Open Access
ARTICLE
Chest Radiographs Based Pneumothorax Detection Using Federated Learning
1 Department of Computer Engineering and Networks, College of Computer and Information Sciences, Jouf University, Sakaka, 72388, Saudi Arabia
2 Department of Computer Science, College of Computing, Khon Kaen University, Khon Kaen, 40002, Thailand
3 School of Telecommunication Engineering, Suranaree University of Technology, Nakhon Ratchasima, 30000, Thailand
4 Department of Computer Science and Software Engineering, International Islamic University, Islamabad, Pakistan
5 Information Systems Department, Faculty of Management, Comenius University in Bratislava, Odbojárov, Bratislava, 440, Slovakia
6 Prince Sattam bin Abdulaziz University, AlKharj, Saudi Arabia
* Corresponding Authors: Ahmad Almadhor. Email: ; Chitapong Wechtaisong. Email:
Computer Systems Science and Engineering 2023, 47(2), 1775-1791. https://doi.org/10.32604/csse.2023.039007
Received 07 January 2023; Accepted 20 March 2023; Issue published 28 July 2023
Abstract
Pneumothorax is a thoracic condition that occurs when a person’s lungs collapse, causing air to enter the pleural cavity, the area close to the lungs and chest wall. The most persistent disease, as well as one that necessitates particular patient care and the privacy of their health records. The radiologists find it challenging to diagnose pneumothorax due to the variations in images. Deep learning-based techniques are commonly employed to solve image categorization and segmentation problems. However, it is challenging to employ it in the medical field due to privacy issues and a lack of data. To address this issue, a federated learning framework based on an Xception neural network model is proposed in this research. The pneumothorax medical image dataset is obtained from the Kaggle repository. Data preprocessing is performed on the used dataset to convert unstructured data into structured information to improve the model’s performance. Min-max normalization technique is used to normalize the data, and the features are extracted from chest X-ray images. Then dataset converts into two windows to make two clients for local model training. Xception neural network model is trained on the dataset individually and aggregates model updates from two clients on the server side. To decrease the over-fitting effect, every client analyses the results three times. Client 1 performed better in round 2 with a 79.0% accuracy, and client 2 performed better in round 2 with a 77.0% accuracy. The experimental result shows the effectiveness of the federated learning-based technique on a deep neural network, reaching a 79.28% accuracy while also providing privacy to the patient’s data.Keywords
Cite This Article
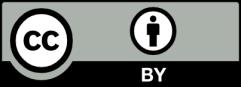
This work is licensed under a Creative Commons Attribution 4.0 International License , which permits unrestricted use, distribution, and reproduction in any medium, provided the original work is properly cited.