Open Access
ARTICLE
Systematic Survey on Big Data Analytics and Artificial Intelligence for COVID-19 Containment
1 Department of Computer Science, College Computing and Information Technology, Shaqra University, Shaqra, Saudi Arabia
2 Department of Computer Science, College of Computer in Al-Lith, Umm Al-Qura University, Makkah, Saudi Arabia
3 High-Performance Computing Center, King Abdulaziz University, Jeddah, Saudi Arabia
* Corresponding Author: Nayyar Ahmed Khan. Email:
Computer Systems Science and Engineering 2023, 47(2), 1793-1817. https://doi.org/10.32604/csse.2023.039648
Received 09 February 2023; Accepted 09 May 2023; Issue published 28 July 2023
Abstract
Artificial Intelligence (AI) has gained popularity for the containment of COVID-19 pandemic applications. Several AI techniques provide efficient mechanisms for handling pandemic situations. AI methods, protocols, data sets, and various validation mechanisms empower the users towards proper decision-making and procedures to handle the situation. Despite so many tools, there still exist conditions in which AI must go a long way. To increase the adaptability and potential of these techniques, a combination of AI and Bigdata is currently gaining popularity. This paper surveys and analyzes the methods within the various computational paradigms used by different researchers and national governments, such as China and South Korea, to fight against this pandemic. The process of vaccine development requires multiple medical experiments. This process requires analyzing datasets from different parts of the world. Deep learning and the Internet of Things (IoT) revolutionized the field of disease diagnosis and disease prediction. The accurate observations from different datasets across the world empowered the process of drug development and drug repurposing. To overcome the issues generated by the pandemic, using such sophisticated computing paradigms such as AI, Machine Learning (ML), deep learning, Robotics and Bigdata is essential.Keywords
This study comprises essential and interesting facts about the containment of COVID-19. Using computational paradigms such as big data and artificial Intelligence, along with machine learning algorithms, can be very fruitful and helpful for this containment. This research aimed to identify all possible areas in which computer systems can be important for healthcare units. The present situation for the digital technology used to contain and identify various diseases should be benchmarked by nations. The use of sophisticated devices and machine learning will be constructive for the entire humanity. The unavailability of data from various healthcare units was unavailable due to several research gaps. The data from sophisticated means and sources was not achievable. The use of technology and computational paradigms is very helpful for collecting data and analyzing them for the containment for diseases like Covid-19.
A cluster of pneumonia cases from Wuhan, China, was reported to the World Health Organization (WHO) on 31 December 2019. The following January, a virus belonging to the coronavirus family caused this pneumonia [1]. The new disease was named Coronavirus Disease 2019 (COVID-19) by the WHO, while the causing virus disease was named SARS-CoV-2. The condition halted almost all outdoor activities in weeks, from international travel to leaving a patient home for everyday tasks. It has affected the economy and infected over one million people worldwide [2]. COVID-19 containment is posing severe challenges to the authorities of every country. The typical response to new diseases is medical experiments to detect, diagnose, and develop drugs. Therefore, governments should adopt new technologies to help in COVID-19 containment. These technologies include Big Data Analytics techniques, Machine learning (ML), IoT, and Robotics. However, the volume of data available to process is increasing every day. Therefore, organizations must refrain from using traditional techniques to analyze it because of the size, variety, and speed at which new data is being added. In addition, medical datasets have large dimensionality, which means conventional data analytics techniques become irrelevant because of challenges applying those techniques to these datasets [3].
Consequently, Big Data Analytics techniques are used for this purpose as they have shown effective results. Along with the rise of Big Data analytics, a subfield of artificial Intelligence named machine learning has emerged. It has shown potential in many fields, such as Natural Language Processing, Marketing, Sentiment Analysis, and Time Series Forecasting. Subsequently, many areas of the healthcare industry have started using machine learning for various purposes, such as disease diagnosis, prognosis, and drug development. The past decade’s improvements in data storage and computational resources have enabled machine learning to play a significant role in medicine [4]. Medical professionals are trying to contain the pandemic through multiple technologies, including telemedicine and the deployment of robots. However, progress in computer vision techniques provides a better understanding. Computer vision techniques can use 3D and 4D information by exploiting contour, shape, texture, and prior knowledge of medical image sequences [5]. Many IoT-enabled thermometers have been employed to monitor body temperatures as early detection of COVID-19. These easy-to-use devices take accurate measurements, be worn under clothes, and are available in various forms such as patch, touch, and radiometric [6]. Besides, electrocardiograms, glucose monitors, thermometers, and ultrasounds are becoming connected via the IoT paradigm, which helps doctors monitor patients’ health in real-time [7]. As per [8,9], intelligent bot systems for COVID-19 detection and prevention were designed to report the infection and provide proper diagnostics. Reference [10] suggested many mechanisms relative to industrial IOT-based services that are used in the medical industry. Various technical systems are expected to detect several vulnerabilities [11]. There are multiple methods suggested to overcome such vulnerabilities by the author.
The main objective of this paper is an in-depth analysis of various technologies and methods used for COVID-19 containment. First, a background about coronavirus is given in Section 2. Next, Section 3 discusses the adopted methodology. Section 4 details the analysis of current datasets, Big Data Analytics, Machine learning, IoT, and Robotics. Then, Section 5 discusses their effectiveness in providing support in detecting, measuring, and tracking disease progression. Finally, the paper is concluded in Section 6.
During 2002–2003, Severe Acute Respiratory Syndrome (SARS) emerged as a coronavirus from southern China and spread across multiple countries [12]. SARS-CoV-2 attacked humanity at the end of 2019, causing the COVID-19 diseases, and many countries are struggling to counter this pandemic. Over the past decades, the virus has caused illnesses such as Middle East Respiratory Syndrome (MERS), SARS, and many deaths. However, COVID-19 has affected the world more severely than any other coronavirus due to its fast transmission to humans. The name of the virus is due to phylogenic analysis and whole-genome sequencing revealing that SARS-CoV-2 belongs to the same subgenus as SARS but is in a different clade [13]. The article focuses primarily on the factors which led to the non-identification of respiratory syndrome diseases such as SARS, MERS etc. The research gap revealed that the data from various healthcare units remained undiscovered. The use of technology and simplifying this to a greater extent. The article tends to identify such factors and technologies which can provide proper solution to collection of clinical data and sophisticated prediction techniques to identify the disease.
Furthermore, the receptor-binding gene structure resembles the structure of the SARS coronavirus, and for cell entry, the virus uses the same receptor, namely the Angiotensin-Converting Enzyme 2 (ACE2) [14]. After exposure to SARS-CoV-2, the assumed incubation period of COVID-19 is within 14 days, whereas most known cases occur within 4–5 days [15]. According to the WHO, patients with mild disease can recover in 2 weeks, while it takes up to 6 weeks for patients with severe infections [16]. WHO suggested that the original SARS outbreak was contained on 05 July 2003, causing 774 deaths from 8,096 cases [16]. It spread across 29 countries and had an overall Case-Fatality Rate (CFR) of 9.6%. On the other hand, MERS is responsible for eight hundred and fifty-eight deaths from 2,496 cases across 27 countries, having 34.4% CFR, and it has yet to be contained. Even though SARS and MERS have higher CFRs when compared with COVID-19, the total number of COVID-19 cases worldwide is several orders of magnitude greater and, as a result, has led to more overall deaths, and these numbers continue to grow [17]. Despite of the statistics available there is still a possibility to identify and create the combinatorial drugs needed for the remedy of the diseases that were rising.
Until now, no clinical features have been used by healthcare to distinguish COVID-19 specifically. A study of 138 confirmed COVID-19 patients from Wuhan, China, found 36 patients admitted to the intensive care unit (ICU). Medical tests of these patients revealed that 98.6% experienced fever, 69.4% had fatigue and dry cough, anorexia, myalgias, dyspnea, and expectoration were experienced by 59.4%, 39.9%, 34.8%, 31.2%, and 26.8% patients, respectively (see Table 1). The table represents all the visible symptoms in a Covid-infected patient. Patients also experienced other respiratory symptoms, such as sore throat and headache, which are uncommon. Other relatively unusual symptoms reported in a few patients are gastrointestinal problems such as diarrhea and nausea [18]. The symptoms mentioned above can be experienced from mild to severe by different patients. The same study [18] revealed that patients with severe disease could also develop Acute Respiratory Distress Syndrome (ARDS). Among the 138 patients of the study mentioned above, ARDS developed in 20%. In addition, ground-glass opacification is presented in chest Computed Tomography (CT) of COVID-19 patients. Therefore, chest CT [19] is helpful for diagnosis. Still, no test results cannot rule out or in the possibility of COVID-19 as there are no apparent symptoms specifically related to the disease. There is another scope to use several technologies like AI and ML to identify the nature of infection that a patient is suffering from. This was supposed to be implemented on the data sets received from several healthcare units. However due to a research gap in the previous two decades, the use of technology remained a step back in the identification and containment of infectious diseases.
As discussed, the pandemic exposes vast amounts of information analysts should process to help humanity. New IT and Artificial Intelligence should help discover, track, and contain the disease. The complication is due to many dimensions, including the limits of current technologies and the immature available datasets. Still, a successful COVID-19 containment solution depends on current information and datasets. Unfortunately, there need to be more data sources and expertise in labeling the data particular to this new virus strain in humans.
Nevertheless, the literature review illustrates the doors that could be opened to resolve open issues. Various researchers proposed several methods and models for detecting, identifying, and predicting the COVID-19 disease outbreak. However, this study identifies all the possible existing research and investigations for containment of big data, including artificial Intelligence towards COVID-19 containment. The analysis provided different results, which are discussed in the later sections below:
Inclusion Criteria:
(1) Studies must be published starting from January 2019 till March 2021.
(2) Studies must be written in the English Language.
(3) Studies must discuss the technology or an information-related approach for COVID-19 containment.
(4) Studies should be published work with a paper length of more than five pages.
Execution Criteria:
(1) Non-English papers.
(2) Papers that do not resolve an issue related to COVID-19 from a technical point of view.
(3) Short papers.
After carrying out the process, the result of this study reports 73 primary studies. Several articles were studied in this other than these primary studies. The study provides an in-depth explanation for several latest technologies that are arising up in the field of medical healthcare. The use of clinical data and technology discussed in this article provides a complete explanation for the COVID-19 containment. The study also explains the use of computational paradigms, such as bioinformatics, big data analytics, deep learning, IoT, and machine learning, in addressing the global COVID-19 pandemic.
4 Literature Review and Analysis of COVID-19 Containment
4.1 Availability of COVID–19 Datasets
Various government and non-government organizations are providing COVID-19 datasets, which are constantly updated to bring the most up-to-date information to the world on how quickly this disease is spreading and how many people worldwide have lost their lives. A few of these data sources and datasets are mentioned below. Centers for Disease Control and Prevention (CDC) is providing COVID-19 case data in the United States of America (USA), publishing a weekly surveillance summary [20]. The CDC is also providing information about the number of tests being conducted nationwide. The WHO provides information on infected and deceased cases for every country worldwide. An RNA-seq dataset of COVID-19 patients [21] is also available on Kaggle [22]. In addition, Microsoft Research, The Allen Institute for AI, The National Library of Medicine at the National Institute of Health, The White House Office of Science and Technology, and various other institutes collaborated to publish 29,000 scholarly articles related to coronavirus are available on the Kaggle website.
In the 21st century, a large amount of data can be used for various purposes due to recent developments in Information and Communication Technologies. This massive data comes from multiple sources such as social media, internet search trends, transportation dynamics, genome databases, human dynamics information, outbreak investigation reports, vaccinology-related data, and numerous sources. In addition, medical datasets have large dimensionality, which means conventional data analytics techniques become irrelevant because of challenges applying those techniques to these datasets [3]. Fig. 1 illustrates some Big Data analytics techniques to gain insights into COVID-19. All the methods stated in Fig. 1 contain different engagement techniques to handle COVID-19 infection using technology. They are grouped into four logical groups: disease detection and tracking, outbreak prediction, modeling, and visualization, and containing the spread.
Figure 1: Classification of big data Analytics methods for COVID-19 control
4.2.1 Disease Detection and Tracking
For several years, scientists have been tracking the spread of diseases through mapping tools. For example, the Influenza Net has been used since 2003 to track flu symptoms across ten European countries, while America started using Flu near you in 2011. Therefore, data from China, the US, Europe, and the WHO are visualized by dashboards tracking suspected and confirmed disease cases. In addition, social media is used for the early identification of a pandemic. For example, an online dashboard was launched by the Center of Systems Science and Engineering to track its spread [23]. Sally Elghamrawy’s [20] Deep-Learning-Inspired Model Based on Big Data Analytics (DLBD-COV) used H2O architecture that contained large-scale X-ray and CT image data to diagnose COVID-19. First, data is fed into the model from the Hadoop Distributed File System (HDFS) of the H2O architecture and then processed through GANs to generate even larger data for training neural networks. Finally, a state-of-the-art Convolutional Neural Network (CNN) architecture extracts the features associated with the COVID-19 disease. Then, in the classification phase, Support Vector Machine (SVM), Naive Bayes (NB), and Random Forest (RF) classifiers are applied to classify positive or negative cases. Accordingly, the Big Data Analytics method shows an outstanding accuracy of over 98 percent. During the Ebola epidemic that primarily affected the world’s poorest regions, Big Data tools and techniques were employed to predict the outbreak and determine the transmission rate and measures for disease prevention [24]. Research conducted to model the Ebola spread has also proven to be very effective in containing the spread of the coronavirus. Villanustre et al. [25] leveraged the experience from the Ebola model. They proposed a study that used the HPCC Big Data platform that instantly tracks various data sources and delivers them to the public. The platform also presents the statistical analysis of the data at a global or country level and visualizes it daily or weekly.
4.2.2 Modeling and Visualization
Researchers at the London School of Hygiene and Tropical Medicine modeled the spread of COVID-19. London School researchers developed a tool to map outbreaks while statistics published by the WHO are visualized online. The tool allows users to get back in time and visualize global statistics on any given day of the disease outbreak. It also enables users to compare ongoing diseases with previous episodes, including SARS, “swine” flu, and “Ebola” outbreaks [26]. A report was released based on COVID-19 by a WHO-China joint mission. Big Data analytics tools were used to present various aspects of the disease, such as epidemic curves and case fatality ratio of different scenarios generated through China’s National Infectious Disease Information System (IDIS). In addition, the Sankey diagram pattern of COVID-19 was visualized, which showed the detailed flow of recovered and deceased patients ranging from mild to critical severity. Measures taken by the Chinese government at the national level were also visualized [27]. Recently, some European researchers proposed a novel Meaningful Integration of Data Analytics and Services (MIDAS) platform that effectively responded to the coronavirus pandemic. It gathered data from various sources, including world news, social networking websites, published research, and local health data from public health organizations. It also provided valuable insights to the professionals working in public health departments. In addition, the platform was equipped with advanced data analytics methods and visualization tools that supported public health organizations across Europe in making policy decisions [28].
The MIDAS platform is based on the Apache Hadoop Framework, Apache Hive, and Apache Spark and was successfully tested throughout Europe by four pilot sites. It analyzed and assessed the impact of COVID-19 confinement restrictions, associated risks, and deprivation of healthcare services to the general population. A platform named COVID-19 SG used graph visualization techniques to visualize information about COVID-19 in Singapore [29]. Information such as how a specific patient became infected, the patient’s background information, and where they were admitted are used in the visualization. Other graphs on the platform indicate that more people were infected in Singapore because of those from other countries than local disease transmissions. While many of the proposed visualization models consider the human-to-human transmission of COVID-19 infection when modeling the pandemic, they do not emphasize critical epidemiological factors such as patient contact duration, social distancing, and other crucial COVID-19 characteristics [30]. By considering the mentioned epidemiological factors, a graph-based model can trace the undetected cases of the virus and help visualize the data starting from the first detected case of COVID-19. Thus, it allows the policymakers to make the right decisions at the right time and mitigate the spread.
Qin et al. [31] employed social media search indexes (SMSI) to identify new confirmed or suspected COVID-19 cases. SMSI compiles a list of keywords that surface on social networking platforms to predict new cases, including symptoms like pneumonia, cough, fever, and chest pain. People’s movement has been described as the primary mode of infection transmission; in this respect, social media posts may help identify any information related to the risk of contracting the virus. However, these social media posts may need to be more reliable and trustworthy in many cases, which is one of the most complex challenges of social media Big Data analytics. During the COVID-19 pandemic, population flow from one state to another has significantly contributed to the virus spread. By monitoring human movement, Li et al. [32] predicted the spatial spread of COVID-19. They used Big Data Analytics on the data from social media, geotagged Twitter data, and predicted the future risk of infection at different geospatial scales. As a result of their model, a Fused Neural Network (enclosing a CNN and LSTM network) has been used for the prediction. Therefore, public health officials, emergency responders, and researchers can use this study to solve various problems during the pandemic. Wu et al. [21] studied a 41-year-old patient admitted on 26 December last year to Wuhan central hospital with no history of diabetes, tuberculosis, or hepatitis. The patient was admitted to the hospital because he was experiencing severe respiratory syndrome, including cough, dizziness, and fever. A sample of bronchoalveolar lavage fluid was obtained for metagenomics RNA sequencing. The complete viral genome was analyzed through phylogenetic analysis, revealing that the virus had 89.1% nucleotide similarity to the coronaviruses group. The associated dataset with the previous search, available on Kaggle, was adopted by the research community analyzing the dataset that provides valuable insights.
In the current outbreak of COVID-19, there are reports that Taiwan has brought the spread of pathogens under control, even though the island is just 130 kilometers away from mainland China and thousands of people travel to and from the mainland each day. The main reason behind their success was that the country leveraged Big Data Analytics techniques right after the disease outbreak across customs and immigration databases integrated with the national health insurance database. During clinical visits, real-time alerts were generated to identify causes, and these alerts were based on a person’s clinical symptoms and travel history. Furthermore, travelers were classified based on the travel history of the last 14 days and flight origin by scanning QR codes, online reporting of health symptoms, and recent travel history [33]. Kumar Das et al. [34] conducted a detailed study to develop tools for data scientists and medical professionals to help them manage clinical data and tackle the pandemic. In their research, large-scale data has been processed through a pipeline of several Big Data Analytics algorithms and tools to develop a roadmap for the experts to help them handle situations like the COVID-19 pandemic. The vendors of “TigerGraph”—a graph database—are providing free licenses during this time of pandemic so that they can help fight COVID-19 by modeling its spread. Victor Lee, the head of product strategy and relations, mentioned the factors to take into consideration while working on this problem, such as getting the infection, being a patient neighbor, how many passengers are traveling by air every day on each route, chances of being contagious, the likelihood of showing symptoms and possibilities of dying [35].
Li et al. [32,36] tried to compute mean values of the Covid-19 main epidemiological parameters, including mortality rate, recovery, infection, and reproduction number, with 90% confidence intervals. However, as reported earlier, the number of infected and recovered patients were expected to be higher than reported. That is why another scenario was created by adding twenty times and forty times the number of registered and recovered cases, respectively. As a result of the above estimates, tentative forecasts were provided. Furthermore, a Susceptible-Infected-Recovered-Dead (SIRD) model was used to estimate the infection rate. During this global pandemic, it is crucial to understand interactions among places, infected people, and their means of transportation, such as automobiles, planes, and trains, so disease spread can be easily tracked. Graph databases exhibit the exceptional potential to visualize this information. A Chinese scientist developed an application using Neo4j, an open-source graph database, to help Chinese citizens with self-assessment. They can check whether a known carrier is related to them. Researchers put their efforts into travel data generated during the return of Chinese to their homes for New Year celebrations. Travelers had to take taxis, subways, buses, coaches, trains, or planes. It was bound to happen that they met someone with the disease, even if they were asymptomatic. Chinese citizens can know if they had close contact with an infected person by searching for their flight, train, or vehicle license number [36].
More than 29,000 scholarly articles related to the coronavirus family were released for free to be consumed by Natural Language Processing (NLP) and medical researchers so that insights to fight the pandemic can be extracted. This dataset results from a collaboration between The White House Office of Science and Technology, The National Library of Medicine at The National Institute of Health, The Allen Institute for AI, Microsoft Research, and various other institutes. An artificial intelligence platform named BlueDot identified a cluster of “unusual pneumonia” occurring around a market in Wuhan, China. This platform placed COVID-19 nine days before the WHO alerted the world about it at midnight on 30 December 2019. BlueDot uses ML and NLP to get data from a couple of hundred thousand data sources, such as population demographics, livestock health reports, global airline ticketing data, digital media, and official health organization statements. It can process this information every 15 min. Therefore, it is proprietary software to track, locate, and conceptualize the spread of infectious diseases. Yan et al. [37] proposed an XGBoost machine learning algorithm-based model to predict mortality risk caused by COVID-19. From more than 300 features, the proposed model identified three critical components, including high-sensitivity C-Reactive Protein (hs-CRP), Lymphocyte, and Lactic Dehydrogenase (LDH). The proposed model recognizes urgent cases to help medical specialists encounter them accordingly so that the mortality rate can be potentially reduced.
A Boston Children’s Hospital team is tracking disease by implementing ML techniques using data from official public health channels, news reports, social media posts, chatrooms, and blogs [38]. They specifically focus on social media posts where doctors have already reported COVID-19 cases, and they have provided a live map to track all reported cases. This collected data is organized, filtered, and tagged to develop the health map. Medicines that can treat patients of COVID-19 are urgently required so that patients can be treated. Experimental results of rapid sequencing and molecular modeling indicated that various compounds, such as anti-HIV lopinavir plus ritonavir combination, could be effective for the disease. The knowledge graph of Benevolent-AI used ML to accumulate a large amount of information related to structured medical information and various connections from scientific literature. To find approved drugs to help with COVID-19, Benevolent AI was searched [39]. Results obtained from the search by a suite of algorithms show that AP2-Associated Protein Kinase 1 (AAK1) can be inhibited by therapeutic dosing because there is plasma contraction of baricitinib. Furthermore, researchers suggested that both inflammation and viral entry of COVID-19 in patients can be reduced on a trial basis.
Researchers continued this work by examining the selectivity and affinity of approved drugs having anti-inflammatory and antiviral properties in the Benevolent-AI knowledge graph. As predicted, such drugs can help treat severe COVID-19 cases. Search results show three powerful anti-inflammatories, ruxolitinib, fedratinib, and baricitinib, that can be used to treat the disease to some extent. Deep learning refers to Artificial Neural Networks (ANNs), in which models are designed computationally comprising various processing layers. Researchers have shown that deep learning techniques perform better than traditional machine learning techniques [40]. Fig. 2 displays a summary of AI applications used to mitigate the impact of COVID-19. The applications are powered by artificial Intelligence, including machine learning. The models used in these AI applications make use of real-time data.
Figure 2: Summary of AI applications during COVID-19 pandemic
4.3.1 Drug Discovery and Identification
Like many other deep learning applications in biomedicine and healthcare informatics, these techniques also have much to offer in the current pandemic of COVID-19. As a result, researchers are leveraging various deep learning techniques, from disease diagnostics to discovering already approved drugs and predicting their structure. Zhang et al. [41] presented a Dense, Fully Connected Neural Network-DFCNN to identify protein-ligand interactions of COVID-19. In this research work, RNA sequencing of the virus obtained from the GISSAID database was translated into protein sequencing to build a 3D protein model through the homology model. 2019-nCov_main_protease structure-based drug screening was the focus of this study, as no molecular or docking dynamics were required to perform virtual screening by DFCNN, enhancing potential drug identification capability. The DFCNN identified potential drugs for 2019-n-CoV (2019-nCoV was a provisional name for SARS-CoV-2). To discover already available drugs that can affect SARS-CoV-2 structure components, including helicase, RNA-dependent RNA polymerase, and proteinase, Beck et al. [42] implemented Molecule Transformer-Drug Target Interaction (MT-DTI) model. The model consumes a 1D array of simplified amino acid sequences and molecular-input line-entry system strings; it consumes less time to apply target proteins. Various antiviral drugs were identified by the MT-DTI model that should be under consideration while developing treatment strategies against COVID-19.
Protein structure provides essential information about how it works and interacts with other proteins, and the protein structure of SARS-CoV-2 can provide valuable insights. However, since medical experiments to determine SARS-CoV-2 structure can take months or longer, scientists have had to look for other options. For the same purpose, computational methods have been developed by researchers. When structures of similar proteins have already been discovered through experiments, template modeling-based algorithms can accurately predict protein structure. For example, scientists from DeepMind used AlphaFold, a deep learning-based system, to indicate the SARS-CoV-2 protein structure. AlphaFold focuses on free modeling, which accurately predicts protein structure even when information about similar proteins’ structures is unavailable. It was confirmed by experimental results that AlphaFold predicted the design of the SARS-CoV-2 spike protein shared in the protein Data Bank. Hassantabar et al. [43] developed a deep learning framework for widespread testing to contain the disease. The model collects data from wearable medical devices and some easy-to-answer questions in a questionnaire. During testing, it obtained the highest accuracy of 98.1 percent.
4.3.2 Disease Detection and Diagnosis
Xu et al. [16] presented research to differentiate between healthy cases of Influenza-A viral pneumonia and COVID-19 pneumonia through deep learning techniques using image datasets of pulmonary CT scans. For evaluation, two convolutional neural networks for classification were used. The first was the relatively traditional ResNet-based neural network enhanced to improve accuracy by concatenating the location-attention mechanism in the full-connection layer. Six hundred and eighteen CT samples were classified, including 175 healthy cases, 224 Influenza viral pneumonia, and 219 COVID-19 points. Experimental results exhibit established deep learning models effectively screened COVID-19 cases. Gozes et al. [44] developed an artificial intelligence-based tool to analyze CT images to detect, quantify, and track COVID–19. The presented system implements robust 2D and 3D deep learning models. In addition, various retrospective experiments were conducted that indicated promising results to evaluate the performance of the developed system. Wang et al. [45] proposed a deep learning method to provide a clinical diagnosis by extracting COVID-19 graphical features to save time compared to pathogenic tests. A dataset of those patients having COVID-19 and having typical pneumonia in the past was used. The algorithm was established by modifying the Inception transfer-learning model. The modified algorithm provided 89.5% accuracy and 0.87 sensitivity.
To design drug-like incubators for the current disease, Zhavorronkov et al. [46] used a generative chemistry pipeline as a proprietary for Insilico Medicine. After 2 days, researchers started generating it. Already validated productive chemistry approaches were used, including ligand-based generation, homology modeling-based generation, and crystal-derived packed-based generator. For each input data type, the generative pipeline was launched three times, and, to analyze further, the highest-ranking structures were selected. The analysis results indicate that molecules having the same core did not have similarity values above 0.7. Iktos, specializing in drug design using artificial Intelligence, and SRI International, a research center, started collaborating to discover and design antiviral therapies for COVID-19 on 04 March 2020. Iktos use deep generative models to create novel virtual molecules with a novel drug’s required characteristics. These generative models will be used with SynFiniTM, a synthetic chemistry system designed by SRI International, to treat viruses such as influenza and COVID-19. In Rahimzadeh et al. [47] study for detecting COVID-19, a training methodology incorporates two Deep Convolutional Neural Network (DCNN) architectures, namely Xception and the ResNet50V2, delivered an accuracy of over 99 percent from 11,302 images. In addition, it produced an overall accuracy of over 91 percent for all classes.
Similarly, a framework for COVID-19 detection was recently proposed that used a Deep Convolutional Neural Network to extract features from a dataset of 4356 CT images [48]. The model performed exceptionally well, yielding an accuracy of 96 percent. To further check the robustness of the model, computed tomography (CT) exams of Community-Acquired Pneumonia (CAP) and non-pneumonia abnormalities were added, and it delivered a diagnostic accuracy of 95 percent. Another deep learning method was developed by Yang et al. [49] that also analyzed the CT scans of COVID-19 patients and predicted whether a patient was affected. The model achieved an F1 of 0.90 and an AUC of 0.98 percent.
4.3.3 Disease Outbreak Prediction
To predict disease outbreak time, Fong et al. [50] proposed a methodology that uses three methods proposed in the past: (1) augmentation of existing data, (2) picking the best forecasting model among several models by using a panel selection, and (3) fine-tune parameters of a forecasting algorithm to obtain highest possible accuracy. Using this methodology, an experiment was conducted based on recent COVID-19 data. In addition, a polynomial neural network with corrective feedback (PNN+cf) was used to construct an optimized forecasting model. The experimental results indicate that the proposed methodology and PNN+cf provided acceptable forecasts. An online real-time Kalman filter algorithm was implemented to predict the spread of COVID-19 [51]. A Kalman filter algorithm offers estimates of unknown variables using measurements observed over time. There are two stages of the said algorithm, (1) propagation and (2) correlation. The results showed that more cases recovered than those confirmed to have the disease. Due to the limited availability of medical resources, hospitals will not monitor or care for all patients with severe illnesses. Therefore, it becomes essential for them to predict the recovery or mortality rate of the patients to use the medical resources more efficiently. Al-Najjar et al. [52] collected the patients’ data from hospitals in South Korea and used a neural network classifier to predict their recovery and mortality rate. The algorithm yields an overall testing accuracy of 99.5 percent and 93.8 percent for death and recovery rates. Gemmar et al. [53] also employed a fuzzy classifier to predict the mortality rate of coronavirus patients.
4.3.4 Machine Learning Tracking Methods
Abd-Alrazaq et al. [54] studied the applications of AI and non-AI systems in the pandemic and concluded that AI systems reduce the patients’ treatment time and catalyze disease prediction. In addition, AI systems can assist in tracing COVID-19 cases and effectively control the spread if the proper steps and measures are taken. One area of concern for handling many patients is the need for more facilities, such as ventilators. In these scenarios, public health institutions must make difficult decisions regarding which patients should receive such treatment. Albahri et al. [55] employed an AI-driven decision-analysis technique that prioritizes patients with certain health conditions. The method employs a collection of data that also includes laboratory tests of the patients. The machine learning models LSTMs, GANs, and Extreme Learning Machine (ELM) methods were implemented to accelerate the patient diagnostic process and treatment [56]. A map-based trajectory design was developed by [57], which used artificial Intelligence to provide safety from radio waves or infectious viruses. Likewise, a deep learning algorithm coupled with state-of-the-art machine learning methods is adopted in [58] that helps monitor the patients and offers augmented curation. In [59], Zhu et al. also proposed a mortality prediction algorithm. He used a deep neural network that takes various clinical variables of the COVID-19 disease as input and generates a risk stratification score of the patients. Table 2a summarizes machine learning tracking methods.
Several researchers used machine learning techniques to track infected patients. As per the table above, almost all the researchers have used data visualization. However, the method of identifying and monitoring an infected person is used by [36]. Yet another fantastic technique employed by [34] is of immense importance for the containment of COVID-19. Almost all the researchers make use of graph-based analytics techniques. However, the neural network-based decisions course is calculated using deep learning algorithms. A more precise and accurate system can be designed by combining the features which various scholars identify in different research.
One of the most important factors discussed in Table 2b above is detecting the disease with the help of machine learning tools. All the algorithms described by various researchers worldwide tend to use image processing to identify and compare the scans of healthy and infected patients. Different scholars used various Deep-Learning algorithms to achieve good results [60]. Deep-Learning algorithms were beneficial in this case. However, using neural network-based deep convolutional theorems was more profound and provided good results.
The three steps for using machine learning in the containment of COVID-19 disease are identification, comparison, and prediction [61]. The predictive algorithms for the recovery and mortality prediction of the disease are an essential part of this studyas shown in Table 2c. The deep learning prediction and recovery model provided 99.5% accuracy and are one of the most effective algorithms that can be used for prediction.
Different intelligent tools have been used in various fields to help humankind. During the COVID-19 pandemic, it has also been used in multiple ways. A few of them are discussed below.
A passenger infected with SARS-CoV-2 boarded a cruise ship named Diamond Princess and infected at least 712, resulting in seven deaths. A Japanese expert indicated that disease spread on the cruise ship through food trays passed by the infected crew. If robots were used for this purpose, there might be a chance that passengers would have a different story to tell [62]. Medical workers are trying to contain the pandemic through multiple technologies, including telemedicine and the deployment of robots. Authorities monitored lockdown campaigns in Spain and China using drones [63]. They disinfected an epidemic hotspot in South Korea named Daegu. During the height of the pandemic in February, a Japanese company called Terra Drone used drones to transport quarantine material and medical samples in Xinchang, China [64]. Next, we discuss the IoT and robotics techniques shown in Fig. 3. These techniques are also a subset of Artificial Intelligence. The technical model created in these studies is well qualified to provide a satisfactory solution for COVID-19 containment.
Figure 3: Illustration of how some intelligent devices helped during the COVID-19 pandemic
In March 2020, robots were deployed as medical staff for the newly opened hospitals in Wuhan, China. These medical facilities were a joint project of CloudMinds, China Mobile, and Wuhan Wuchang Hospital. This hospital aimed to relieve healthcare workers who had become exhausted because of continuous work. Robots and various other IoT devices carry out all medical services. The medical staff was alerted for feverish patients upon entering the hospital, made possible by connected 5G thermometers. Vital signs of patients, including oxygen levels, heart rate, and temperature, were monitored by CloudMind’s AI platform synced to intelligent rings and bracelets worn by them. The medical staff was also equipped with smart devices for early signs of disease if they became infected [65]. Founder and CEO of CloudMinds Bill Huang stated that “quarantined patients were helped reduce boredom by entertainment, interaction and information provided by robots such as Cloud Ginger. In addition, these robots reduced in-person contact of doctors and other medical personnel with patients”. According to CloudMinds, the hospital in discussion and several other hospitals like this are currently on standby if the disease resurges.
A Denmark-based company, UVD Robotics, has deployed hundreds of robots to fight against the pandemic [66]. Disinfection robots were dispatched in Wuhan, Veneto, and Rome. Powerful ultraviolet rays from these machines can tear apart strands of virus DNA after completing their tasks in their environment, including patient rooms and hospital corridors. Lidar guided them to roam autonomously, shining a 360-degree UV-C light emitting from the mast. UVD Robotics spokesperson stated, “UVD robots have proven their ability to kill viruses like MER-CoV and mouse hepatitis virus (MHV-A59), which means they can be effective against SARS-CoV-2 as well”. Fig. 3 shows some applications of IoT and Robotics during the pandemic. On the other hand, Xenex Disinfection Services, a healthcare services company based in Texas, also provides robots that can zap viruses to Houston, Italy, and East Asia. These robots disinfect the area through ultraviolet light [67].
4.4.2 Smart Thermometers and IoT
Since the start of the disease, another technology has experienced demand, named telemedicine. Medical specialists from George Washington University Hospital in Washington and Rush University Medical Center in Chicago used them to detect possible COVID-19 patients [68].
Questionnaires are being deployed by Zipnosis, a Minneapolis-based company, so that doctors can identify whether a patient is suffering from COVID-19 or other respiratory diseases. According to company officials, assessing a patient takes less than one and a half minutes [68]. For early detection of COVID-19, several IoT-enabled thermometers have been employed to monitor body temperatures. These easy-to-use devices take accurate measurements, be worn under clothes, and are available in various forms such as patch, touch, and radiometric [6]. Moreover, capturing body temperatures via infrared thermometers will increase the risk of infection as the health workers get close to the patients. So, it is strongly advised to use these IoT-enabled thermometers [69]. Kinsa’s smart thermometers are now commonly used in households in the United States. Based on the temperature readings from the people, the manufacturer can now predict the Covid-19 infected areas using its network of IoT-enabled smart thermometers [70].
To make the diagnostic process quicker and contain the virus spread, it is necessary to find the infected people as early as possible [71]. Therefore, Unmanned Ariel Vehicles (UAVs) are being deployed in many countries to scan out the infected people and zones that are hard to access. These drones are equipped with thermal imaging technology that captures people’s body temperatures and heart rates and can detect sneezing and coughing symptoms. One such example is “Pandemic-Drone,” designed and developed by Draganfly Inc, a Company in Canada [71]. As schools and workplaces reopen, vehicles have started resurfacing on the highways, and people have returned to their daily routines, the coronavirus pandemic is transitioning to a new level. Therefore, everybody must understand the necessity of maintaining social distancing to prevent the virus from spreading. So, autonomous systems can be deployed in such scenarios. In Singapore, four-legged robots, namely Spots [72], are being used to monitor social distancing. These remotely controlled robots can also send the data to a web application for further surveillance. The hybrid model presented by [73] overcomes the disadvantages of the logistic model’s inability to predict the number of confirmed diagnoses and the drawbacks of too many tuning parameters of the SEIR (Susceptible, Exposed, Infectious, Recovered) model for the COVID-19 trend. Reference [74] suggested an exciting method for identifying and helping the patient’s condition based on the replies using natural language processing using the iOS applications.
4.4.4 IoT and Robotics Tools Summary
Table 3 summarizes the significant studies that use an AI tool to contain COVID-19. It shows that Drones, Thermometers, and Robots are well-used techniques in the domain.
Advances in healthcare informatics have brought massive datasets of different types and various subfields of Artificial Intelligence, including machine learning and deep learning. Machine learning and deep learning techniques have been implemented in many fields and have solved previously nearly impossible or very time-consuming problems. Reference [75] proposed InfusedHeart, which was capable to diagnose cardiovascular diseases. Several techniques of this type have also been implemented in the healthcare sector providing efficient solutions such as disease identification and diagnosis, drug discovery and development, medical imaging diagnosis, personalized treatments, and repurposing already approved medicine for a new disease. A decentralized, efficient, and privacy-enhanced federated edge learning for healthcare cyber physical systems was proposed by [76] which was able to use DEEP-FEL for learning model from the healthcare data. From the beginning of COVID-19, these techniques had shown their potential, as BlueDot showed when it flagged the risk of COVID-19 days before authorities took notice. Other applications are critical case recognition, tracking it, repurposing drugs that have been clinically tested and approved, predicting SARS-CoV-2 protein structure, predicting disease outbreak time, predicting disease spread, diagnosing disease through CT images, and discovering and designing antiviral therapies. The rise of Big Data Analytics has also played its part in the current situation.
Coronavirus symptoms were revealed by analysis of RNA-sequencing of a patient admitted to a hospital. Taiwan has prevented the spread of disease by harnessing its abilities. Various online platforms have used disease datasets to visualize suspected and confirmed COVID–19 cases and statistics of the disease on any given day since its start.
Machine learning techniques were used in various ways to predict mortality. Medicines are required for those who have already been diagnosed with the disease, but drug development is time-consuming and requires conducting medical trials. A knowledge graph provided by BenevolentAI was used to discover already approved medicine that can help treat COVID-19 patients in some way. Deep learning techniques have also been used to help with this pandemic, such as identifying protein-protein interactions, discovering approved drugs, and being effective against the new virus. AlphaFold predicted protein structure using a deep learning-based system. Techniques are proposed to predict outbreaks and the spread of disease. Despite machine learning and deep learning algorithms available to analyze the datasets, a significant challenge, which was phased in, is the availability of the datasets from various parts of the world. It is not very easy to collect information despite the large datasets available on free global platforms.
Deep learning computer vision techniques were used to detect and diagnose COVID-19. Various robots and drones are being deployed in the frontline to fight the virus and disease physically, as they were used to disinfect contaminated areas, help patients, and transport medical samples and quarantine materials, scanning potential patients to find out if they were infected or not. While the WHO promotes examining many suspects, many countries could not comply due to a shortage of resources. Here, one alternative could be utilizing different radiographic imaging with the help of AI to support the detection of COVID-19 by providing a fast option and controlling the outbreak. However, it is not objective or accurate to detect COVID-19 from other diseases only with human eyes. Yet it is challenging to analyze the inputs for the computer vision techniques to identify and diagnose COVID-19 based on various image processing-based systems. It is probably the lack of applications or procedures due to limited training data from multiple parts of the world. The machine learning algorithms for computer vision can work well with the help of datasets for training the model. But due to the unavailability of these resources, the training model cannot support the entire system and predict 100% efficiency. There is always scope for improvement in the available datasets. None of the algorithms for computer vision provides a cent percent accuracy.
In comparison, deep learning and other technologies can give specific and reliable results by digitizing and standardizing information. Although Big Data Analytics and AI have numerous applications and have proven to be very effective while countering the COVID-19 disease, many areas need to be explored further. For instance, analyzing data from multiple and disjointed sources in Big Data Analytics remains a big challenge. Combining multiple pieces of data from different sources is time-consuming and may result in inaccurate insights and analysis. Likewise, unreliable data, such as fake social media posts about COVID-19, is a big problem and can significantly harm data analytics. AI-based systems require a large amount of data for accurate prediction, while deep learning algorithms are incredibly data-hungry. Therefore, to solve this problem, the community of researchers can work on a common platform where they can easily share or access the data.
Several diseases remain undiscovered due to unavailability of clinical data. Computational paradigms have been developed during the past two decades. They can prove to be helpful for drug discovery, infection prediction, disease diagnosis and many more factors. Several research have developed some models which contributes a lot to the medicinal healthcare units. The family of coronaviruses has a long-standing history of more than a half-century and has hit humanity in different forms claiming many lives each time. The current coronavirus disease, named COVID-19, has taken over four million lives, and numbers are still rising with each passing day. Previously, medical experts and researchers used traditional techniques to fight against such outbreaks, which were ineffective or time-consuming. This time researchers did not approach COVID-19 in conventional ways. Medical data and computational methods, including Machine Learning and Artificial Intelligence, have experienced a surge, leading to many healthcare advancements. It is now less time-consuming to diagnose diseases, treat them, develop drugs, repurpose already approved drugs for rare or new conditions, and predict the extent of a particular disease. Scientists are using these techniques to tackle the current pandemic. However, many measures could be taken to prevent this pandemic from claiming thousands of lives. If results from BlueDot had been taken seriously at the time, the outcomes of the current pandemic could have been very different. In this paper, we conduct an in-depth analysis of utilizing various techniques such as deep learning, the Internet of Things, and robotics to demonstrate their effectiveness in providing support in detecting, measuring, and tracking disease progression. Consequently, these technologies can provide fast and accurate analyses on different datasets, thus helping clinicians detect and monitor the disease more effectively.
In this article the authors look forward to identifying various containment techniques for COVID-19 with the help of Deep Learning algorithms. The use of robotics to identify patients having an infection is also one of the critical factors which needs to be discussed in the later reviews of the manuscript. IoT devices in healthcare are becoming prominent and very impressive recently. The recording of biometric features like blood pressure, oxygen level, heartbeat, diabetes check, and various other features of a human can be done with the help of such devices. The future enhancement for this research includes the containment of IoT along with robotics and deep learning algorithms for the containment of COVID-19. Despite the various proposed models and analysis of data sets, there is a very strong scope for future research in the field of clinical healthcare. The paper summarizes several articles and a systematic review for the existing models to achieve the sophisticated use of technology. The authors also believe that there is a wide scope for more such technical adaption to achieve quality clinical healthcare with the use of the latest computational paradigms.
Funding Statement: The authors would like to thank the Deanship of Scientific Research at Shaqra University for supporting this work.
Conflicts of Interest: The authors declare that they have no conflicts of interest to report regarding the present study.
References
1. Y. Bai, L. Yao, T. Wei, F. Tian and D. Y. Jin, “Presumed Asymptomatic carrier transmission of COVID-19,” JAMA, vol. 323, no. 14, pp. 1406–1407, 2020. [Google Scholar] [PubMed]
2. J. T. Wu, K. Leung, M. Bushman, N. Kishore, R. Niehus et al., “Estimating clinical severity of COVID-19 from the transmission dynamics in Wuhan, China,” Nature Medicine, vol. 26, no. 4, pp. 506–510, 2020. [Google Scholar] [PubMed]
3. P. Galetsi, K. Katsaliaki and S. Kumar, “Big data analytics in Health Sector: Theoretical framework, techniques and prospects,” Interntional Journal of Information Management, vol. 50, no. 1, pp. 206–216, 2020. [Google Scholar]
4. J. Vamathevan, D. Clark, P. Czodrowski, I. Dunham, E. Ferran et al., “Applications of machine learning in drug discovery and development,” Nature Reviews Drug Discovery, vol. 18, no. 6, pp. 463–477, 2019. [Google Scholar] [PubMed]
5. A. Esteva, A. Robicquet, B. Ramsundar, V. Kuleshov and M. DePristo, “A guide to deep learning in Healthcare,” Nature Medicine, vol. 25, no. 1, pp. 24–29, 2019. [Google Scholar] [PubMed]
6. T. Tamura, M. Huang and T. Togawa, “Current developments in wearable thermometers,” Advanced Biomedical Engineering, vol. 7, pp. 88–99, 2018. [Google Scholar]
7. N. Ahmad, R. P. George and R. Jahan, “Emerging trends in IoT for categorized health care,” in 2nd Int. Conf. on Intelligent Computing, Instrumentation and Control Technologies (ICICICT), Kannur, India, vol. 1, pp. 1438–1441, 2019. [Google Scholar]
8. Y. Yang, W. Wang, Z. Yin, R. Xu, X. Zhou et al., “Mixed game-based AoI optimization for combating COVID-19 with AI bots,” IEEE Journal on Selected Areas in Communications, vol. 40, no. 11, pp. 3122–3138, 2022. [Google Scholar]
9. N. A. Khan and J. Albatein, “COVIBOT-An intelligent WhatsApp based advising bot for COVID-19,” in Int. Conf. on Computational Intelligence and Knowledge Economy (ICCIKE), Amity University Dubai, UAE, pp. 1–5, 2021. [Google Scholar]
10. J. L. Sarkar, V. Ramasamy, A. Majumder, B. Pati, B. Panigrahi et al., “I-health: SDN-based fog architecture for IIoT applications in healthcare,” IEEE/ACM Transactions on Computational Biology and Bioinformatics, vol. 19, pp. 1–8, 2022. [Google Scholar]
11. L. Zhang, J. Wang, W. Wang, Z. Jin, Y. Su et al., “Smart contract vulnerability detection combined with multi-objective detection,” Computer Networks, vol. 217, pp. 109289, 2022. [Google Scholar]
12. A. L. Lau, I. Chi, R. A. Cummins, T. M. Lee, K. L. Chou et al., “The SARS (Severe Acute Respiratory Syndrome) pandemic in Hong Kong: Effects on the subjective wellbeing of elderly and younger people,” Aging and Mental Health, vol. 12, no. 6, pp. 746–760, 2008. [Google Scholar] [PubMed]
13. E. De Wit, N. Van Doremalen, D. Falzarano and V. J. Munster, “SARS and MERS: Recent insights into emerging coronaviruses,” Nature Reviews Microbiology, vol. 14, no. 8, pp. 523–534, 2016. [Google Scholar] [PubMed]
14. H. A. Rothan and S. N. Byrareddy, “The epidemiology and pathogenesis of coronavirus disease (COVID-19) outbreak,” Journal of Autoimmunity, vol. 109, pp. 102433, 2020. [Google Scholar] [PubMed]
15. Q. Li, X. Guan, P. Wu, X. Wang, L. Zhou et al., “Early transmission dynamics in Wuhan, China, of novel coronavirus-infected pneumonia,” New England Journal of Medicine, vol. 382, pp. 1199–1207, 2020. [Google Scholar] [PubMed]
16. X. Xu, X. Jiang, C. Ma, P. Du and X. Li, “A deep learning system to screen novel coronavirus disease 2019 pneumonia,” Engineering, vol. 6, no. 10, pp. 1122–1129, 2020. [Google Scholar] [PubMed]
17. Z. Wu and J. McGoogan, “Characteristics and important lessons from the coronavirus disease 2019 (COVID-19) outbreak in China: Summary of a report of 72 314 cases from the chinese center for disease control and prevention,” JAMA, vol. 323, no. 13, pp. 1239–1242, 2020. [Google Scholar] [PubMed]
18. D. Wang, B. Hu, C. Hu, F. Zhu, X. Liu et al., “Clinical characteristics of 138 hospitalized patients with 2019 novel coronavirus-infected pneumonia in Wuhan, China,” JAMA, vol. 323, no. 11, pp. 1061–1069, 2019. [Google Scholar]
19. F. Pan, T. Ye, P. Sun, S. Gui, B. Liang et al., “Time course of lung changes on chest CT during recovery from 2019 novel coronavirus (COVID-19) pneumonia,” Radiology, vol. 295, no. 3, pp. 715–721, 2020. [Google Scholar] [PubMed]
20. A. Booth, “Systematic reviews of health information services and systems,” Health Information and Libraries Journal, vol. 18, pp. 60–63, 2001. [Google Scholar]
21. F. Wu, S. Zhao, B. Yu, Y. M. Chen, W. Wang et al., “A new coronavirus associated with human respiratory disease in China,” Nature, vol. 579, no. 7798, pp. 265–269, 2020. [Google Scholar] [PubMed]
22. A. Alimadadi, S. Aryal, I. Manandhar, P. B. Munroe, B. Joe et al., “Artificial intelligence and machine learning to fight COVID-19,” Physiological Genomics, vol. 52, no. 4, pp. 200–202, 2020. [Google Scholar] [PubMed]
23. G. Divya Zion and B. K. Tripathy, “Comparative analysis of tools for big data visualization and challenges,” in Data Visualization: Trends and Challenges Toward Multidisciplinary Perception, Singapore: Springer, pp. 33–52, 2020. [Google Scholar]
24. B. Furht, F. Villanustre, J. Shaw, F. Villanustre, B. Furht et al., “Modeling Ebola spread and using HPCC/KEL system,” in Big Data Technologies and Applications, Cham: Springer, pp. 347–385, 2016. [Google Scholar]
25. F. Villanustre, A. Chala, R. Dev, L. Xu and B. Furht, “Modeling and tracking Covid-19 cases using Big Data analytics on HPCC system platform,” Journal of Big Data, vol. 8, no. 1, pp. 1–24, 2021. [Google Scholar]
26. A. Kunjir, J. Shah, N. Singh and T. Wadiwala, “Big data analytics and visualization for hospital recommendation using HCAHPS standardized patient survey,” IJ Information Technology and Computer Science, vol. 3, no. 3, pp. 1–9, 2019. [Google Scholar]
27. L. Mica, C. Niggli, P. Bak, A. Yaeli, M. McClain et al., “Development of a visual analytics tool for polytrauma patients: Proof of concept for a new assessment tool using a multiple layer Sankey diagram in a single-center database,” World Journal of Surgery, vol. 44, pp. 764–772, 2020. [Google Scholar] [PubMed]
28. J. P. Costa, M. Grobelnik, F. Fuart, L. Stopar, G. Epelde et al., “Meaningful big data integration for a global COVID-19 strategy,” IEEE Computational Intelligence Magazine, vol. 15, no. 4, pp. 51–61, 2020. [Google Scholar]
29. D. Souther, A. Samanta, M. Jedruch, M. Tschiegg and S. Barglowski, “Graph visualization by organizing connections in collapsible hierarchical graphs,” Technical Disclosure Commons, vol. 2996, pp. 1–8, 2020. [Google Scholar]
30. R. Alguliyev and F. Yusifov, “Graph modelling for tracking the COVID-19 pandemic spread,” Infectious Disease Modelling, vol. 6, pp. 112–122, 2021. [Google Scholar] [PubMed]
31. L. Qin, Q. Sun, Y. Wang, K. F. Wu, M. Chen et al., “Prediction of number of cases of 2019 novel coronavirus (COVID-19) using social media search index,” International Journal of Environmental Research and Public Health, vol. 17, no. 7, pp. 2365, 2020. [Google Scholar] [PubMed]
32. Z. Li, X. Li, D. Porter, J. Zhang, Y. Jiang et al., “Monitoring the spatial spread of COVID-19 and effectiveness of control measures through human movement data: Proposal for a predictive model using big data analytics,” JMIR Research Protocols, vol. 9, no. 12, pp. e24432, 2020. [Google Scholar] [PubMed]
33. H. Cheng, S. Li and C. Yang, “Initial rapid and proactive response for the COVID-19 outbreak—Taiwan’s experience,” Journal of the Formosan Medical Association, vol. 119, no. 4, pp. 771, 2020. [Google Scholar] [PubMed]
34. J. Kumar Das, G. Tradigo, P. Veltri, H. Guzzi and S. Roy, “Data science in unveiling COVID-19 pathogenesis and diagnosis: Evolutionary origin to drug repurposing,” Briefings in Bioinformatics, vol. 22, no. 2, pp. 855–872, 2021. [Google Scholar] [PubMed]
35. A. Deutsch, Y. Xu, M. Wu and V. Lee, “Tigergraph: A native MPP graph database,” arXiv preprint arXiv:1901.08248, pp. 1–28, 2019. [Google Scholar]
36. W. Mueller, P. Idziaszek, K. Przybył, D. Wojcieszak, J. Frankowski et al., “Mapping and visualization of complex relational structures in the graph form using the Neo4j graph database,” in Eleventh Int. Conf. on Digital Image Processing (ICDIP 2019), Guangzhaou, China, vol. 11179, pp. 581–587, 2019. [Google Scholar]
37. L. Yan, H. Zhang, Y. Xiao, M. Wang, Y. Guo et al., “Prediction of criticality in patients with severe COVID-19 infection using three clinical features: A machine learning-based prognostic model with clinical data in Wuhan,” MedRxiv, pp. 2020–02, 2020. [Google Scholar]
38. Q. Baker, F. Shatnawi, S. Rawashdeh, M. Al-Smadi and Y. Jararweh, “Detecting epidemic diseases using sentiment analysis of arabic tweets,” Journal of Universal Computer Science, vol. 26, no. 1, pp. 50–70, 2020. [Google Scholar]
39. P. Richardson, I. Griffin, C. Tucker, D. Smith, O. Oechsle et al., “Baricitinib as potential treatment for 2019-nCoV acute respiratory disease,” Lancet, vol. 395, no. 10223, pp. e30, 2020. [Google Scholar] [PubMed]
40. J. Stebbing, A. Phelan, I. Griffin, C. Tucker, O. Oechsle et al., “COVID-19: Combining antiviral and anti-inflammatory treatments,” The Lancet Infectious Diseases, vol. 20, no. 4, pp. 400–402, 2020. [Google Scholar] [PubMed]
41. H. Zhang, K. Saravanan, Y. Yang, M. Hossain, J. Li et al., “Deep learning based drug screening for novel coronavirus 2019-nCov,” Interdisciplinary Sciences: Computational Life Sciences, vol. 12, pp. 368–376, 2019. [Google Scholar]
42. B. Beck, B. Shin, Y. Choi, S. Park and K. Kang, “Predicting commercially available antiviral drugs that may act on the novel coronavirus (SARS-CoV-2) through a drug-target interaction deep learning model,” Computational and Structural Biotechnology Journal, vol. 18, pp. 784–790, 2020. [Google Scholar] [PubMed]
43. S. Hassantabar, N. Stefano, V. Ghanakota, A. Ferrari, G. Nicola et al., “CovidDeep: SARS-CoV-2/COVID-19 test based on wearable medical sensors and efficient neural networks,” IEEE Transactions on Consumer Electronics, vol. 67, no. 4, pp. 244–256, 2021. [Google Scholar]
44. O. Gozes, M. Frid-Adar, H. Greenspan, P. Browning, H. Zhang et al., “Rapid AI development cycle for the coronavirus (covid-19) pandemic: Initial results for automated detection & patient monitoring using deep learning ct image analysis,” arXiv preprint, arXiv:2003.05037, pp. 1–22, 2003. [Google Scholar]
45. S. Wang, B. Kang, J. Ma, X. Zeng, M. Xiao et al., “A deep learning algorithm using CT images to screen for Corona Virus Disease (COVID-19),” European Radiology, vol. 31, no. 8, pp. 6096–6104, 2021. [Google Scholar] [PubMed]
46. A. Zhavoronkov, V. Aladinskiy, A. Zhebrak, B. Zagribelnyy, V. Terentiev et al., “Potential 2019-nCoV 3C-like protease inhibitors designed using generative deep learning approaches,” Theoretical and Computational Chemistry, ChemRxiv, pp. 1–17, 2020. https://doi.org/10.26434/chemrxiv.11829102.v1 [Google Scholar] [CrossRef]
47. M. Rahimzadeh and A. Attar, “A modified deep convolutional neural network for detecting COVID-19 and pneumonia from chest X-ray images based on the concatenation of Xception and ResNet50V2,” Informatics in Medicine Unlocked, vol. 19, pp. 100360, 2020. [Google Scholar] [PubMed]
48. L. Li, L. Qin, Z. Xu, Y. Yin, X. Wang et al., “Using artificial intelligence to detect COVID-19 and community-acquired pneumonia based on pulmonary CT: Evaluation of the diagnostic accuracy,” Radiology, vol. 296, no. 2, pp. e65–71, 2020. [Google Scholar]
49. X. Yang, X. He, J. Zhao, Y. Zhang, S. Zhang et al., “COVID-CT-dataset: A CT scan dataset about COVID-19,” arXiv preprint, arXiv:2003.13865, pp. 1–14, 2003. [Google Scholar]
50. S. Fong, G. Li, N. Dey, R. Crespo and E. Herrera-Viedma, “Finding an accurate early forecasting model from small dataset: A case of 2019-ncov novel coronavirus outbreak,” arXiv preprint, arXiv:2003.10776, pp. 1–9, 2003. [Google Scholar]
51. A. Rausch and T. Schanze, “On the use of an extended Kalman filter for subviral particle tracking,” Proceedings on Automation in Medical Engineering, vol. 1, no. 1, pp. 03, 2020. [Google Scholar]
52. N. Al-Najjar and N. Al-Rousan, “A classifier prediction model to predict the status of Coronavirus COVID-19 patients in South Korea,” European Review for Medical and Pharmacological Sciences, vol. 24, no. 6, pp. 3400–3403, 2020. [Google Scholar] [PubMed]
53. P. Gemmar, “An interpretable mortality prediction model for COVID-19 patients-alternative approach,” MedRxiv, vol. 2, pp. 2020–06, 2020. [Google Scholar]
54. A. Abd-Alrazaq, M. Alajlani, D. Alhuwail, J. Schneider, S. Al-Kuwari et al., “Artificial intelligence in the fight against COVID-19: Scoping review,” Journal of Medical Internet Research, vol. 22, no. 12, pp. e20756, 2020. [Google Scholar] [PubMed]
55. A. S. Albahri, J. R. Al-Obaidi, A. Zaidan, O. S. Albahri, R. A. Hamid et al., “Multi-biological laboratory examination framework for the prioritization of patients with COVID-19 based on integrated AHP and group VIKOR methods,” International Journal of Information Technology & Decision Making, vol. 19, no. 5, pp. 1247–1269, 2020. [Google Scholar]
56. M. Jamshidi, A. Lalbakhsh, J. Talla, Z. Peroutka, F. Hadjilooei et al., “Artificial intelligence and COVID-19: Deep learning approaches for diagnosis and treatment,” IEEE Access, vol. 8, pp. 109581–109595, 2020. [Google Scholar] [PubMed]
57. Z. Han, Y. Yang, W. Wang, L. Zhou, T. Gadekallu et al., “RSSI map-based trajectory design for UGV against malicious radio source: A reinforcement learning approach,” IEEE Transactions on Intelligent Transportation Systems, vol. 24, no. 4, pp. 1–10, 2022. [Google Scholar]
58. T. Wagner, F. Shweta, K. Murugadoss, S. Awasthi, J. Venkatakrishnan et al., “Augmented curation of clinical notes from a massive EHR system reveals symptoms of impending COVID-19 diagnosis,” Elife, vol. 9, pp. e58227, 2020. [Google Scholar] [PubMed]
59. J. S. Zhu, P. Ge, C. Jiang, Y. Zhang, X. Li et al., “Deep-learning artificial intelligence analysis of clinical variables predicts mortality in COVID-19 patients,” Journal of the American College of Emergency Physicians Open, vol. 1, no. 6, pp. 1364–1373, 2020. [Google Scholar] [PubMed]
60. S. Elghamrawy, “An h 2 o’s deep learning-inspired model based on big data analytics for coronavirus disease (covid-19) diagnosis,” in Big Data Analytics and Artificial Intelligence Against COVID-19: Innovation Vision and Approach, Cham: Springer, pp. 263–279, 2020. [Google Scholar]
61. C. Anastassopoulou, L. Russo, A. Tsakris and C. Siettos, “Data-based analysis, modelling and forecasting of the COVID-19 outbreak,” PLoS One, vol. 15, no. 3, pp. e0230405, 2020. [Google Scholar] [PubMed]
62. G. Yang, J. Nelson, R. Murphy, H. Choset, H. Christensen et al., “Combating COVID-19—The role of robotics in managing public health and infectious diseases,” Science Robotics, vol. 5, no. 40, pp. eabb5589, 2020. [Google Scholar] [PubMed]
63. B. Skorup and C. Haaland, “How drones can help fight the coronavirus,” Mercatus Center Research Paper Series, Special Edition Policy Brief, pp. 1–4, 2020. [Google Scholar]
64. T. Amukele, “The economics of medical drones,” The Lancet Global Health, vol. 8, no. 1, pp. e22, 2020. [Google Scholar] [PubMed]
65. W. Naudé, “Artificial intelligence against COVID-19: An early review,” Institute for the Study of Labor, pp. 1–17, 2020. [Google Scholar]
66. E. Yetis, A. Gunaldi, M. Atasoy and L. Celik, “Suplement 3,” Annals of Clinical and Analytical Medicine, vol. 12, no. 3, pp. S250–253, 2021. [Google Scholar]
67. Q. C. Feng and X. Wang, “Design of disinfection robot for livestock breeding,” Procedia Computer Science, vol. 166, pp. 310–314, 2020. [Google Scholar]
68. J. Hollander and B. Carr, “Virtually perfect? Telemedicine for COVID-19,” New England Journal of Medicine, vol. 382, no. 18, pp. 1679–1681, 2020. [Google Scholar] [PubMed]
69. M. Mohammed, N. Hazairin, S. Al-Zubaidi, S. AK, S. Mustapha et al., “Toward a novel design for coronavirus detection and diagnosis system using IoT based drone technology,” International Journal of Psychosocial Rehabilitation, vol. 24, no. 7, pp. 2287–2295, 2020. [Google Scholar]
70. S. D. Chamberlain, I. Singh, C. Ariza, A. Daitch, P. Philips et al., “Real-time detection of COVID-19 epicenters within the United States using a network of smart thermometers,” MedRxiv, vol. 528, pp. 2020–04, 2020. [Google Scholar]
71. A. Haleem, M. Javaid, R. Vaishya and S. Deshmukh, “Areas of academic research with the impact of COVID-19,” The American Journal of Emergency Medicine, vol. 38, no. 7, pp. 1524–1526, 2020. [Google Scholar] [PubMed]
72. T. Cozzens, “Pandemic drones to monitor, detect those with COVID-19—GPS world,” GPS World, vol. 20, 2020. [Google Scholar]
73. S. A. Nawaz, J. Li, U. A. Bhatti, S. U. Bazai, A. Zafar et al., “A hybrid approach to forecast the COVID-19 epidemic trend,” PLoS One, vol. 16, no. 10, pp. e0256971, 2021. [Google Scholar] [PubMed]
74. S. M. Alshahrani and N. A. Khan, “COVID-19 advising application development for Apple devices (iOS),” PeerJ Computer Science, vol. 9, no. 10, pp. e1274, 2023. [Google Scholar] [PubMed]
75. S. Pandya, T. R. Gadekallu, P. K. Reddy, W. Wang and M. Alazab, “InfusedHeart: A novel knowledge-infused learning framework for diagnosis of cardiovascular events,” IEEE Transactions on Computational Social Systems, vol. 9, pp. 1–10, 2022. [Google Scholar]
76. Z. Lian, Q. Yang, W. Wang, Q. Zeng, M. Alazab et al., “DEEP-FEL: Decentralized, efficient and privacy-enhanced federated edge learning for healthcare cyber physical systems,” IEEE Transactions on Network Science and Engineering, vol. 9, no. 5, pp. 3558–3569, 2022. [Google Scholar]
Cite This Article
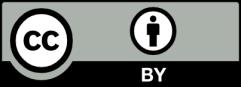
This work is licensed under a Creative Commons Attribution 4.0 International License , which permits unrestricted use, distribution, and reproduction in any medium, provided the original work is properly cited.