Open Access
ARTICLE
Artificial Humming Bird Optimization with Siamese Convolutional Neural Network Based Fruit Classification Model
1 Department of Information Technology, Chaitanya Bharathi Institute of Technology, Hyderabad, Telangana, India
2 Department of Computer Science, College of Computer and Information Sciences, Majmaah University, Al-Majmaah, 11952, Saudi Arabia
3 Department of Electrical Engineering, College of Engineering, Jouf University, Sakaka, Saudi Arabia
4 Department of Computer Science and Engineering, Vignan’s Institute of Information Technology, Visakhapatnam, 530049, India
5 Department of International Affairs and Education, Gangseo University, Seoul, 07661, Korea
6 Department of Information and Communication Engineering and Convergence Engineering for Intelligent Drone, Sejong University, Seoul, 05006, Korea
7 Department of Computer Science and Engineering, Sejong University, Seoul, 05006, Korea
* Corresponding Author: Hyeonjoon Moon. Email:
Computer Systems Science and Engineering 2023, 47(2), 1633-1650. https://doi.org/10.32604/csse.2023.034769
Received 27 July 2022; Accepted 26 October 2022; Issue published 28 July 2023
Abstract
Fruit classification utilizing a deep convolutional neural network (CNN) is the most promising application in personal computer vision (CV). Profound learning-related characterization made it possible to recognize fruits from pictures. But, due to the similarity and complexity, fruit recognition becomes an issue for the stacked fruits on a weighing scale. Recently, Machine Learning (ML) methods have been used in fruit farming and agriculture and brought great convenience to human life. An automated system related to ML could perform the fruit classifier and sorting tasks previously managed by human experts. CNN’s (convolutional neural networks) have attained incredible outcomes in image classifiers in several domains. Considering the success of transfer learning and CNNs in other image classifier issues, this study introduces an Artificial Humming Bird Optimization with Siamese Convolutional Neural Network based Fruit Classification (AMO-SCNNFC) model. In the presented AMO-SCNNFC technique, image preprocessing is performed to enhance the contrast level of the image. In addition, spiral optimization (SPO) with the VGG-16 model is utilized to derive feature vectors. For fruit classification, AHO with end to end SCNN (ESCNN) model is applied to identify different classes of fruits. The performance validation of the AMO-SCNNFC technique is tested using a dataset comprising diverse classes of fruit images. Extensive comparison studies reported improving the AMO-SCNNFC technique over other approaches with higher accuracy of 99.88%.Keywords
Cite This Article
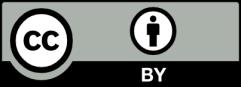
This work is licensed under a Creative Commons Attribution 4.0 International License , which permits unrestricted use, distribution, and reproduction in any medium, provided the original work is properly cited.