Open Access
ARTICLE
Dimensionality Reduction Using Optimized Self-Organized Map Technique for Hyperspectral Image Classification
School of Computer Science and Engineering, VIT University, Vellore, 632014, India
* Corresponding Author: K. Rajakumar. Email:
Computer Systems Science and Engineering 2023, 47(2), 2481-2496. https://doi.org/10.32604/csse.2023.040817
Received 31 March 2023; Accepted 25 May 2023; Issue published 28 July 2023
Abstract
The high dimensionalhyperspectral image classification is a challenging task due to the spectral feature vectors. The high correlation between these features and the noises greatly affects the classification performances. To overcome this, dimensionality reduction techniques are widely used. Traditional image processing applications recently propose numerous deep learning models. However, in hyperspectral image classification, the features of deep learning models are less explored. Thus, for efficient hyperspectral image classification, a depth-wise convolutional neural network is presented in this research work. To handle the dimensionality issue in the classification process, an optimized self-organized map model is employed using a water strider optimization algorithm. The network parameters of the self-organized map are optimized by the water strider optimization which reduces the dimensionality issues and enhances the classification performances. Standard datasets such as Indian Pines and the University of Pavia (UP) are considered for experimental analysis. Existing dimensionality reduction methods like Enhanced Hybrid-Graph Discriminant Learning (EHGDL), local geometric structure Fisher analysis (LGSFA), Discriminant Hyper-Laplacian projection (DHLP), Group-based tensor model (GBTM), and Lower rank tensor approximation (LRTA) methods are compared with proposed optimized SOM model. Results confirm the superior performance of the proposed model of 98.22% accuracy for the Indian pines dataset and 98.21% accuracy for the University of Pavia dataset over the existing maximum likelihood classifier, and Support vector machine (SVM).
Keywords
Cite This Article
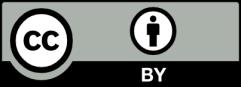
This work is licensed under a Creative Commons Attribution 4.0 International License , which permits unrestricted use, distribution, and reproduction in any medium, provided the original work is properly cited.