Open Access
ARTICLE
Efficient Explanation and Evaluation Methodology Based on Hybrid Feature Dropout
Department of Information Security, Hoseo University, Asan, 31499, Korea
* Corresponding Author: Taejin Lee. Email:
(This article belongs to the Special Issue: Artificial Intelligence for Cyber Security)
Computer Systems Science and Engineering 2023, 47(1), 471-490. https://doi.org/10.32604/csse.2023.038413
Received 12 December 2022; Accepted 03 March 2023; Issue published 26 May 2023
Abstract
AI-related research is conducted in various ways, but the reliability of AI prediction results is currently insufficient, so expert decisions are indispensable for tasks that require essential decision-making. XAI (eXplainable AI) is studied to improve the reliability of AI. However, each XAI methodology shows different results in the same data set and exact model. This means that XAI results must be given meaning, and a lot of noise value emerges. This paper proposes the HFD (Hybrid Feature Dropout)-based XAI and evaluation methodology. The proposed XAI methodology can mitigate shortcomings, such as incorrect feature weights and impractical feature selection. There are few XAI evaluation methods. This paper proposed four evaluation criteria that can give practical meaning. As a result of verifying with the malware data set (Data Challenge 2019), we confirmed better results than other XAI methodologies in 4 evaluation criteria. Since the efficiency of interpretation is verified with a reasonable XAI evaluation standard, The practicality of the XAI methodology will be improved. In addition, The usefulness of the XAI methodology will be demonstrated to enhance the reliability of AI, and it helps apply AI results to essential tasks that require expert decision-making.Keywords
Cite This Article
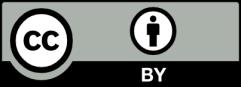
This work is licensed under a Creative Commons Attribution 4.0 International License , which permits unrestricted use, distribution, and reproduction in any medium, provided the original work is properly cited.