Open Access
ARTICLE
An Efficient 3D CNN Framework with Attention Mechanisms for Alzheimer’s Disease Classification
1 Department of Electronics and Communication Engineering, College of Engineering, Trivandrum, 695016, India
2 Department of Computer Science and Engineering, College of Engineering Muttathara, Trivandrum, 695008, India
3 Department of Electronics and Communication, Rajeev Gandhi Institute of Technology, Kottayam, 686501, India
4 Department of Electronics and Communication Engineering, Government Engineering College, Wayanad, 670644, India
* Corresponding Author: Sivakumar Ramachandran. Email:
Computer Systems Science and Engineering 2023, 47(2), 2097-2118. https://doi.org/10.32604/csse.2023.039262
Received 18 January 2023; Accepted 25 April 2023; Issue published 28 July 2023
Abstract
Neurodegeneration is the gradual deterioration and eventual death of brain cells, leading to progressive loss of structure and function of neurons in the brain and nervous system. Neurodegenerative disorders, such as Alzheimer’s, Huntington’s, Parkinson’s, amyotrophic lateral sclerosis, multiple system atrophy, and multiple sclerosis, are characterized by progressive deterioration of brain function, resulting in symptoms such as memory impairment, movement difficulties, and cognitive decline. Early diagnosis of these conditions is crucial to slowing down cell degeneration and reducing the severity of the diseases. Magnetic resonance imaging (MRI) is widely used by neurologists for diagnosing brain abnormalities. The majority of the research in this field focuses on processing the 2D images extracted from the 3D MRI volumetric scans for disease diagnosis. This might result in losing the volumetric information obtained from the whole brain MRI. To address this problem, a novel 3D-CNN architecture with an attention mechanism is proposed to classify whole-brain MRI images for Alzheimer’s disease (AD) detection. The 3D-CNN model uses channel and spatial attention mechanisms to extract relevant features and improve accuracy in identifying brain dysfunctions by focusing on specific regions of the brain. The pipeline takes pre-processed MRI volumetric scans as input, and the 3D-CNN model leverages both channel and spatial attention mechanisms to extract precise feature representations of the input MRI volume for accurate classification. The present study utilizes the publicly available Alzheimer’s disease Neuroimaging Initiative (ADNI) dataset, which has three image classes: Mild Cognitive Impairment (MCI), Cognitive Normal (CN), and AD affected. The proposed approach achieves an overall accuracy of 79% when classifying three classes and an average accuracy of 87% when identifying AD and the other two classes. The findings reveal that 3D-CNN models with an attention mechanism exhibit significantly higher classification performance compared to other models, highlighting the potential of deep learning algorithms to aid in the early detection and prediction of AD.Keywords
Cite This Article
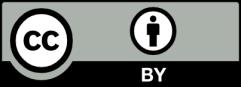
This work is licensed under a Creative Commons Attribution 4.0 International License , which permits unrestricted use, distribution, and reproduction in any medium, provided the original work is properly cited.