Open Access
ARTICLE
Detecting and Classifying Darknet Traffic Using Deep Network Chains
1 Computer Engineering Department, Umm Al-Qura University, Mecca, Saudi Arabia
2 Smart Lab, Umm Al-Qura University, Mecca, Saudi Arabia
3 Information Systems Department, Umm Al-Qura University, Mecca, Saudi Arabia
4 College of Computer and Information Sciences, King Saud University, Riyadh, Saudi Arabia
* Corresponding Author: Amr Munshi. Email:
(This article belongs to the Special Issue: Intelligent Uni-modal and Multi-modal Agents against Adversarial Cyber Attacks)
Computer Systems Science and Engineering 2023, 47(1), 891-902. https://doi.org/10.32604/csse.2023.039374
Received 25 January 2023; Accepted 12 April 2023; Issue published 26 May 2023
Abstract
The anonymity of the darknet makes it attractive to secure communication lines from censorship. The analysis, monitoring, and categorization of Internet network traffic are essential for detecting darknet traffic that can generate a comprehensive characterization of dangerous users and assist in tracing malicious activities and reducing cybercrime. Furthermore, classifying darknet traffic is essential for real-time applications such as the timely monitoring of malware before attacks occur. This paper presents a two-stage deep network chain for detecting and classifying darknet traffic. In the first stage, anonymized darknet traffic, including VPN and Tor traffic related to hidden services provided by darknets, is detected. In the second stage, traffic related to VPNs and Tor services is classified based on their respective applications. The methodology of this paper was verified on a benchmark dataset containing VPN and Tor traffic. It achieved an accuracy of 96.8% and 94.4% in the detection and classification stages, respectively. Optimization and parameter tuning were performed in both stages to achieve more accurate results, enabling practitioners to combat alleged malicious activities and further detect such activities after outbreaks. In the classification stage, it was observed that the misclassifications were due to the audio and video streaming commonly used in shared real-time protocols. However, in cases where it is desired to distinguish between such activities accurately, the presented deep chain classifier can accommodate additional classifiers. Furthermore, additional classifiers could be added to the chain to categorize specific activities of interest further.Keywords
Cite This Article
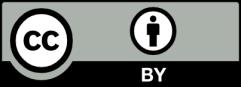