Open Access
ARTICLE
Predictive Multimodal Deep Learning-Based Sustainable Renewable and Non-Renewable Energy Utilization
1 Department of Information Systems, College of Business Administration in Hawtat bani Tamim, Prince Sattam bin Abdulaziz University, Howtat Bani Tamim, 16622, Saudi Arabia
2 Department of Biomedical Engineering, College of Engineering, Princess Nourah bint Abdulrahman University, P. O. Box 84428, Riyadh, 11671, Saudi Arabia
3 Department of Information Systems, College of Computer Science, King Khalid University, Abha, Saudi Arabia
4 Department of Computer Sciences, College of Computing and Information System, Umm Al-Qura University, Saudi Arabia
5 Department of Computer Science, Faculty of Computers and Information Technology, Future University in Egypt, New Cairo, 11835, Egypt
6 Department of Computer and Self Development, Preparatory Year Deanship, Prince Sattam bin Abdulaziz University, AlKharj, Saudi Arabia
* Corresponding Author: Abdelwahed Motwakel. Email:
Computer Systems Science and Engineering 2023, 47(1), 1267-1281. https://doi.org/10.32604/csse.2023.037735
Received 15 November 2022; Accepted 02 February 2023; Issue published 26 May 2023
Abstract
Recently, renewable energy (RE) has become popular due to its benefits, such as being inexpensive, low-carbon, ecologically friendly, steady, and reliable. The RE sources are gradually combined with non-renewable energy (NRE) sources into electric grids to satisfy energy demands. Since energy utilization is highly related to national energy policy, energy prediction using artificial intelligence (AI) and deep learning (DL) based models can be employed for energy prediction on RE and NRE power resources. Predicting energy consumption of RE and NRE sources using effective models becomes necessary. With this motivation, this study presents a new multimodal fusion-based predictive tool for energy consumption prediction (MDLFM-ECP) of RE and NRE power sources. Actual data may influence the prediction performance of the results in prediction approaches. The proposed MDLFM-ECP technique involves pre-processing, fusion-based prediction, and hyperparameter optimization. In addition, the MDLFM-ECP technique involves the fusion of four deep learning (DL) models, namely long short-term memory (LSTM), bidirectional LSTM (Bi-LSTM), deep belief network (DBN), and gated recurrent unit (GRU). Moreover, the chaotic cat swarm optimization (CCSO) algorithm is applied to tune the hyperparameters of the DL models. The design of the CCSO algorithm for optimal hyperparameter tuning of the DL models, showing the novelty of the work. A series of simulations took place to validate the superior performance of the proposed method, and the simulation outcome emphasized the improved results of the MDLFM-ECP technique over the recent approaches with minimum overall mean absolute percentage error of 3.58%.Keywords
Cite This Article
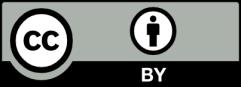