Open Access
ARTICLE
Network Learning-Enabled Sensor Association for Massive Internet of Things
1 Department of Information Systems, Faculty of Computing and Information Technology, King Abdulaziz University, Jeddah, 21589, Saudi Arabia
2 Department of Information and Communication Technologies, Universitat Pompeu Fabra, Barcelona, 08018, Spain
* Corresponding Author: Alaa Omran Almagrabi. Email:
(This article belongs to the Special Issue: Machine Learning for Industrial Internet of Things (IIoT))
Computer Systems Science and Engineering 2023, 47(1), 843-853. https://doi.org/10.32604/csse.2023.037652
Received 12 November 2022; Accepted 07 April 2023; Issue published 26 May 2023
Abstract
The massive Internet of Things (IoT) comprises different gateways (GW) covering a given region of a massive number of connected devices with sensors. In IoT networks, transmission interference is observed when different sensor devices (SD) try to send information to a single GW. This is mitigated by allotting various channels to adjoining GWs. Furthermore, SDs are permitted to associate with any GW in a network, naturally choosing the one with a higher received signal strength indicator (RSSI), regardless of whether it is the ideal choice for network execution. Finding an appropriate GW to optimize the performance of IoT systems is a difficult task given the complicated conditions among GWs and SDs. Recently, in remote IoT networks, the utilization of machine learning (ML) strategies has arisen as a viable answer to determine the effect of various models in the system, and reinforcement learning (RL) is one of these ML techniques. Therefore, this paper proposes the use of an RL algorithm for GW determination and association in IoT networks. For this purpose, this study allows GWs and SDs with intelligence, through executing the multi-armed bandit (MAB) calculation, to investigate and determine the optimal GW with which to associate. In this paper, rigorous mathematical calculations are performed for this purpose and evaluate our proposed mechanism over randomly generated situations, which include different IoT network topologies. The evaluation results indicate that our intelligent MAB-based mechanism enhances the association as compared to state-of-the-art (RSSI-based) and related research approaches.Keywords
Cite This Article
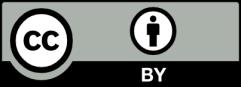
This work is licensed under a Creative Commons Attribution 4.0 International License , which permits unrestricted use, distribution, and reproduction in any medium, provided the original work is properly cited.