Open Access
ARTICLE
A PSO Improved with Imbalanced Mutation and Task Rescheduling for Task Offloading in End-Edge-Cloud Computing
1 Faculty of Engineering, Huanghe Science and Technology University, Zhengzhou 450003, China
2 Computer School, Beijing Information Science and Technology University, Beijing, 100192, China
3 Software Engineering College, Zhengzhou University of Light Industry, Zhengzhou, 450001, China
* Corresponding Author: Bo Wang. Email:
Computer Systems Science and Engineering 2023, 47(2), 2259-2274. https://doi.org/10.32604/csse.2023.041454
Received 23 April 2023; Accepted 31 May 2023; Issue published 28 July 2023
Abstract
To serve various tasks requested by various end devices with different requirements, end-edge-cloud (E2C) has attracted more and more attention from specialists in both academia and industry, by combining both benefits of edge and cloud computing. But nowadays, E2C still suffers from low service quality and resource efficiency, due to the geographical distribution of edge resources and the high dynamic of network topology and user mobility. To address these issues, this paper focuses on task offloading, which makes decisions that which resources are allocated to tasks for their processing. This paper first formulates the problem into binary non-linear programming and then proposes a particle swarm optimization (PSO)-based algorithm to solve the problem. The proposed algorithm exploits an imbalance mutation operator and a task rescheduling approach to improve the performance of PSO. The proposed algorithm concerns the resource heterogeneity by correlating the probability that a computing node is decided to process a task with its capacity, by the imbalance mutation. The task rescheduling approach improves the acceptance ratio for a task offloading solution, by reassigning rejected tasks to computing nodes with available resources. Extensive simulated experiments are conducted. And the results show that the proposed offloading algorithm has an 8.93%–37.0% higher acceptance ratio than ten of the classical and up-to-date algorithms, and verify the effectiveness of the imbalanced mutation and the task rescheduling.Keywords
Cite This Article
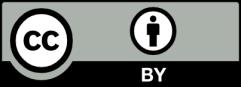
This work is licensed under a Creative Commons Attribution 4.0 International License , which permits unrestricted use, distribution, and reproduction in any medium, provided the original work is properly cited.