Open Access
ARTICLE
Adversarial Attack-Based Robustness Evaluation for Trustworthy AI
Department of Information Security, Hoseo University, Asan 31499, Korea
* Corresponding Author: Taejin Lee. Email:
Computer Systems Science and Engineering 2023, 47(2), 1919-1935. https://doi.org/10.32604/csse.2023.039599
Received 07 February 2023; Accepted 11 May 2023; Issue published 28 July 2023
Abstract
Artificial Intelligence (AI) technology has been extensively researched in various fields, including the field of malware detection. AI models must be trustworthy to introduce AI systems into critical decision-making and resource protection roles. The problem of robustness to adversarial attacks is a significant barrier to trustworthy AI. Although various adversarial attack and defense methods are actively being studied, there is a lack of research on robustness evaluation metrics that serve as standards for determining whether AI models are safe and reliable against adversarial attacks. An AI model’s robustness level cannot be evaluated by traditional evaluation indicators such as accuracy and recall. Additional evaluation indicators are necessary to evaluate the robustness of AI models against adversarial attacks. In this paper, a Sophisticated Adversarial Robustness Score (SARS) is proposed for AI model robustness evaluation. SARS uses various factors in addition to the ratio of perturbated features and the size of perturbation to evaluate robustness accurately in the evaluation process. This evaluation indicator reflects aspects that are difficult to evaluate using traditional evaluation indicators. Moreover, the level of robustness can be evaluated by considering the difficulty of generating adversarial samples through adversarial attacks. This paper proposed using SARS, calculated based on adversarial attacks, to identify data groups with robustness vulnerability and improve robustness through adversarial training. Through SARS, it is possible to evaluate the level of robustness, which can help developers identify areas for improvement. To validate the proposed method, experiments were conducted using a malware dataset. Through adversarial training, it was confirmed that SARS increased by 70.59%, and the recall reduction rate improved by 64.96%. Through SARS, it is possible to evaluate whether an AI model is vulnerable to adversarial attacks and to identify vulnerable data types. In addition, it is expected that improved models can be achieved by improving resistance to adversarial attacks via methods such as adversarial training.Keywords
Cite This Article
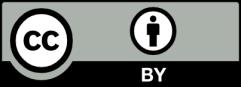
This work is licensed under a Creative Commons Attribution 4.0 International License , which permits unrestricted use, distribution, and reproduction in any medium, provided the original work is properly cited.