Open Access
ARTICLE
Performance Analysis of Intelligent Neural-Based Deep Learning System on Rank Images Classification
1 College of Computer and Information Sciences, Jouf University, Sakaka, Aljouf, 73211, Kingdom of Saudi Arabia
2 Institute of Computer Science and Information Technology, ICS/IT FMCS, University of Agriculture, Peshawar, 25000, Pakistan
* Corresponding Author: Muhammad Hameed Siddiqi. Email:
Computer Systems Science and Engineering 2023, 47(2), 2219-2239. https://doi.org/10.32604/csse.2023.040212
Received 09 March 2023; Accepted 25 May 2023; Issue published 28 July 2023
Abstract
The use of the internet is increasing all over the world on a daily basis in the last two decades. The increase in the internet causes many sexual crimes, such as sexual misuse, domestic violence, and child pornography. Various research has been done for pornographic image detection and classification. Most of the used models used machine learning techniques and deep learning models which show less accuracy, while the deep learning model ware used for classification and detection performed better as compared to machine learning. Therefore, this research evaluates the performance analysis of intelligent neural-based deep learning models which are based on Convolution neural network (CNN), Visual geometry group (VGG-16), VGG-14, and Residual Network (ResNet-50) with the expanded dataset, trained using transfer learning approaches applied in the fully connected layer for datasets to classify rank (Pornographic vs. Nonpornographic) classification in images. The simulation result shows that VGG-16 performed better than the used model in this study without augmented data. The VGG-16 model with augmented data reached a training and validation accuracy of 0.97, 0.94 with a loss of 0.070, 0.16. The precision, recall, and f-measure values for explicit and non-explicit images are (0.94, 0.94, 0.94) and (0.94, 0.94, 0.94). Similarly, The VGG-14 model with augmented data reached a training and validation accuracy of 0.98, 0.96 with a loss of 0.059, 0.11. The f-measure, recall, and precision values for explicit and non-explicit images are (0.98, 0.98, 0.98) and (0.98, 0.98, 0.98). The CNN model with augmented data reached a training and validation accuracy of 0.776 & 0.78 with losses of 0.48 & 0.46. The f-measure, recall, and precision values for explicit and non-explicit images are (0.80, 0.80, 0.80) and (0.78, 0.79, 0.78). The ResNet-50 model with expanded data reached with training accuracy of 0.89 with a loss of 0.389 and 0.86 of validation accuracy and a loss of 0.47. The f-measure, recall, and precision values for explicit and non-explicit images are (0.86, 0.97, 0.91) and (0.86, 0.93, 0.89). Where else without augmented data the VGG-16 model reached a training and validation accuracy of 0.997, 0.986 with a loss of 0.008, 0.056. The f-measure, recall, and precision values for explicit and non-explicit images are (0.94, 0.99, 0.97) and (0.99, 0.93, 0.96) which outperforms the used models with the augmented dataset in this study.Keywords
Cite This Article
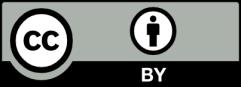
This work is licensed under a Creative Commons Attribution 4.0 International License , which permits unrestricted use, distribution, and reproduction in any medium, provided the original work is properly cited.