Open Access
ARTICLE
A Triplet-Branch Convolutional Neural Network for Part-Based Gait Recognition
1 Department of Computer Engineering, Mokwon University, Daejeon, Korea
2 Department of Industrial Security, Chung-Ang University, Seoul, 06974, Korea
3 Department of Computer Engineering, Semyung University, Jechun-si, Korea
4 Department of Computer Science, UET Taxila, Taxila, Pakistan
5 Department of Computer Science, COMSATS University Islamabad, Attock Campus, Attock, Pakistan
* Corresponding Author: Seungmin Rho. Email:
Computer Systems Science and Engineering 2023, 47(2), 2027-2047. https://doi.org/10.32604/csse.2023.040327
Received 14 March 2023; Accepted 15 May 2023; Issue published 28 July 2023
Abstract
Intelligent vision-based surveillance systems are designed to deal with the gigantic volume of videos captured in a particular environment to perform the interpretation of scenes in form of detection, tracking, monitoring, behavioral analysis, and retrievals. In addition to that, another evolving way of surveillance systems in a particular environment is human gait-based surveillance. In the existing research, several methodological frameworks are designed to use deep learning and traditional methods, nevertheless, the accuracies of these methods drop substantially when they are subjected to covariate conditions. These covariate variables disrupt the gait features and hence the recognition of subjects becomes difficult. To handle these issues, a region-based triplet-branch Convolutional Neural Network (CNN) is proposed in this research that is focused on different parts of the human Gait Energy Image (GEI) including the head, legs, and body separately to classify the subjects, and later on, the final identification of subjects is decided by probability-based majority voting criteria. Moreover, to enhance the feature extraction and draw the discriminative features, we have added soft attention layers on each branch to generate the soft attention maps. The proposed model is validated on the CASIA-B database and findings indicate that part-based learning through triplet-branch CNN shows good performance of 72.98% under covariate conditions as well as also outperforms single-branch CNN models.Keywords
Cite This Article
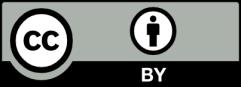
This work is licensed under a Creative Commons Attribution 4.0 International License , which permits unrestricted use, distribution, and reproduction in any medium, provided the original work is properly cited.