Open Access
ARTICLE
Detecting Ethereum Ponzi Schemes Through Opcode Context Analysis and Oversampling-Based AdaBoost Algorithm
1 Faculty of Information Technology, Beijing University of Technology, Beijing, 100124, China
2 Beijing Key Laboratory of Computational Intelligence and Intelligence System, Beijing, 100124, China
* Corresponding Author: Jing Huang. Email:
(This article belongs to the Special Issue: Advances in Mobile Internet Security)
Computer Systems Science and Engineering 2023, 47(1), 1023-1042. https://doi.org/10.32604/csse.2023.039569
Received 06 February 2023; Accepted 13 April 2023; Issue published 26 May 2023
Abstract
Due to the anonymity of blockchain, frequent security incidents and attacks occur through it, among which the Ponzi scheme smart contract is a classic type of fraud resulting in huge economic losses. Machine learning-based methods are believed to be promising for detecting ethereum Ponzi schemes. However, there are still some flaws in current research, e.g., insufficient feature extraction of Ponzi scheme smart contracts, without considering class imbalance. In addition, there is room for improvement in detection precision. Aiming at the above problems, this paper proposes an ethereum Ponzi scheme detection scheme through opcode context analysis and adaptive boosting (AdaBoost) algorithm. Firstly, this paper uses the n-gram algorithm to extract more comprehensive contract opcode features and combine them with contract account features, which helps to improve the feature extraction effect. Meanwhile, adaptive synthetic sampling (ADASYN) is introduced to deal with class imbalanced data, and integrated with the Adaboost classifier. Finally, this paper uses the improved AdaBoost classifier for the identification of Ponzi scheme contracts. Experimentally, this paper tests our model in real-world smart contracts and compares it with representative methods in the aspect of F1-score and precision. Moreover, this article compares and discusses the state of art methods with our method in four aspects: data acquisition, data preprocessing, feature extraction, and classifier design. Both experiment and discussion validate the effectiveness of our model.Keywords
Cite This Article
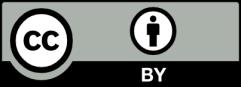