis approaches with shallow architectural models play an essential role in healthcare. Deep Learning (DL) models with unsupervised learning concepts have been proposed because high-quality feature extraction and adequate labelled details significantly influence shallow models. On the other hand, skin lesion-based segregation and disintegration procedures play an essential role in earlier skin cancer detection. However, artefacts, an unclear boundary, poor contrast, and different lesion sizes make detection difficult. To address the issues in skin lesion diagnosis, this study creates the UDLS-DDOA model, an intelligent Unsupervised Deep Learning-based Stacked Auto-encoder (UDLS) optimized by Dynamic Differential Annealed Optimization (DDOA). Pre-processing, segregation, feature removal or separation, and disintegration are part of the proposed skin lesion diagnosis model. Pre-processing of skin lesion images occurs at the initial level for noise removal in the image using the Top hat filter and painting methodology. Following that, a Fuzzy C-Means (FCM) segregation procedure is performed using a Quasi-Oppositional Elephant Herd Optimization (QOEHO) algorithm. Besides, a novel feature extraction technique using the UDLS technique is applied where the parameter tuning takes place using DDOA. In the end, the disintegration procedure would be accomplished using a SoftMax (SM) classifier. The UDLS-DDOA model is tested against the International Skin Imaging Collaboration (ISIC) dataset, and the experimental results are examined using various computational attributes. The simulation results demonstrated that the UDLS-DDOA model outperformed the compared methods significantly.Keywords
Cite This Article
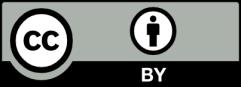