Open Access
ARTICLE
Automatic Diagnosis of Polycystic Ovarian Syndrome Using Wrapper Methodology with Deep Learning Techniques
1 Department of Mathematics, Faculty of Science, Mansoura University, Mansoura, 35516, Egypt
2 Department of Computational Mathematics, Science, and Engineering (CMSE), College of Engineering, Michigan State University, East Lansing, MI, 48824, USA
3 Sri Ramachandra Faculty of Engineering & Technology, Sri Ramachandra Institute of Higher Education and Research, Porur, Chennai, 600116, India
4 Department of Information Technology, Panimalar Institute of Technology, Chennai, 600123, India
5 Department of Computer Science and Engineering, School of Engineering, MIT Art, Design and Technology University, Pune, 412201, India
6 Department of Computer Sciences, College of Computer and Information Sciences, Princess Nourahbint Abdulrahman University, P.O. Box 84428, Riyadh, 11671, Saudi Arabia
* Corresponding Author: Doaa Sami Khafaga. Email:
Computer Systems Science and Engineering 2023, 47(1), 239-253. https://doi.org/10.32604/csse.2023.037812
Received 17 November 2022; Accepted 24 February 2023; Issue published 26 May 2023
Abstract
One of the significant health issues affecting women that impacts their fertility and results in serious health concerns is Polycystic ovarian syndrome (PCOS). Consequently, timely screening of polycystic ovarian syndrome can help in the process of recovery. Finding a method to aid doctors in this procedure was crucial due to the difficulties in detecting this condition. This research aimed to determine whether it is possible to optimize the detection of PCOS utilizing Deep Learning algorithms and methodologies. Additionally, feature selection methods that produce the most important subset of features can speed up calculation and enhance the effectiveness of classifiers. In this research, the tri-stage wrapper method is used because it reduces the computation time. The proposed study for the Automatic diagnosis of PCOS contains preprocessing, data normalization, feature selection, and classification. A dataset with 39 characteristics, including metabolism, neuroimaging, hormones, and biochemical information for 541 subjects, was employed in this scenario. To start, this research pre-processed the information. Next for feature selection, a tri-stage wrapper method such as Mutual Information, ReliefF, Chi-Square, and Xvariance is used. Then, various classification methods are tested and trained. Deep learning techniques including convolutional neural network (CNN), multi-layer perceptron (MLP), Recurrent neural network (RNN), and Bi long short-term memory (Bi-LSTM) are utilized for categorization. The experimental finding demonstrates that with effective feature extraction process using tri stage wrapper method + CNN delivers the highest precision (97%), high accuracy (98.67%), and recall (89%) when compared with other machine learning algorithms.Keywords
Cite This Article
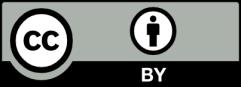
This work is licensed under a Creative Commons Attribution 4.0 International License , which permits unrestricted use, distribution, and reproduction in any medium, provided the original work is properly cited.