Open Access
ARTICLE
Chicken Swarm Optimization with Deep Learning Based Packaged Rooftop Units Fault Diagnosis Model
1 Department of Electronics and Instrumentation Engineering, B. S. Abdur Rahman Crescent Institute of Science and Technology, Chennai, 600048, India
2 Department of Computer Science and Engineering, University Institute of Engineering and Technology (UIET), Guru Nanak University, Hyderabad, India
3 Department of Electrical Engineering, College of Engineering, Jouf University, Saudi Arabia
4 Department of Computer Science and Engineering, Vignan’s Institute of Information Technology, Visakhapatnam, 530049, India
5 Department of Computer Science and Engineering, Sejong University, Seoul, 05006, Korea
6 Seculayer Company, Ltd., Seoul, 04784, Korea
* Corresponding Author: Jinsang You. Email:
Computer Systems Science and Engineering 2023, 47(1), 221-238. https://doi.org/10.32604/csse.2023.036479
Received 01 October 2022; Accepted 17 February 2023; Issue published 26 May 2023
Abstract
Rooftop units (RTUs) were commonly employed in small commercial buildings that represent that can frequently do not take the higher level maintenance that chillers receive. Fault detection and diagnosis (FDD) tools can be employed for RTU methods to ensure essential faults are addressed promptly. In this aspect, this article presents an Optimal Deep Belief Network based Fault Detection and Classification on Packaged Rooftop Units (ODBNFDC-PRTU) model. The ODBNFDC-PRTU technique considers fault diagnosis as a multi-class classification problem and is handled using DL models. For fault diagnosis in RTUs, the ODBNFDC-PRTU model exploits the deep belief network (DBN) classification model, which identifies seven distinct types of faults. At the same time, the chicken swarm optimization (CSO) algorithm-based hyperparameter tuning technique is utilized for resolving the trial and error hyperparameter selection process, showing the novelty of the work. To illustrate the enhanced performance of the ODBNFDC-PRTU algorithm, a comprehensive set of simulations are applied. The comparison study described the improvement of the ODBNFDC-PRTU method over other recent FDD algorithms with maximum accuracy of 99.30% and TPR of 93.09%.Keywords
Cite This Article
G. Anitha, N. Supriya, F. Alenezi, E. Laxmi Lydia, G. P. Joshi et al., "Chicken swarm optimization with deep learning based packaged rooftop units fault diagnosis model," Computer Systems Science and Engineering, vol. 47, no.1, pp. 221–238, 2023. https://doi.org/10.32604/csse.2023.036479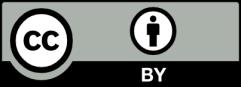