Open Access
ARTICLE
Signal Processing and AI-based Assessment of Rehabilitation Exercises for Diastasis Recti Abdominis
1 Centre for Cyber Physical Systems (CCPS), School of Electronics Engineering, Vellore Institute of Technology, Chennai, 600127, India
2 Faculty of Physiotherapy, Sri Ramachandra Institute of Higher Education and Research, Chennai, 600116, India
3 System Sciences and Industrial Engineering, Binghamton University, Binghamton, 13902-6000, USA
4 School of Electronics Engineering, Vellore Institute of Technology, Chennai, 600127, India
* Corresponding Author: R. Menaka. Email:
Computer Systems Science and Engineering 2023, 47(1), 333-348. https://doi.org/10.32604/csse.2023.037661
Received 12 November 2022; Accepted 09 February 2023; Issue published 26 May 2023
Abstract
Diastasis Recti Abdominis (DRA) is the separation of abdominal recti muscles which occurs in women during their pregnancy and postpartum time. This is because of the stretching of the linea alba, a fibrous connective tissue on the abdominal wall. The Linea Alba is elastic and retracts back after the delivery of the baby. When this tissue gets overstretched, it loses its elasticity and the gap in the abdominals may not be closed leading to DRA. The motive of this research is to analyze the postpartum rehabilitation for signals from Inertial Measurement Unit (IMU) sensors. The conservative treatment for women who are experiencing DRA is given in the form of physiotherapy. These physiotherapy exercises focus on the recti abdominis muscle to bring back the Linea alba together. It will be a difficult process for the physiotherapist to monitor, whether patients did the exercises correctly or not. If the exercises are not correct, they will not be effective in the reduction of inter-recti distance. This research aims to analyze the effectiveness of IMU signals in classifying the correct and incorrect exercises. It was inferred that the IMU signals are effective in classifying the correct and incorrect exercises with an accuracy of 92%.Keywords
Diastasis Recti Abdominis (DRA) is the divarication of the rectus abdominis muscle along the linea alba which occurs during and after pregnancy. This condition is prevalent in postpartum women. The linea alba is a fibrous tissue connecting two parts of the recti muscles on either side of the abdomen. During pregnancy, the linea alba gets stretched and the inter-recti distance (IRD) on the abdominal wall increases. In most women, this separation regresses to normal after pregnancy with reduced IRD. Due to the heterogeneity in the factors used for diagnosis, such as the IRD cut-off used for diagnoses, the methodologies used for diagnosis, and the measuring site in the abdomen, the prevalence of DRA differs across different research works. DRA can exist in women even after many years of pregnancy [1].
DRA occurs when these recti muscles do not regress to normal even after 8 weeks of pregnancy and the linea alba remains overstretched. If the abdominal separation is small, the woman may not experience any difficulty in her life. When the abdominal separation is greater, DRA causes discomfort in women and affects their quality of life [2–5]. Hence women feel difficulty in walking, lifting heavy weights, and performing daily tasks. Women may also experience lumbopelvic pain. It was reported that after 6 months of postpartum nearly 39% of the women are experiencing DRA [6]. DRA is more significant in post-cesarean section (CS) women [7]. In some extreme conditions where the separation does not regress to normal for a long time, there is a chance of developing a hernia. In such cases, abdominal correction is done using surgery.
DRA is diagnosed by palpation of the abdomen. Medical professionals use vernier calipers to measure IRD. They also prefer abdominal ultrasonography of the abdomen region for the diagnosis of DRA [8]. Abdominal core stability exercises such as sit-ups, curl-up, etc. are effective in treating postpartum women with DRA [9]. Abdominal exercises with bracing were effective in reducing the separation of the diastasis recti muscles [10]. The advancement of computer technology in the medical field has highly assisted healthcare professionals in effective measurements and ease of identification of a problem and less time consumption. It is a tedious process for doctors and physiotherapists to track and monitor the movement or exercises done by patients constantly. If the exercises were done incorrectly, the patients could not see any positive results. Hence, an artificial intelligence-based system aids in the identification of the correctness of rehabilitation exercises which can assist patients in their recovery from DRA.
Women after delivery seek medical professionals for concerns including lower abdominal discomfort, lower back pain, or urinary incontinence. Patients would approach the doctor after an upper abdominal bulge [11]. Hence these deformations cause functional and aesthetic disturbances [12]. Women also tend to develop impairments in trunk strength and endurance as an influence of DRA [13]. Diastasis Recti can be determined by medical professionals either through palpation of the abdomen or using ultrasound imaging. Reference [14] The vernier calipers can be used to measure the inter recti distance and when the gap is measured more than 2.7 cm in the middle region of the abdomen the condition is diagnosed as DRA. Diastasis recti can be either treated conservatively with physiotherapy or surgically. The reduction in IRD requires the activation of abdominal muscles. This improves the strength of the core abdominal muscle [15]. It has been discussed that Kinesio taping of abdominal walls can minimalize the effects of DRA [16]. Various exercises can be suggested by the physiotherapist to bind the gap between the recti muscles. For those women who do not show any improvements in inter recti distance, surgery is required for the correction of the abdomen. As the need for recovery in support of functional indications of DRA has increased, surgical reconstruction has gained acceptance in recent times [17]. Even with favorable outcomes of surgical reconstruction, improvement in abdominal core function lacks control. Also, the surgery tends to cause a hematoma, unsightly scarring, and local sepsis which also requires much effort to avoid the recurrence of the condition. Hence rehabilitation exercises for DRA have been highly suggested to provide the stability to lower back and focus to reduce IRD [18]. Transverse abdominis activation exercises and pelvic floor muscle exercises are the most used exercises recommended for women’s health and are effective in the prevention and treatment of DRA [19]. The transverse abdominal muscle is the deepest abdominal muscle which has the deeper connections with the rectus abdominis muscle and linea alba. The activation of the transverse abdominis muscle brings the recti muscles together thereby improving the effective binding of the linea alba and fastening the recovery of DRA allowing women to resume back with their physical and social well-being of life [20].
Inertial measurement units are used for the diagnosis of a particular disease or trauma based on specific muscle movements [21]. The IMU sensor consists of an accelerometer for linear acceleration, a gyroscope for angular velocity, and a magnetometer; all three are in triaxial form to get measurements from all three axes [22,23]. IMU sensor is used for gait rehabilitation, the sensors are placed in lower limbs to track the muscle activity in elderly people. These IMU sensors are helpful in telerehabilitation. The IMU sensors are used for sports rehabilitation purposes too. In athletics, a wearable band with an IMU sensor helps in tracking the step count, impact load, and bone stimulus of the athlete [24]. IMU sensor is used along with Electromyography (EMG) sensors for rehabilitation of the exoskeleton in the arm and the IMU sensors are used for detecting forearm movement and EMG sensors for forearm strength detection [25]. Many smart wearable devices are developed which incorporate both IMU sensor and EMG sensor for rehabilitation in which the IMU sensors are used for tracking the motion and surface electromyography (sEMG) are used for detecting the strength of the muscle [26]. IMU sensors in a wearable device for pregnant women to monitor the position of fetal movement and to measure its position, duration, and relative force of fetal movement [27]. Many studies related to IMU sensors discuss the development of wireless wearable devices with one or many IMU sensors for tracking the motion of the affected or targeted parts of the body [26,28].
In this study, IMU sensors are used to evaluate the correctness of the exercises during rehabilitation therapy. IMU sensor signals are obtained from DRA patients when they are performing exercises. Signals are acquired for seven exercises for both correct and incorrect methods of carrying out the exercises. Linear acceleration, Gyroscope, and magnetometer outputs of IMU sensor are taken into consideration. Although many researchers worked on various bio signals like Electroencephalography (EEG) and EMG [29] to study and classify them for their abnormality using essential features [30,31], however, no previous studies were focused on different exercise analyses, especially for DRA patients. The exercises performed by the patients have to be assessed individually to study the meaningful insight of the activity that requires to function effectively. Each exercise considered for rehabilitation of DRA works in a unique way to strengthen the core abdominal muscles and also to reduce IRD. Hence with the acquisition of IMU signals for every exercise, the essential features are identified and thereby finding the effectiveness of exercises during rehabilitation.
Machine learning is an application of Artificial Intelligence to solve a wide range of problems in a supervised learning approach. In particular, the classification and optimization problems are well handled with machine learning algorithms. Perceptrons, k- Nearest Neighbor (kNN), Support Vector Machines (SVM), and Multilayer Perceptrons (MLP) are the classifiers used for the classification of different kinds of data in enormous applications. Convolutional Neural Networks and MLP [32,33] use the feedforward neural network principle for classification. The performance can be improved using optimization algorithms in terms of convergence rate, sensitivity, and accuracy [33–35].
The remaining portions of the manuscript are arranged as follows. A summary of the suggested methodology can be found in Section 3. Section 4, specifics the datasets used for experiments carried out, and their analyses were addressed. The summary of this research project and the area for future effort are presented in Section 5.
The analysis of exercises for DRA is minimally researched in literature and the results contribute towards the assistance to self-paced patients and physicians. The correct and incorrect exercises can be classified efficiently to assist the patients with DRA. The significant features are extracted from the IMU signals and using feature selection algorithms the best features are selected and classified using various machine learning classification models. Hence the algorithm with the best accuracy is used for the classification of correct and incorrect exercises by the ensembled learning method. The assessment of various exercises for DRA patients places a vital need to indicate to the patients about any incorrectness and perform the exercises effectively. The workflow of the proposed model is given in Fig. 1.
Figure 1: Workflow of the proposed methodology
The rectus abdominis muscles are the paired strap of muscles located in the abdominal region. They are separated by a thin fibrous sheath called Linea Alba. IRD is the distance of the Linea Alba between the connective tissue surrounding the rectus abdominis muscles. From Fig. 2, it is evident that the Linea Alba stretches during pregnancy and the width is very small in normally functioning abdomen muscle. These recti muscles would return to their natural position and the IRD decreases after delivering the infant. During pregnancy, the expansion of the uterus causes strains over the recti abdominis muscles and leads to the detachment of Linea Alba. This causes a surge in inter-recti-distance which is an indication of Diastasis Recti Abdominis (DRA) [36]. A substantial number of women in their postpartum are experiencing DRA. Women with DRA experience lower back pain, difficulty in lifting objects, pelvic pain, etc. In some extreme cases, they get hernia as a complication [37]. The separation between the recti abdominis muscles of less than 3 cm is labeled as mild diastasis. When it is in the range of 3 to 5 cm, patients are with moderate diastasis. When the separation is larger than 5 cm, the patients are classified as having severe diastasis [38]. The DRA is usually diagnosed through physical examination or abdominal ultrasonography. In most cases, conservative treatment physiotherapy is used to treat DRA patients. The abdominal exercises are used to treat patients to reduce the width of the recti abdominis muscles [39]. For some extreme diastasis cases of abdominoplasty, surgery on the abdomen is carried out to correct the recti abdominis muscle [40].
Figure 2: Anatomy of normal abdominal muscles and with DRA [41]
3.3 Role of Therapeutic Exercises
Abdominal exercises are the first stage of DRA treatment recommended by doctors or physiotherapists. This study entails a few exercises prescribed that aid in the rehabilitation of the abdominal muscles and the reconnection of the Linea Alba. These core strengthening exercises are only effective if performed properly, thus providing a chance for recovery. Performing incorrect exercises can cause an increase in Inter-recti Distance (IRD), resulting in further complications. The exercises performed during the study were Sit-Ups (SU), Curl-Ups (CU) Transverse Abdominis with Sit-Ups (TASU), Transverse Abdominis with Curl-Ups (TACU), Transverse Abdominis Pelvic Floor Muscles Curl Up (TAPFM-CU), Straight Leg Exercise (SLR) and Reverse Curl Up (RCU). The rectus abdominis muscle is contracted in both Sit-Ups and Curl-Ups [42]. Sit-Ups should be performed when the muscles are in the TA-contracted state. Curl-ups must be performed in addition to Transverse Abdominis (TA) and Pelvic floor Muscle (PFM) contractions. The lower abdominal muscles primarily benefit from straight leg raises [43]. The inner unit muscles provide stabilization to the core. The insistence must be given to achieve the controlled isolation of each muscle in the unit when performing inner unit exercises. Once the isolation in the inner unit is achieved, exercises should be progressed to include the outer unit as well. It is recommended that during the prenatal and early prenatal periods, a diaphragmatic breathing pattern should be adopted.
3.4 Experimental Set-Up and IMU Signal Acquisition
The datasets are collected from twenty subjects with each subject performing seven exercises both correctly and incorrectly. IMU signals tend to have small shakes and distortions and they are removed using rectification and filtering. The sensors are calibrated initially to reduce the unstable nature of IMU signals. In Fig. 3 the IMU sensor unit is placed on the abdominal region of the subject. The subjects perform each exercise three times in regular intervals. The Shimmer3 IMU sensor is used to acquire IMU sensor signals for this study. The sensor output includes the values of low noise acceleration, wide-range acceleration, angular velocity, and magnetic flux. In Shimmer, the sampling frequency can be varied up to 512 Hz and for IMU data acquisition it is set at 50 Hz as the values of IMU components fall within the range. The analysis of the spectrum of the signals reveals sampling rate of 20–50 Hz is ideal for human activity recognition in IMU for its better performance [44]. Table 1 describes the hardware specifications of the accelerometer, gyroscope, and magnetometer in the Shimmer3 IMU sensor unit. The IMU signals acquired are processed in the Python platform with Sklearn and Pandas library. These libraries are essential tools for data processing and machine learning-based classification problems. The feature ranking part of programming is done in MATLAB.
Figure 3: IMU sensor unit
The workflow of the preprocessing is presented in Fig. 4.
Figure 4: Block diagram of the preprocessing steps
Rectifying inclinations
During the collection of data, the sensor is rigidly attached to the body. As the body is in a state of constant motion, inaccuracy in the sensed data can be observed as the sensor attached to the body is prone to movements. To reduce the sensor inclination, an average value for a channel is obtained, Then, each data value is subtracted from the average value while standing still.
Filtering the signal
To eliminate the noise, a low-pass filter is applied. This filter was implemented to minimize the noise:
where y is the filtered output of the input
A set of features in different domains such as time domain, frequency domain, time-frequency domain, and some non-linear features are extracted from the data.
Statistical features are determined through the statistical analysis of the data. Root mean square (RMS), variance and kurtosis are the statistical features used for extracting features.
Root Mean Square (RMS)
Root mean square is a measure that shows the average difference between the predicted values and values that are observed. It is a risk function related to the expected value of the squared error loss or quadratic loss. It can tell how far off we should expect our model to be in its next prediction.
where
Variance
It shows the changes in the model when using different portions of the training datasets. It refers to how much the ML function can adjust depending on the given data set and shows how far the data is laid out. Models with high variance will have low bias and vice versa.
where
Kurtosis
It measures the relative peak of a distribution and shows the total degree of outliers present. It compares the extreme values with the other tail. It is simply a measure of outliers present in the distribution.
where
3.6.2 Frequency Domain Features
In the frequency domain, the signal’s features are extracted by analyzing the signals in the frequency domain. Power Spectral Density (PSD), mean frequency, and median frequency features are features applied to the data.
Power Spectral Density (PSD)
Power spectral density is the measure of the signal’s power content versus frequency. PSD gives an analysis of power distribution over a range of frequencies. It shows the strength of the variations (energy) as a function of frequency The amplitude of the PSD is normalized by the spectral resolution employed to digitize the signal.
where
PSD helps to identify the sections of frequencies that are strong and weak. It is also used to characterize broadband random signals.
Mean Frequency
The mean frequency of the observations is the sum of all the observed frequencies divided by the total number of observations. The power spectrum of the signal in the time domain is obtained and then the mean normalized frequency of that spectrum is calculated.
where
Median Frequency
The median frequency represents the midpoint of the power distribution and is the frequency below and above which lies 50% of the total power. This is a frequency domain-based feature where the PSD of the signal is initially calculated. Then the median normalized frequency is calculated.
where
Non-linear features are useful in characterizing the vibrational nature of the signal. Detrended fluctuation analysis (DFA), Lyapunov’s exponent, and entropy are the non-linear features used for the feature extraction.
Detrended Fluctuation Analysis (DFA)
It is used to characterize long-range power-law correlations in complex signals. It describes the degree of self-affinity in a signal. Time series can be split into different windows using Hurst’s Rescaled Range (R/S) and the range R can be inspected by rescaling the standard deviation [45].
It measures the scaling behavior of the signal fluctuations; the scaling exponent is a generalization of the Hurst exponent. The slope of the (R/S) component vs. the time period (T) hives the Hurst exponent.
Lyapunov’s Exponent
It gives the rate of exponential divergence from perturbed initial conditions. One sequence of computations will yield an estimate of both the level of chaos and the system complexity. It describes the degree of convergence or divergence of a signal. Convergence is indicated by the negative values and divergence is indicated by positive values.
where d is the distance between each phase-space point and its nearest neighbor.
The signal points maintain similar phase-space points throughout the signal duration for values closer to zero.
Entropy
Entropy describes the regularity of the signal. When the signal is highly complex, the value of this entropy is high. Low approximate entropy is observed for a regular and predictable time-series signal. It can measure the uncertainty of a random process thus it is useful in classification [46].
where N is the length of a data sequence, and
For modeling the classifier, the top features which are obtained from feature ranking are considered. So, the classifier could learn only the necessary features and the remaining features can be ignored. Since the remaining features do not have any influence on the final response of the classifier. Fischer score and Minimum Redundancy and Maximum Relevance (MRMR) score algorithms are used to rank the features. The top common features from the two algorithms are taken and given as input to the classifier for the prediction.
The top-ranked common features from both the feature ranking algorithms are given as input to the classifier. The classifier takes these features and gets trained. The final output would be binary, whether the exercise is done correctly or not. For classification, a dataset is made by combining the features of each column in the actual data. This is given for feature ranking and the top features columns are separately made as a dataset along with the label. This dataset is used for the classification. The data is split into training and testing datasets, the training data is used to train the classifier model. The train-test split for this study is 80%–20%.
Ensemble learning is used for this study which combines the multiple decision tree classifiers and makes the model predictive. Bagging and boosting are the types of ensemble techniques employed in this work. In bagging, multiple subsets of data are created and the model learns from multiple subsets of data by taking the overall average of each subset’s accuracy. The final prediction is made by combining all these models. Because of generating multiple subsets of the dataset prediction variance is reduced in bagging. In boosting, first, a prediction model is developed and then the next model is developed that tries to reduce the errors that occur in the first model. This progress continues until all the values are correctly predicted or until the number of models is specified for model generation. In this study, boosting algorithms such as AdaBoost, XG Boost, and Gradient Boost are used.
The IMU sensor data is acquired through the Shimmer3 device. The patients are initially asked to lie on a flat surface and they were given a small rundown about all the exercises. The device is strapped over the abdomen region of the patient and the data is acquired when the exercises are performed. The signals acquired from the IMU sensors are the outputs of the accelerometer, gyroscope, and magnetometer in the IMU sensor along the triaxial directions X, Y, and Z. The data is acquired for both correctly and incorrectly performed exercises. The sample size of the IMU dataset is between 1000–1500 data points for all the exercises which have been recorded as 12-column data of accelerometer (includes low noise and wide range), gyroscope, and magnetometer along directions X, Y, and Z. The statistical, non-linear, and frequency domain features are calculated for 9 parameters altogether and the top 10 ranked features are obtained through a feature selection algorithm and used for classification.
4.2 Analysis of Feature Selection
This section discusses the feature extraction and feature selection methods used in the proposed model. For a single column of data (i.e. acceleration along the X-axis) all the features mentioned in Fig. 5 are extracted for all the exercises. Similarly, features are applied to all the columns of data. Then the feature selection is done using the MRMR score and Fischer score. The features selected from both the feature ranking methods are merged and the top 10 features are used for classification. The observations related to feature ranking algorithms are presented in Fig. 6.
Figure 5: Workflow of feature extraction and feature selection
Figure 6: The Feature ranking score-(a) MRMR score (b) Fischer score
There are few observations based on the feature selection by ranking methods that can be inferred. The non-linear features show more differences between correctly and incorrectly done exercises than the other extracted features as they carry the most feature importance score. The importance score is greater for the motion along the X-axis. The wide-range noise accelerometer has performed well with the dataset and has more feature importance scores. So, among all other sensors, it plays a major role.
4.3 Analysis of Machine Learning Models
The classification models are applied to the dataset which consists of ten features and one categorical output variable to predict whether the exercise is done correctly or incorrectly. The observations of the feature ranking phase are presented in Fig. 6. In Table 2, the performance metrics are used to compare the efficiency of these models. The Adaboost algorithm has the highest accuracy of 92% as stated in Fig. 7, followed by boosting classifiers and then bagging classifiers. It is observed that precision, recall, and F1 score are high which is considered to be so important.
Figure 7: Analysis of classification accuracy
IMU sensors are in general used for gait analysis, fall detection, body posture analysis, and inertial motion measurements. In this research, we have proposed a model based on IMU sensors for the analysis of rehabilitation exercises of DRA. The key objective of this research is to analyze the correctness of abdominal exercises in the rehabilitation of patients facing DRA. To the best of our knowledge, this is the first attempt to present an objectified assessment of abdominal exercises of DRA using IMU sensors. The designed machine learning model is used for classifying the exercises performed as correct or incorrect. The non-linear features in addition to the frequency-domain features and time-frequency domain features were effective in classifying the IMU signals. This research was conducted with a minimum sample size based on the prevalence. Hence as future work, data can be collected from subjects of different geographical location and the inclusion of multiple sensors provide better assessment and grading of the correctness of exercises performed.
Funding Statement: This research was funded by the Department of Science and Technology DST under Biomedical Device and Technology Development (File No: TDP/BDTD/07/2021).
Conflicts of Interest: The authors declare that they have no conflicts of interest to report regarding the present study.
References
1. M. Cavalli, A. Aiolf, P. G. Bruni, L. Manfredini, F. Lombardo et al., “Prevalence and risk factors for diastasis recti abdominis: A review and proposal of a new anatomical variation,” Hernia, vol. 25, no. 4, pp. 883–890, 2021. [Google Scholar] [PubMed]
2. M. Eriksson Crommert, K. Petrov Fieril and C. Gustavsson, “Women’s experiences of living with increased inter-recti distance after childbirth: An interview study,” BMC Women’s Health, vol. 20, no. 1, pp. 260, 2020. [Google Scholar] [PubMed]
3. M. Radhakrishnan and K. Ramamurthy, “Efficacy and challenges in the treatment of diastasis recti abdominis—a scoping review on the current trends and future perspectives,” Diagnostics, vol. 12, no. 9, pp. 2044, 2022. [Google Scholar] [PubMed]
4. M. Radhakrishnan, K. Ramamurthy, A. Kothandaraman, V. J. Premkumar and N. Ramesh, “Automatic assessment of abdominal exercises for the treatment of diastasis recti abdominis using electromyography and machine learning,” Symmetry, vol. 14, no. 8, pp. 1654, 2022. [Google Scholar]
5. M. Radhakrishnan, K. Ramamurthy and V. J. Premkumar, EMG-based analysis of rehabilitation exercises for diastasis recti abdominis. In: Communication, Software and Networks. Singapore: Springer, pp. 61–70, 2023. [Google Scholar]
6. P. G. F. Da Mota, A. G. B. A. Pascoal, A. I. A. D. Carita and K. Bø, “Prevalence and risk factors of diastasis recti abdominis from late pregnancy to 6 months postpartum, and relationship with lumbo-pelvic pain,” Manual Therapy, vol. 20, no. 1, pp. 200–205, 2015. [Google Scholar]
7. P. Tian, D. M. Liu, C. Wang, Y. Gu, G. Du et al., “An ultrasound observation study on the levator hiatus with or without diastasis recti abdominis in postpartum women,” International Urogynecology Journal, vol. 32, no. 7, pp. 1839–1846, 2021. [Google Scholar] [PubMed]
8. C. M. Chiarello, L. A. Falzone, K. E. McCaslin, M. N. Patel and K. R. Ulery, “The effects of an exercise program on diastasis recti abdominis in pregnant women,” Journal of Women’s Health Physical Therapy, vol. 29, no. 1, pp. 11–16, 2005. [Google Scholar]
9. A. A. Thabet and M. A. Alshehri, “ Efficacy of deep core stability exercise program in postpartum women with diastasis recti abdominis: A randomised controlled trial,” Journal of Musculoskeletal & Neuronal Interactions, vol. 19, no. 1, pp. 62–68, 2019. [Google Scholar]
10. N. Acharry and R. K. Kutty, “Abdominal exercise with bracing, a therapeutic efficacy in reducing diastasis-recti among postpartal females,” International Journal of Physiotherapy and Research, vol. 3, no. 2, pp. 999–1005, 2015. [Google Scholar]
11. R. C. Tung and S. Towfigh, “Diagnostic techniques for diastasis recti,” Hernia, vol. 25, no. 4, pp. 915–919, 2021. [Google Scholar] [PubMed]
12. N. F. Hills, R. B. Graham and L. McLean, “Comparison of trunk muscle function between women with and without diastasis recti abdominis at 1 year postpartum,” Physical Therapy, vol. 98, no. 10, pp. 891–901, 2018. [Google Scholar] [PubMed]
13. S. M. McGill, A. Childs and C. Liebenson, “Endurance times for low back stabilization exercises: Clinical targets for testing and training from a normal database,” Archives of Physical Medicine and Rehabilitation, vol. 80, no. 8, pp. 941–944, 1999. [Google Scholar] [PubMed]
14. A. T. M. Van de Water and D. R. Benjamin, “Measurement methods to assess diastasis of the rectus abdominis muscle (DRAMA systematic review of their measurement properties and meta-analytic reliability generalisation,” Manual Therapy, vol. 21, no. 2, pp. 41–53, 2016. [Google Scholar] [PubMed]
15. P. Z. Bobowik and A. Dąbek, “Physiotherapy in women with diastasis of the rectus abdominis muscles,” Advances in Rehabilitation, vol. 32, no. 3, pp. 11–17, 2018. [Google Scholar]
16. P. Emanuelsson, “Alternatives in the treatment of abdominal rectus muscle diastasis. An evaluation,” Karolinska Institutet, 2014. [Google Scholar]
17. A. Olsson, O. Kiwanuka, G. Sandblom and O. Stackelberg, “Evaluation of functional outcomes following rectus diastasis repair—an up-to-date literature review,” Hernia, vol. 25, no. 4, pp. 905–914, 2021. [Google Scholar] [PubMed]
18. S. S. Al-azzawi, S. Khaksar, E. K. Hadi, H. Agrawal and I. Murray, “HeadUp: A low-cost solution for tracking head movement of children with cerebral palsy using IMU,” Sensors, vol. 21, no. 23, pp. 8148, 2021. [Google Scholar] [PubMed]
19. S. Gluppea, M. E. Engh and K. Bo, “What is the evidence for abdominal and pelvic floor muscle training to treat diastasis recti abdominis postpartum? A systematic review with meta-analysis,” Brazilian Journal of Physical Therapy, vol. 25, no. 6, pp. 664–675, 2021. [Google Scholar]
20. D. R. Benjamin, A. T. Van de Water and C. L. Peiris, “Effects of exercise on diastasis of the rectus abdominis muscle in the antenatal and postnatal periods: a systematic review,” Physiotherapy, vol. 100, no. 1, pp. 1–8, 2014. [Google Scholar] [PubMed]
21. G. Fenu and G. Steri, “IMU based post-traumatic rehabilitation assessment,” in 3rd Int. Symp. on Applied Sciences in Biomedical and Communication Technologies, Rome, Italy, pp. 1–5, 2010. [Google Scholar]
22. N. Ahmad, R. A. R. Ghazilla, N. M. Khairi and V. Kasi, “Reviews on various inertial measurement unit (imu) sensor applications,” International Journal of Signal Processing Systems, vol. 1, no. 2, pp. 256–262, 2013. [Google Scholar]
23. W. Kong, S. Sessa, S. Cosentino, M. Zecca, K. Saito et al., “Development of a real-time IMU-based motion capture system for gait rehabilitation,” in IEEE Int. Conf. on Robotics and Biomimetics, Shenzhen, China, pp. 2100–2105, 2013. [Google Scholar]
24. M. Armitage, M. Beato and S. A. McErlain-Naylor, “Inter-unit reliability of IMU step metrics using IMeasureU blue trident inertial measurement units for running-based team sport tasks,” Journal of Sports Sciences, vol. 39, no. 13, pp. 1512–1518, 2021. [Google Scholar] [PubMed]
25. Y. Ganesan, S. Gobee and V. Durairajah, “Development of an upper limb exoskeleton for rehabilitation with feedback from emg and imu sensor,” Procedia Computer Science, vol. 76, no. 15, pp. 53–59, 2015. [Google Scholar]
26. C. Cifuentes, A. Braidot, L. Rodríguez, M. Frisoli, A. Santiago et al., “Development of a wearable ZigBee sensor system for upper limb rehabilitation robotics,” in 4th IEEE RAS & EMBS Int. Conf. on Biomedical Robotics and Biomechatronics (BioRob), Rome, Italy, pp. 1989–1994, 2012. [Google Scholar]
27. Y. C. Du, L. B. Yen, P. L. Kuo and P. Y. Tsai, “A wearable device for evaluation of relative position, force, and duration of fetal movement for pregnant woman care,” IEEE Sensors Journal, vol. 21, no. 17, pp. 19341–19350, 2021. [Google Scholar]
28. W. Zhao, S. Yang and X. Luo, “Towards rehabilitation at home after total knee replacement,” Tsinghua Science and Technology, vol. 26, no. 6, pp. 791–799, 2021. [Google Scholar]
29. N. Pakniyat and H. Namazi, “Complexity-based analysis of the variations of brain and muscle reactions in walking and standing balance while receiving different perturbations,” Frontiers in Human Neuroscience, vol. 15, pp. 749082, 2021. [Google Scholar] [PubMed]
30. P. Carpena, M. Gómez-Extremera and P. A. Bernaola-Galván, “On the validity of detrended fluctuation analysis at short scales,” Entropy (Basel), vol. 24, no. 1, pp. 61, 2021. [Google Scholar] [PubMed]
31. A. Hekmatmanesh, H. Wu and H. Handroos, “largest lyapunov exponent optimization for control of a bionic-hand: A brain computer interface study,” Frontiers in Rehabilitation Sciences, vol. 2, pp. 802070, 2022. [Google Scholar] [PubMed]
32. M. Khishe and A. Safari, “Classification of sonar targets using an MLP neural network trained by dragonfly algorithm,” Wireless Personal Communications, vol. 108, no. 4, pp. 2241–2260, 2019. [Google Scholar]
33. M. Khishe and H. Mohammadi, “Passive sonar target classification using multi-layer perceptron trained by salp swarm algorithm,” Ocean Engineering, vol. 181, no. 2, pp. 98–108, 2019. [Google Scholar]
34. M. R. Mosavi, M. Khishe, M. J. Naseri, G. R. Parvizi and M. Ayat, “Multi-layer perceptron neural network utilizing adaptive best-mass gravitational search algorithm to classify sonar dataset,” Archives of Acoustics, vol. 44, pp. 137–151, 2019. [Google Scholar]
35. M. R. Mosavi and M. Khishe, “Training a feed-forward neural network using particle swarm optimizer with autonomous groups for sonar target classification,” Journal of Circuits, Systems and Computers, vol. 26, no. 11, pp. 1750185, 2017. [Google Scholar]
36. N. Keshwani, N. Hills and L. McLean, “Inter-rectus distance measurement using ultrasound imaging: Does the rater matter?,” Physiotherapy Canada, vol. 68, no. 3, pp. 223–229, 2015. [Google Scholar]
37. Q. Wang, X. Yu, G. Chen, X. Sun and J. Wang, “Does diastasis recti abdominis weaken pelvic floor function? A cross-sectional study,” International Urogynecology Journal, vol. 31, no. 2, pp. 277–283, 2020. [Google Scholar] [PubMed]
38. W. Reinpold, F. Köckerling, R. Bittner, J. Conze, R. Fortelny et al., “Classification of rectus diastasis-A proposal by the german hernia society (DHG) and the international endohernia society (IEHS),” Frontiers in Surgery, vol. 6, no. 1, pp. 1–6, 2019. [Google Scholar] [PubMed]
39. A. Michalska, W. Rokita, D. Wolder, J. Pogorzelska and K. Kaczmarczyk, “Diastasis recti abdominis— a review of treatment methods,” Ginekologia polska, vol. 89, no. 2, pp. 97–101, 2018. [Google Scholar] [PubMed]
40. J. Gormley, A. Copeland, H. Augustine, C. Axelrod and M. McRae, “Impact of rectus diastasis repair on abdominal strength and function: A systematic review,” Cureus, vol. 12, no. 12, pp. e12358, 2020. [Google Scholar] [PubMed]
41. Brad Shaw, “Postnatal diastasis recti do’s and don’ts,” Sustain Health, 2020. https://sustainhealth.fit/lifestyle/postnatal-diastasis-recti/ [Google Scholar]
42. A. Rutkowska-Kucharska and A. Szpala, “Electromyographic muscle activity in curl-up exercises with different positions of upper and lower extremities,” Journal of Strength and Conditioning Research, vol. 24, no. 11, pp. 3133–3139, 2010. [Google Scholar] [PubMed]
43. A. M. Youssef, A. A. Sabbour and R. M. Kamel, “Muscle activity in upper and lower portions of rectus abdominis during abdominal exercises in postnatal women having diastasis recti,” Bulletin of Faculty Pharmacy, Cairo University, vol. 8, no. 1, pp. 117–125, 2003. [Google Scholar]
44. J. A. Santoyo-Ramón, E. Casilari and J. M. Cano-García, “A study of the influence of the sensor sampling frequency on the performance of wearable fall detectors,” Measurement, vol. 193, no. 4, pp. 110945, 2022. [Google Scholar]
45. G. Gaurav, R. S. Anand and V. Kumar, “EEG based cognitive task classification using multifractal detrended fluctuation analysis,” Cognitive Neurodynamics, vol. 15, no. 6, pp. 999–1013, 2021. [Google Scholar] [PubMed]
46. A. Al-Ezzi, A. A. Al-Shargabi, F. Al-Shargie and A. T. Zahary, “Complexity analysis of EEG in patients with social anxiety disorder using fuzzy entropy and machine learning techniques,” IEEE Access, vol. 10, pp. 39926–39938, 2022. [Google Scholar]
Cite This Article
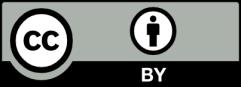
This work is licensed under a Creative Commons Attribution 4.0 International License , which permits unrestricted use, distribution, and reproduction in any medium, provided the original work is properly cited.