Open Access
ARTICLE
Robust Deep Learning Model for Black Fungus Detection Based on Gabor Filter and Transfer Learning
1 Machine Learning and Information Retrieval Department, Faculty of Artificial Intelligence, Kafrelsheikh University, Kafrelsheikh, Egypt
2 Electronics and Communication Engineering Department, Faculty of Engineering Horus University, Damietta, Egypt
3 Information Systems Department, Faculty of Computers and Information, Mansoura University, Mansoura,35516, Egypt
4 Department of ICT Convergence, Soonchunhyang University, Asan, Korea
* Corresponding Author: Yunyoung Nam. Email:
Computer Systems Science and Engineering 2023, 47(2), 1507-1525. https://doi.org/10.32604/csse.2023.037493
Received 06 November 2022; Accepted 13 April 2023; Issue published 28 July 2023
Abstract
Black fungus is a rare and dangerous mycology that usually affects the brain and lungs and could be life-threatening in diabetic cases. Recently, some COVID-19 survivors, especially those with co-morbid diseases, have been susceptible to black fungus. Therefore, recovered COVID-19 patients should seek medical support when they notice mucormycosis symptoms. This paper proposes a novel ensemble deep-learning model that includes three pre-trained models: reset (50), VGG (19), and Inception. Our approach is medically intuitive and efficient compared to the traditional deep learning models. An image dataset was aggregated from various resources and divided into two classes: a black fungus class and a skin infection class. To the best of our knowledge, our study is the first that is concerned with building black fungus detection models based on deep learning algorithms. The proposed approach can significantly improve the performance of the classification task and increase the generalization ability of such a binary classification task. According to the reported results, it has empirically achieved a sensitivity value of 0.9907, a specificity value of 0.9938, a precision value of 0.9938, and a negative predictive value of 0.9907.Keywords
Cite This Article
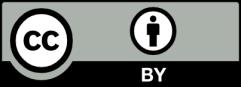