Open Access
ARTICLE
Ensemble Learning for Fetal Health Classification
1 Department of Computer Science, College of Computer Engineering and Sciences, Prince Sattam bin Abdulaziz University Al-Kharj, 16273, Saudi Arabia
2 Department of Computer Science, COMSATS University, Islamabad, 53000, Pakistan
3 Faculty of Computers & Information Technology, Computer Science Department, University of Tabuk, Tabuk, 71491, Saudi Arabia
4 Department of Computer Engineering and Networks, College of Computer and Information Sciences, Jouf University, Sakaka, 72388, Saudi Arabia
5 Information Systems Department, Faculty of Management Comenius University in Bratislava, Odbojárov 10, 82005 Bratislava 25, Slovakia
6 Department of Computer Science, Kinnaird College for Women, Lahore, 54000, Pakistan
* Corresponding Authors: Mesfer Al Duhayyim. Email: ; Natalia Kryvinska. Email:
Computer Systems Science and Engineering 2023, 47(1), 823-842. https://doi.org/10.32604/csse.2023.037488
Received 05 November 2022; Accepted 07 April 2023; Issue published 26 May 2023
Abstract
: Cardiotocography (CTG) represents the fetus’s health inside the womb during labor. However, assessment of its readings can be a highly subjective process depending on the expertise of the obstetrician. Digital signals from fetal monitors acquire parameters (i.e., fetal heart rate, contractions, acceleration). Objective:: This paper aims to classify the CTG readings containing imbalanced healthy, suspected, and pathological fetus readings. Method:: We perform two sets of experiments. Firstly, we employ five classifiers: Random Forest (RF), Adaptive Boosting (AdaBoost), Categorical Boosting (CatBoost), Extreme Gradient Boosting (XGBoost), and Light Gradient Boosting Machine (LGBM) without over-sampling to classify CTG readings into three categories: healthy, suspected, and pathological. Secondly, we employ an ensemble of the above-described classifiers with the over-sampling method. We use a random over-sampling technique to balance CTG records to train the ensemble models. We use 3602 CTG readings to train the ensemble classifiers and 1201 records to evaluate them. The outcomes of these classifiers are then fed into the soft voting classifier to obtain the most accurate results. Results:: Each classifier evaluates accuracy, Precision, Recall, F1-scores, and Area Under the Receiver Operating Curve (AUROC) values. Results reveal that the XGBoost, LGBM, and CatBoost classifiers yielded 99% accuracy. Conclusion:: Using ensemble classifiers over a balanced CTG dataset improves the detection accuracy compared to the previous studies and our first experiment. A soft voting classifier then eliminates the weakness of one individual classifier to yield superior performance of the overall model.Keywords
Cite This Article
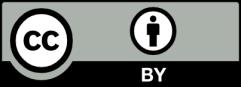
This work is licensed under a Creative Commons Attribution 4.0 International License , which permits unrestricted use, distribution, and reproduction in any medium, provided the original work is properly cited.