Open Access
ARTICLE
Towards Sustainable Agricultural Systems: A Lightweight Deep Learning Model for Plant Disease Detection
1 Department of Software, Sejong University, Seoul, 05006, Korea
2 Department of Convergence Engineering for Intelligent Drone, Sejong University, Seoul, 05006, Korea
3 Department of Electrical Engineering, College of Engineering, Jouf University, Sakaka, Saudi Arabia
* Corresponding Author: Jong Weon Lee. Email:
Computer Systems Science and Engineering 2023, 47(1), 515-536. https://doi.org/10.32604/csse.2023.037992
Received 23 November 2022; Accepted 03 March 2023; Issue published 26 May 2023
Abstract
A country’s economy heavily depends on agricultural development. However, due to several plant diseases, crop growth rate and quality are highly suffered. Accurate identification of these diseases via a manual procedure is very challenging and time-consuming because of the deficiency of domain experts and low-contrast information. Therefore, the agricultural management system is searching for an automatic early disease detection technique. To this end, an efficient and lightweight Deep Learning (DL)-based framework (E-GreenNet) is proposed to overcome these problems and precisely classify the various diseases. In the end-to-end architecture, a MobileNetV3Small model is utilized as a backbone that generates refined, discriminative, and prominent features. Moreover, the proposed model is trained over the PlantVillage (PV), Data Repository of Leaf Images (DRLI), and a new Plant Composite (PC) dataset individually, and later on test samples, its actual performance is evaluated. After extensive experimental analysis, the proposed model obtained 1.00%, 0.96% and 0.99% accuracies on all three included datasets. Moreover, the proposed method achieves better inference speed when compared with other State-Of-The-Art (SOTA) approaches. In addition, a comparative analysis is conducted where the proposed strategy shows tremendous discriminative scores as compared to the various pre-trained models and other Machine Learning (ML) and DL methods.Keywords
Cite This Article
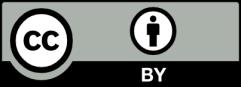
This work is licensed under a Creative Commons Attribution 4.0 International License , which permits unrestricted use, distribution, and reproduction in any medium, provided the original work is properly cited.