Open Access
ARTICLE
Improved Attentive Recurrent Network for Applied Linguistics-Based Offensive Speech Detection
1 Department of Computer and Self Development, Preparatory Year Deanship, Prince Sattam bin Abdulaziz University, AlKharj, Saudi Arabia
2 Department of Applied Linguistics, College of Languages, Princess Nourah Bint Abdulrahman University, P.O. Box 84428, Riyadh, 11671, Saudi Arabia
3 Department of Computer Sciences, College of Computing and Information System, Umm Al-Qura University, Makkah, Saudi Arabia
4 Department of Information Systems, Faculty of Computing and Information Technology, King Abdulaziz University, Jeddah, Saudi Arabia
5 Department of Digital Media, Faculty of Computers and Information Technology, Future University in Egypt, New Cairo, 11835, Egypt
6 Department of English, College of Science & Humanities, Prince Sattam bin Abdulaziz University, AlKharj, Saudi Arabia
* Corresponding Author: Manar Ahmed Hamza. Email:
Computer Systems Science and Engineering 2023, 47(2), 1691-1707. https://doi.org/10.32604/csse.2023.034798
Received 27 July 2022; Accepted 13 November 2022; Issue published 28 July 2023
Abstract
Applied linguistics is one of the fields in the linguistics domain and deals with the practical applications of the language studies such as speech processing, language teaching, translation and speech therapy. The ever-growing Online Social Networks (OSNs) experience a vital issue to confront, i.e., hate speech. Amongst the OSN-oriented security problems, the usage of offensive language is the most important threat that is prevalently found across the Internet. Based on the group targeted, the offensive language varies in terms of adult content, hate speech, racism, cyberbullying, abuse, trolling and profanity. Amongst these, hate speech is the most intimidating form of using offensive language in which the targeted groups or individuals are intimidated with the intent of creating harm, social chaos or violence. Machine Learning (ML) techniques have recently been applied to recognize hate speech-related content. The current research article introduces a Grasshopper Optimization with an Attentive Recurrent Network for Offensive Speech Detection (GOARN-OSD) model for social media. The GOARN-OSD technique integrates the concepts of DL and metaheuristic algorithms for detecting hate speech. In the presented GOARN-OSD technique, the primary stage involves the data pre-processing and word embedding processes. Then, this study utilizes the Attentive Recurrent Network (ARN) model for hate speech recognition and classification. At last, the Grasshopper Optimization Algorithm (GOA) is exploited as a hyperparameter optimizer to boost the performance of the hate speech recognition process. To depict the promising performance of the proposed GOARN-OSD method, a widespread experimental analysis was conducted. The comparison study outcomes demonstrate the superior performance of the proposed GOARN-OSD model over other state-of-the-art approaches.Keywords
Cite This Article
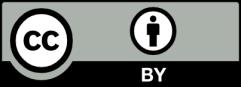
This work is licensed under a Creative Commons Attribution 4.0 International License , which permits unrestricted use, distribution, and reproduction in any medium, provided the original work is properly cited.