Open Access
ARTICLE
An Optimized Feature Selection and Hyperparameter Tuning Framework for Automated Heart Disease Diagnosis
Applied College, Computer Science, and Information Department, Taibah University, Medinah, 41461, Saudi Arabia
* Corresponding Author: Saleh Ateeq Almutairi. Email:
Computer Systems Science and Engineering 2023, 47(2), 2599-2624. https://doi.org/10.32604/csse.2023.041609
Received 29 April 2023; Accepted 12 June 2023; Issue published 28 July 2023
Abstract
Heart disease is a primary cause of death worldwide and is notoriously difficult to cure without a proper diagnosis. Hence, machine learning (ML) can reduce and better understand symptoms associated with heart disease. This study aims to develop a framework for the automatic and accurate classification of heart disease utilizing machine learning algorithms, grid search (GS), and the Aquila optimization algorithm. In the proposed approach, feature selection is used to identify characteristics of heart disease by using a method for dimensionality reduction. First, feature selection is accomplished with the help of the Aquila algorithm. Then, the optimal combination of the hyperparameters is selected using grid search. The experiments were conducted with three datasets from Kaggle: The Heart Failure Prediction Dataset, Heart Disease Binary Classification, and Heart Disease Dataset. Two classes can be distinguished: diseased and healthy (i.e., uninfected). The Histogram Gradient Boosting (HGB) classifier produced the highest Weighted Sum Metric (WSM) scores of 98.65% concerning the Heart Failure Prediction Dataset. In contrast, the Decision Tree (DT) machine learning classifier had the highest WSM scores of 87.64% concerning the Heart Disease Health Indicators Dataset. Measures of accuracy, specificity, sensitivity, and other metrics are used to evaluate the proposed approach. The presented method demonstrates superior performance compared to different state-of-the-art algorithms.Keywords
Cite This Article
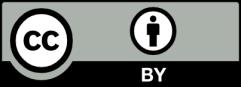